Intrusion detection for high-speed railways based on unsupervised anomaly detection models
Applied Intelligence(2022)
摘要
Intrusion detection for the high-speed railway is important for operational safety. Current detection methods based on deep learning use supervised learning paradigm, which heavily rely on large labeled datasets. However, real intrusion samples for railways are very rare and it is not possible to collect all types of intrusion objects. In this paper, we propose a new unsupervised intrusion detection method based on deep generative networks and auto-regression models. The deep generative network is train to reconstruct the input image, by learning the features, represented by the latent variables, from the input image. The auto-regression model is used to learn the probability distribution of the latent variables. The network is trained using just the normal samples to learn the features of normal samples. Therefore, the model can not reconstruct the abnormal images and the latent variable of abnormal image will have small likelihood probability according to the learned distribution. The anomaly is detected by a two-step mechanism, first the latent likelihood of the input image is computed, the second step is only performed when the likelihood is under a threshold. In the second step the model reconstructs the input image and the anomaly region is located by the difference of the reconstruction and the original input image. The proposed method is evaluated using a dataset built from realistic high-speed railway scenes. The results show that our method is able to detect the anomaly effectively, and has considerable improvement comparing to the current state-of-the-art anomaly detection methods also based on generative models.
更多查看译文
关键词
Intrusion detection,High-speed railway,Anomaly detection,Generative models,Deep learning,Unsupervised learning
AI 理解论文
溯源树
样例
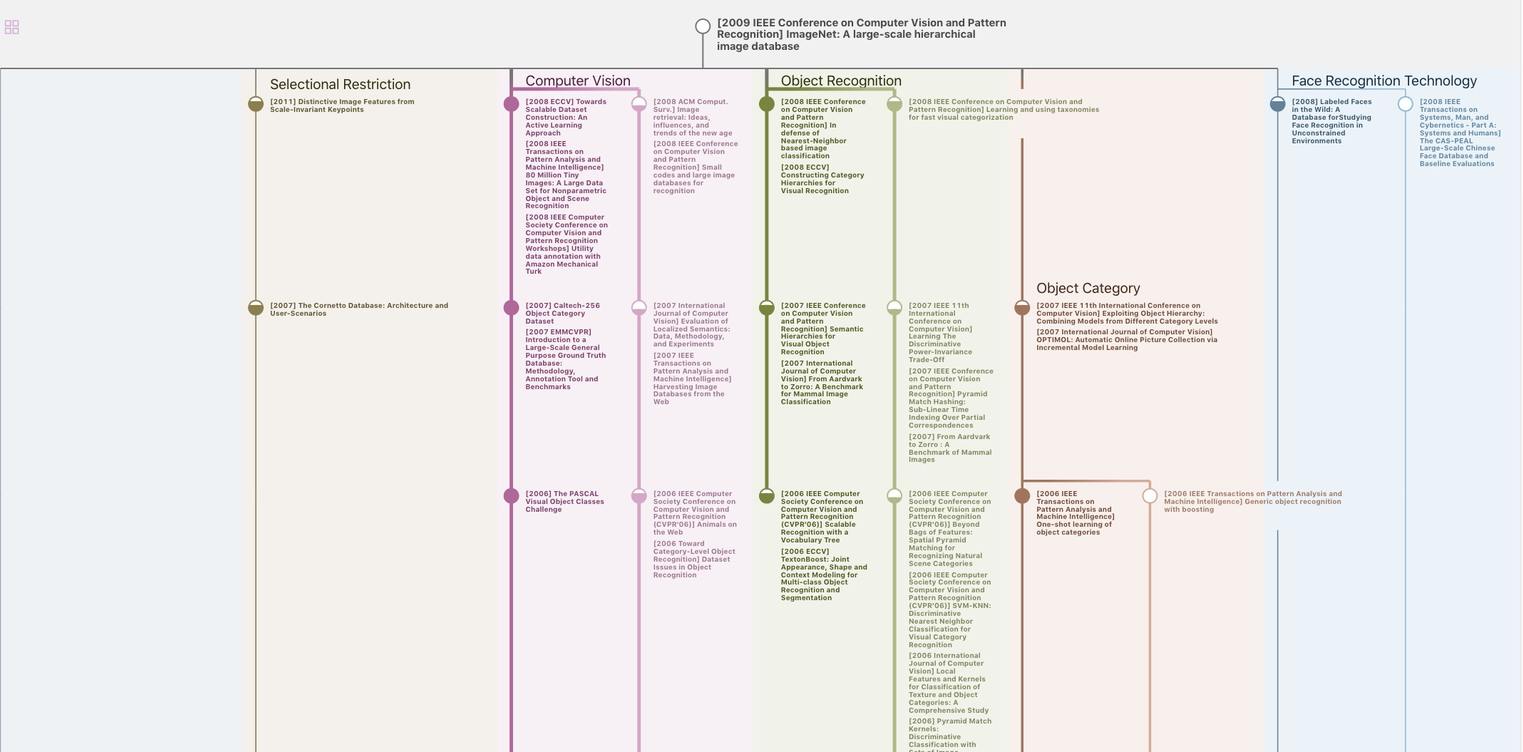
生成溯源树,研究论文发展脉络
Chat Paper
正在生成论文摘要