An Efficient and Decentralized Fuzzy Reinforcement Learning Bandwidth Controller for Multitenant Data Centers
Journal of Network and Systems Management(2022)
摘要
Cloud service providers rely on bandwidth overprovisioning to avoid Service Level Agreements’ violation (SLAs) when allocating tenants’ resources in multitenant cloud environments. Tenants’ network usage is usually dynamic, but the shared resources are often allocated statically and in batches, causing resource idleness. This paper envisions an opportunity for optimizing cloud service networks. As such, we propose an autonomous bandwidth allocation mechanism based on Fuzzy Reinforcement Learning (FRL) to reduce the idleness of cloud network resources. Our mechanism dynamically allocates resources, prioritizing tenants and allowing them to exceed the contracted bandwidth temporarily without violating the SLAs. We assess our mechanism by comparing FRL usage against pure Fuzzy Inference System (FIS) and pure Reinforcement Learning (RL). The evaluation scenario is an emulation in which tenants share resources from a cloud provider and generate traffic based on real HTTP traffic. The results show that our mechanism increases tenant’s cloud network utilization by 30% compared to FIS while maintaining the cloud traffic load within a healthy threshold and more stable than RL.
更多查看译文
关键词
Reinforcement learning,Fuzzy inference system,Bandwidth provisioning,Multitenant data center,Software-defined networking
AI 理解论文
溯源树
样例
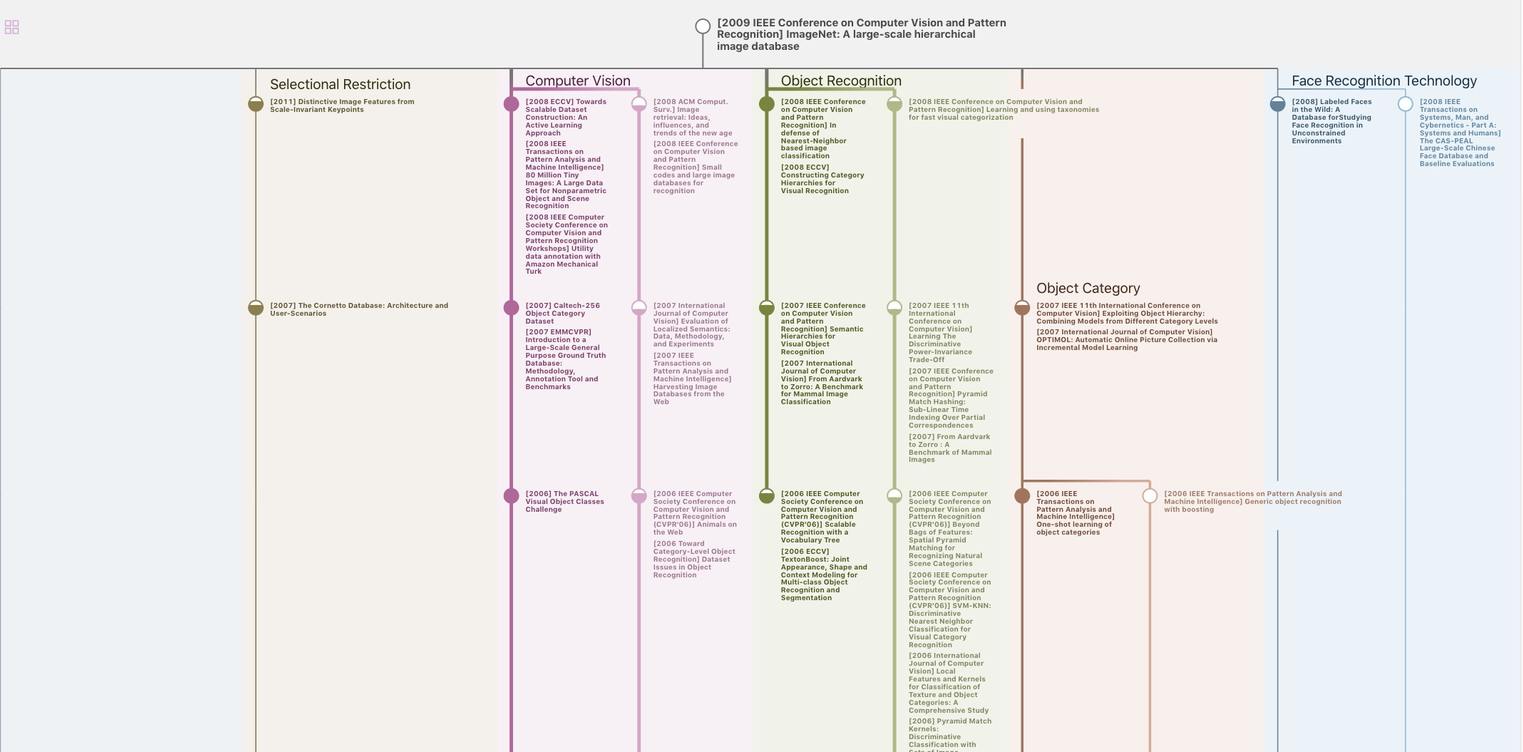
生成溯源树,研究论文发展脉络
Chat Paper
正在生成论文摘要