Refining Graph Structure for Incomplete Multi-View Clustering
IEEE TRANSACTIONS ON NEURAL NETWORKS AND LEARNING SYSTEMS(2024)
摘要
As a challenging problem, incomplete multi-view clustering (MVC) has drawn much attention in recent years. Most of the existing methods contain the feature recovering step inevitably to obtain the clustering result of incomplete multi-view datasets. The extra target of recovering the missing feature in the original data space or common subspace is difficult for unsupervised clustering tasks and could accumulate mistakes during the optimization. Moreover, the biased error is not taken into consideration in the previous graph-based methods. The biased error represents the unexpected change of incomplete graph structure, such as the increase in the intra-class relation density and the missing local graph structure of boundary instances. It would mislead those graph-based methods and degrade their final performance. In order to overcome these drawbacks, we propose a new graph-based method named Graph Structure Refining for Incomplete MVC (GSRIMC). GSRIMC avoids recovering feature steps and just fully explores the existing subgraphs of each view to produce superior clustering results. To handle the biased error, the biased error separation is the core step of GSRIMC. In detail, GSRIMC first extracts basic information from the precomputed subgraph of each view and then separates refined graph structure from biased error with the help of tensor nuclear norm. Besides, cross-view graph learning is proposed to capture the missing local graph structure and complete the refined graph structure based on the complementary principle. Extensive experiments show that our method achieves better performance than other state-of-the-art baselines.
更多查看译文
关键词
Tensors,Task analysis,Kernel,Optimization,Scientific computing,Learning systems,Laplace equations,Biased error,graph recovery,incomplete multi-view clustering (MVC),tensor nuclear norm (TNN)
AI 理解论文
溯源树
样例
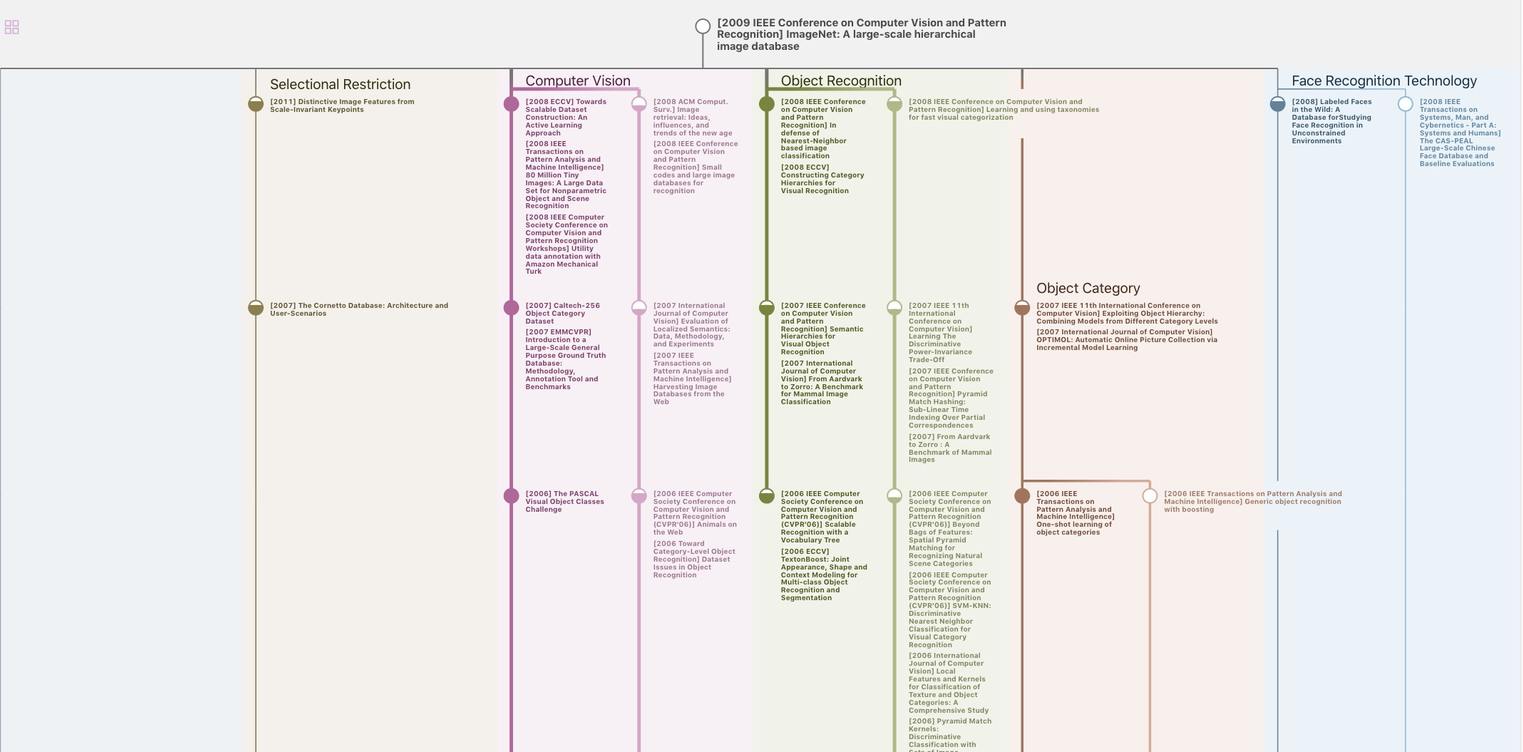
生成溯源树,研究论文发展脉络
Chat Paper
正在生成论文摘要