Leveraging external resources for offensive content detection in social media
AI COMMUNICATIONS(2022)
摘要
Hate speech is a burning issue of today's society that cuts across numerous strategic areas, including human rights protection, refugee protection, and the fight against racism and discrimination. The gravity of the subject is further demonstrated by Antonio Guterres, the United Nations Secretary-General, calling it "a menace to democratic values, social stability, and peace". One central platform for the spread of hate speech is the Internet and social media in particular. Thus, automatic detection of hateful and offensive content on these platforms is a crucial challenge that would strongly contribute to an equal and sustainable society when overcome. One significant difficulty in meeting this challenge is collecting sufficient labeled data. In our work, we examine how various resources can be leveraged to circumvent this difficulty. We carry out extensive experiments to exploit various data sources using different machine learning models, including state-of-the-art transformers. We have found that using our proposed methods, one can attain state-of-the-art performance detecting hate speech on Twitter (outperforming the winner of both the HASOC 2019 and HASOC 2020 competitions). It is observed that in general, adding more data improves the performance or does not decrease it. Even when using good language models and knowledge transfer mechanisms, the best results were attained using data from one or two additional data sets.
更多查看译文
关键词
Hateful and offensive language, deep language processing, transfer learning, vocabulary augmentation, RoBERTa
AI 理解论文
溯源树
样例
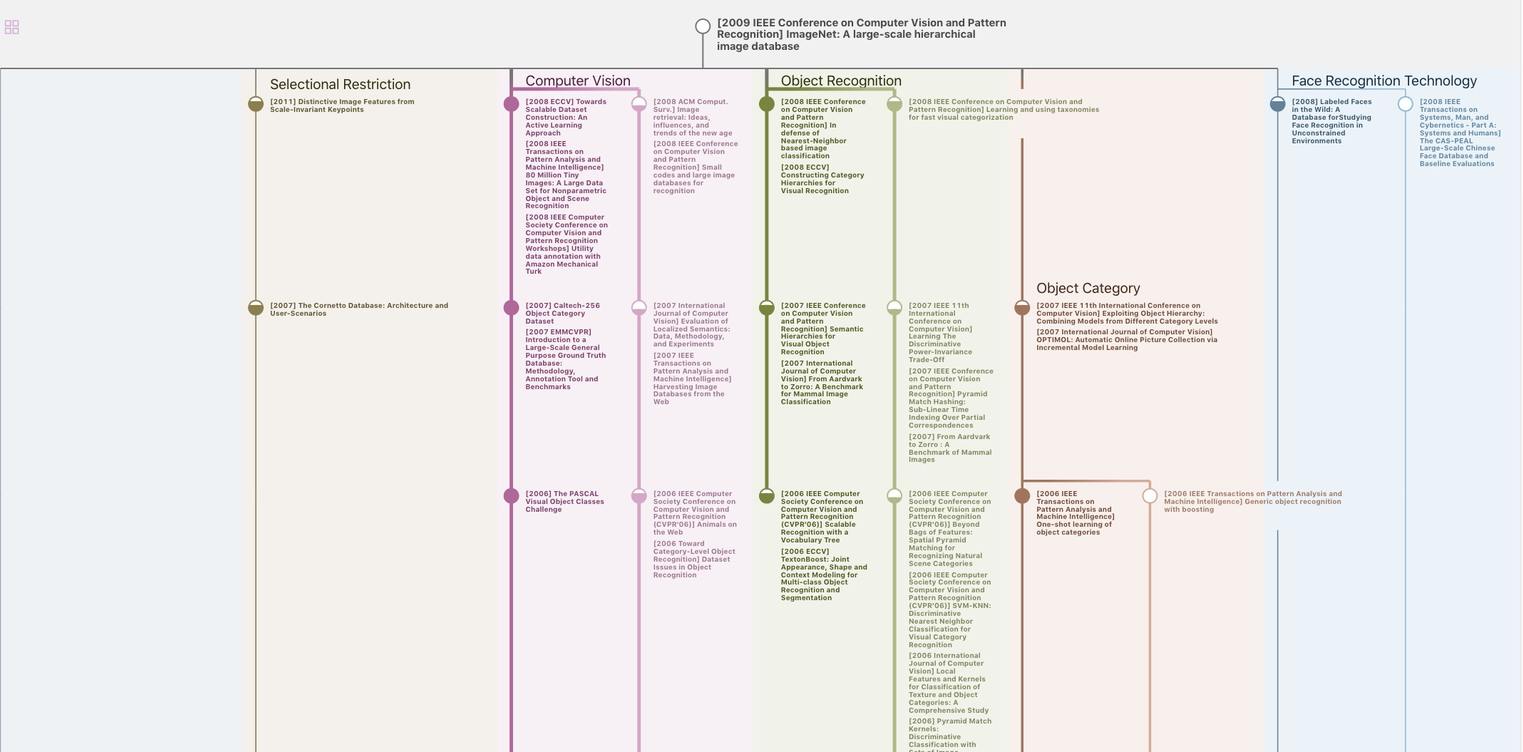
生成溯源树,研究论文发展脉络
Chat Paper
正在生成论文摘要