Preliminary Results on Sensitive Data Leakage in Federated Human Activity Recognition
2022 IEEE INTERNATIONAL CONFERENCE ON PERVASIVE COMPUTING AND COMMUNICATIONS WORKSHOPS AND OTHER AFFILIATED EVENTS (PERCOM WORKSHOPS)(2022)
摘要
Sensor-based Human Activity Recognition (HAR) has been a hot topic in pervasive computing for many years, as an enabling technology for several context-aware applications. However, the deployment of HAR in real-world scenarios is limited by some major challenges. Among those issues, privacy is particularly relevant, since activity patterns may reveal sensitive information about the users (e.g., personal habits, medical conditions). HAR solutions based on Federated Learning (FL) have been recently proposed to mitigate this problem. In FL, each user shares with a cloud server only the parameters of a locally trained model, while personal data are kept private. The cloud server is in charge of building a global model by aggregating the received parameters. Even though FL avoids the release of labelled sensor data, researchers have found that the parameters of deep learning models may still reveal sensitive information through specifically designed attacks. In this paper, we propose a first contribution in this line of research by introducing a novel framework to quantitatively evaluate the effectiveness of the Membership Inference Attack (MIA) for FL-based HAR. Our preliminary results on a public HAR dataset show how the global activity model may actually reveal sensitive information about the participating users and provide hints for future work on countering such attacks.
更多查看译文
关键词
human activity recognition, federated learning, privacy
AI 理解论文
溯源树
样例
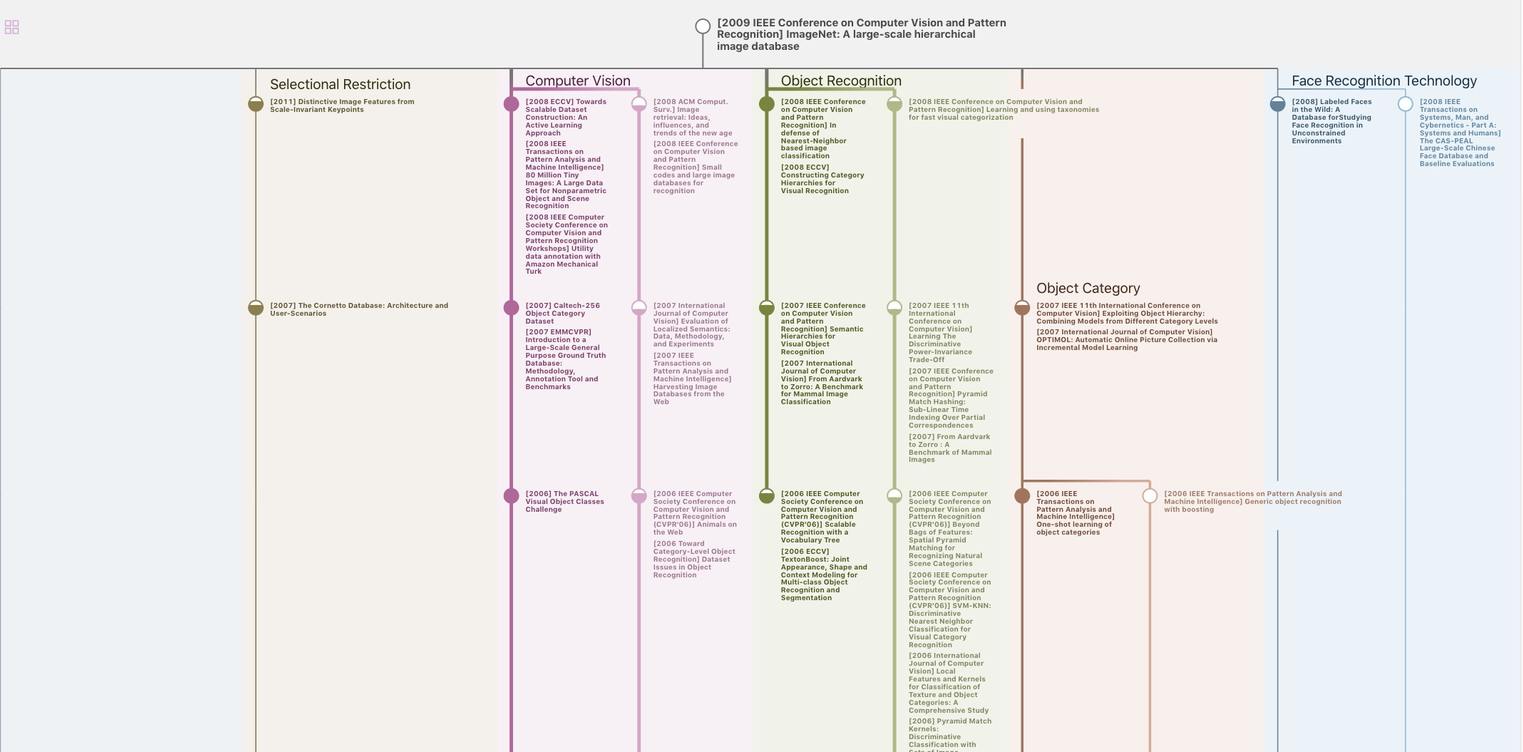
生成溯源树,研究论文发展脉络
Chat Paper
正在生成论文摘要