Snapshot-to-video autoencoder for compressed ultrahigh-speed imaging
AI AND OPTICAL DATA SCIENCES III(2022)
摘要
Single-shot two-dimensional (2D) optical imaging of transient scenes is indispensable for numerous areas of study. Among existing techniques, compressed optical-streaking ultrahigh-speed photography (COSUP) uses a cost-efficient design to endow ultra-high frame rates with off-the-shelf CCD and CMOS cameras. Thus far, COSUP's application scope is limited by the long processing time and unstable image quality in existing analytical-modeling-based video reconstruction. To overcome these problems, we have developed a snapshot-to-video autoencoder (S2V-AE)-a new deep neural network that maps a compressively recorded 2D image to a movie. The S2V-AE preserves spatiotemporal coherence in reconstructed videos and presents a flexible structure to tolerate changes in input data. Implemented in compressed ultrahigh-speed imaging, the S2V-AE enables the development of single-shot machine-learning assisted real-time (SMART) COSUP, which features a reconstruction time of 60 ms and a large sequence depth of 100 frames. SMART-COSUP is applied to wide-field multiple-particle tracking at 20 thousand frames-per-second. As a universal computational framework, the S2V-AE is readily adaptable to other modalities in high-dimensional compressed sensing. SMART-COSUP is also expected to find wide applications in applied and fundamental sciences.
更多查看译文
关键词
compressed sensing, deep learning, optical-streaking, ultrahigh-speed, autoencoder, generative adversarial network
AI 理解论文
溯源树
样例
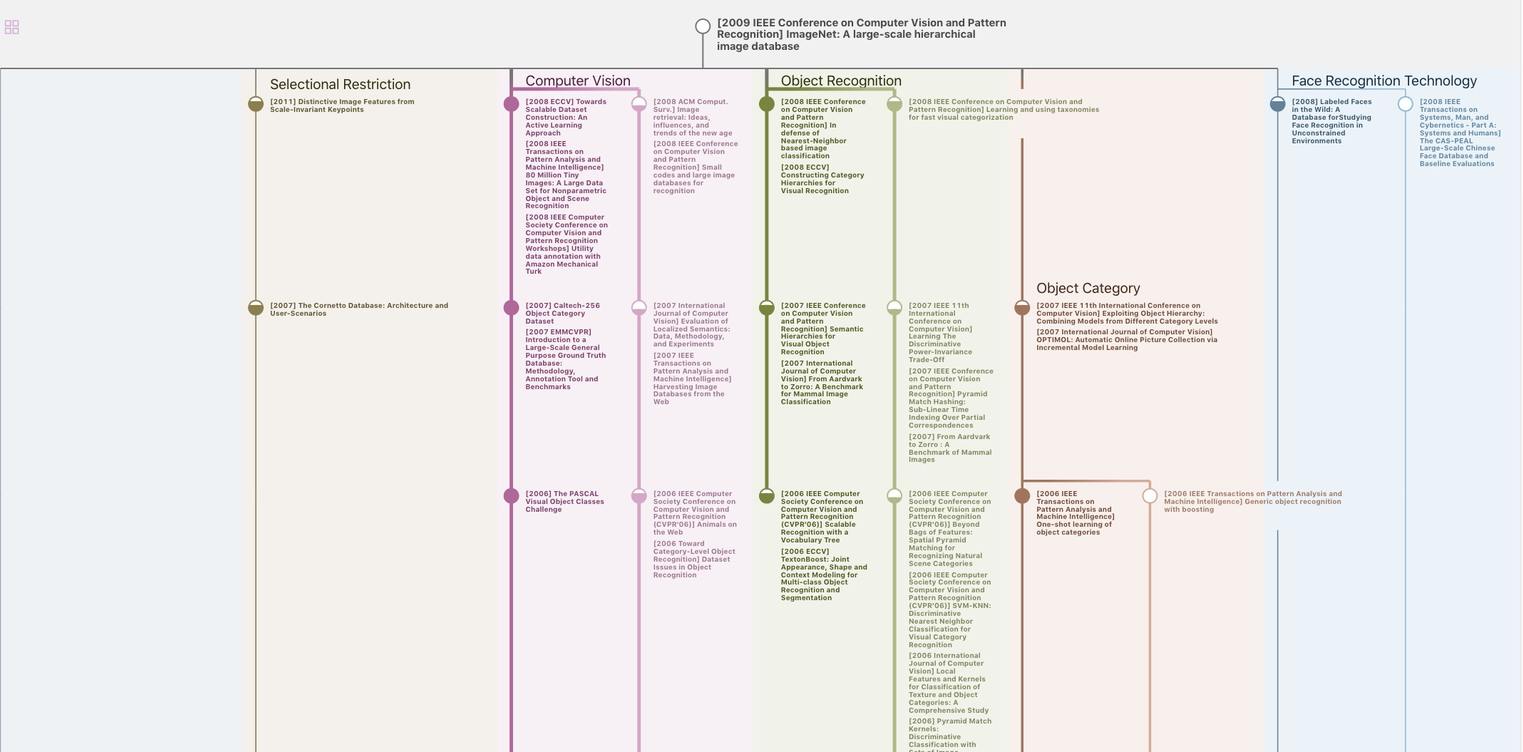
生成溯源树,研究论文发展脉络
Chat Paper
正在生成论文摘要