MEDEA: A Multi-objective Evolutionary Approach to DNN Hardware Mapping
2022 Design, Automation & Test in Europe Conference & Exhibition (DATE)(2022)
摘要
Deep Neural Networks (DNNs) embedded domain-specific accelerators enable inference on resource-constrained devices. Making optimal design choices and efficiently scheduling neural network algorithms on these specialized architectures is challenging. Many choices can be made to schedule computation spatially and temporally on the accelerator. Each choice influences the access pattern to the buffers of the architectural hierarchy, affecting the energy and latency of the inference. Each mapping also requires specific buffer capacities and a number of spatial components instances that translate in different chip area occupation. The space of possible combinations, the mapping space, is so large that automatic tools are needed for its rapid ex-ploration and simulation. This work presents MEDEA, an open-source multi-objective evolutionary algorithm based approach to DNNs accelerator mapping space exploration. MEDEA leverages the Timeloop analytical cost model. Differently from the other schedulers that optimize towards a single objective, MEDEA allows deriving the Pareto set of mappings to optimize towards multiple, sometimes conflicting, objectives simultaneously. We found that solutions found by MEDEA dominates in most cases those found by state-of-the-art mappers.
更多查看译文
关键词
neural network,accelerator,compiler,scheduling,genetic algorithm,multi-objective optimization
AI 理解论文
溯源树
样例
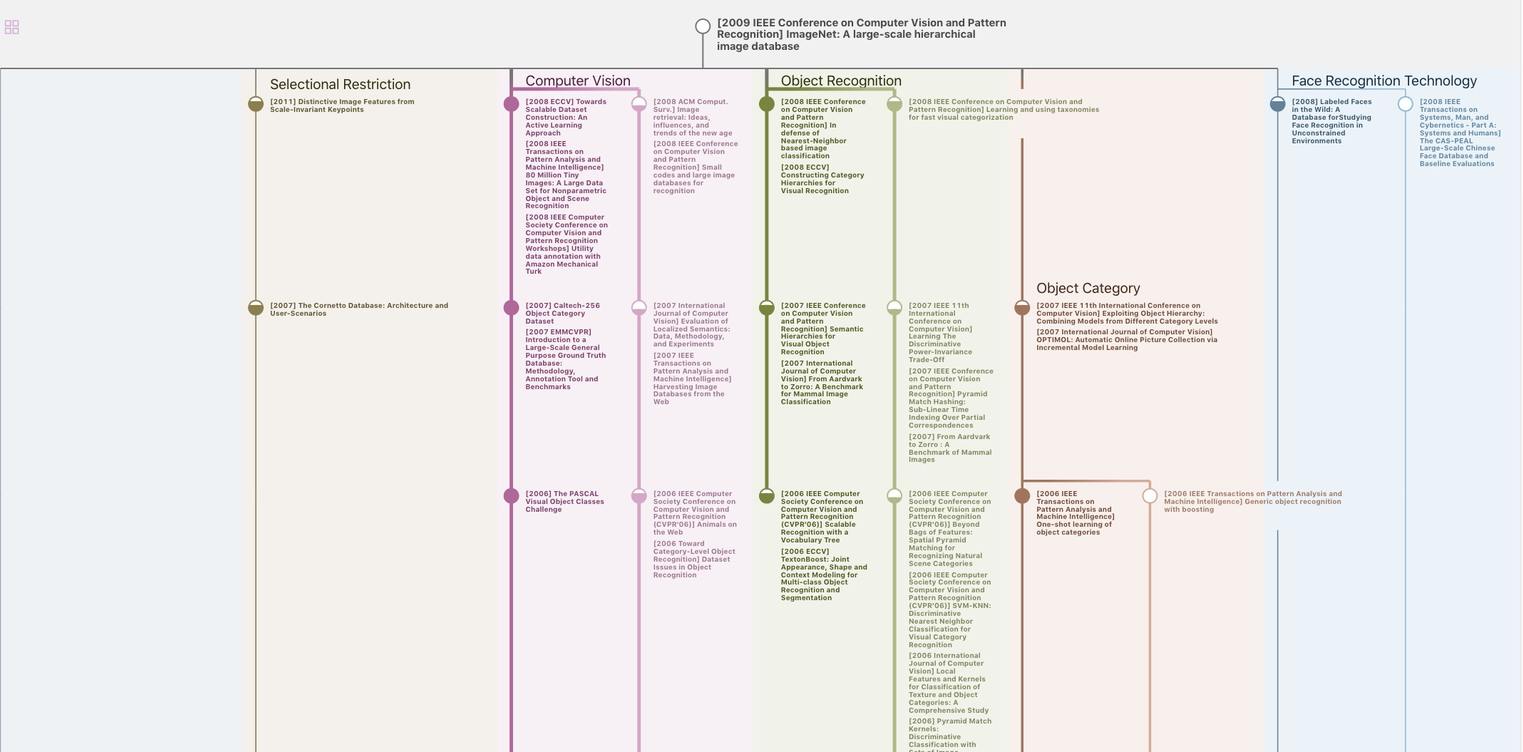
生成溯源树,研究论文发展脉络
Chat Paper
正在生成论文摘要