Reliability Analysis of a Spiking Neural Network Hardware Accelerator
2022 Design, Automation & Test in Europe Conference & Exhibition (DATE)(2022)
摘要
Despite the parallelism and sparsity in neural network models, their transfer into hardware unavoidably makes them susceptible to hardware-level faults. Hardware-level faults can occur either during manufacturing, such as physical defects and process-induced variations, or in the field due to environmental factors and aging. The performance under fault scenarios needs to be assessed so as to develop cost-effective fault-tolerance schemes. In this work, we assess the resilience characteristics of a hardware accelerator for Spiking Neural Networks (SNNs) designed in VHDL and implemented on an FPGA. The fault injection experiments pinpoint the parts of the design that need to be protected against faults, as well as the parts that are inherently fault-tolerant.
更多查看译文
关键词
fault injection experiments,inherently fault-tolerant,reliability analysis,neural network models,hardware-level faults,process-induced variations,fault scenarios,cost-effective fault-tolerance schemes,spiking neural network hardware accelerator,environmental factors,resilience characteristics,VHDL,FPGA
AI 理解论文
溯源树
样例
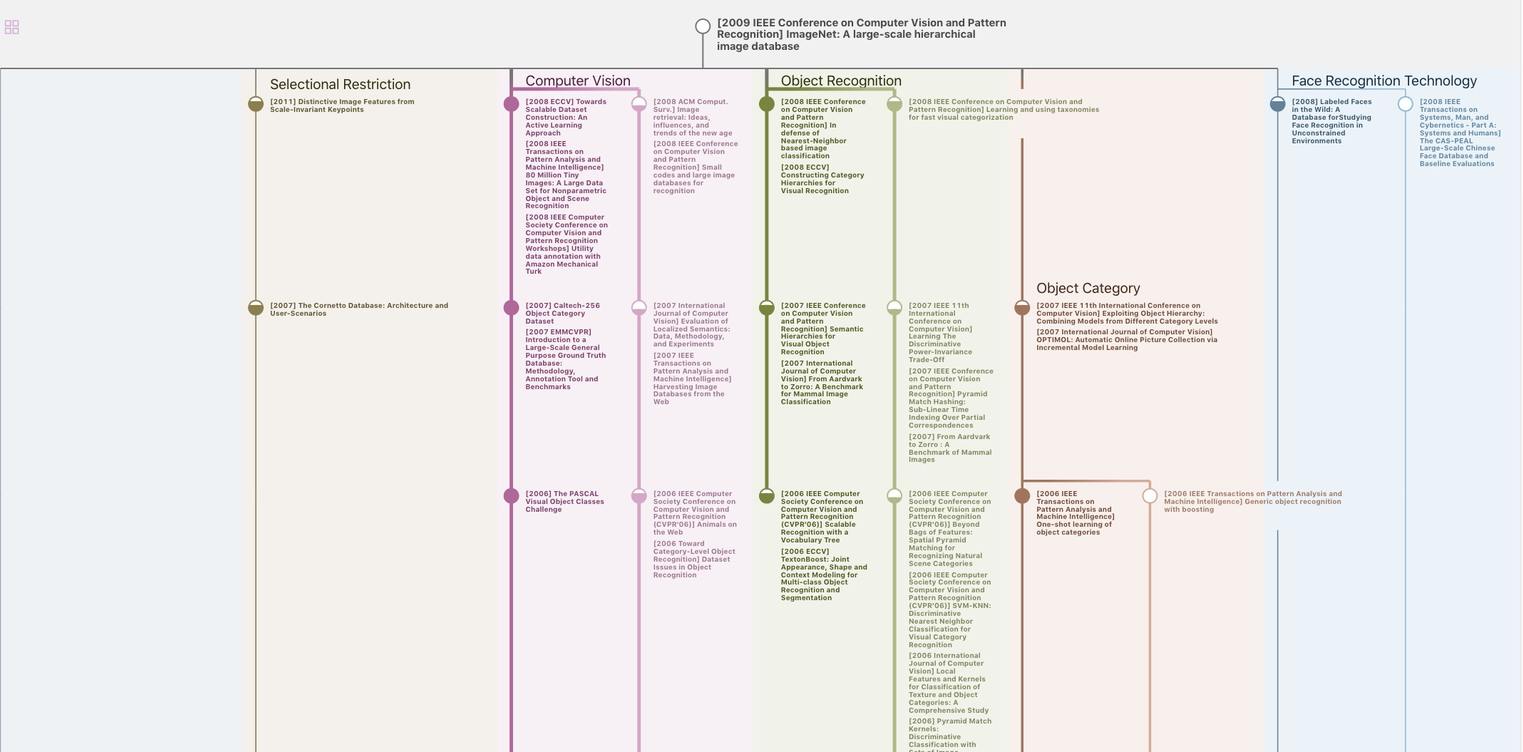
生成溯源树,研究论文发展脉络
Chat Paper
正在生成论文摘要