K-CDFs: A Nonparametric Clustering Algorithm via Cumulative Distribution Function
JOURNAL OF COMPUTATIONAL AND GRAPHICAL STATISTICS(2023)
摘要
We propose a novel partitioning clustering procedure based on the cumulative distribution function (CDF), called K-CDFs. For univariate data, the K-CDFs represent the cluster centers by empirical CDFs and assign each observation to the closest center measured by the Cramer-von Mises distance. The procedure is nonparametric and does not require assumptions on duster distributions imposed by mixture models. A projection technique is used to generalize the K-CDFs for univariate data to an arbitrary dimension. The proposed procedure has several appealing properties. It is robust to heavy-tailed data, is not sensitive to the data dimensions, does not require moment conditions on data and can effectively detect linearly non-separable clusters. To implement the K-CDFs, we propose two kinds of algorithms: a greedy algorithm as the classical Lloyd's algorithm and a spectral relaxation algorithm. We illustrate the finite sample performance of the proposed algorithms through simulation experiments and empirical analyses of several real datasets. Supplementary files for this article are available online.
更多查看译文
关键词
K-means, Nonparametric ANOVA, Nonparametric partitioning clustering, Projection mean variance, Spectral relaxation
AI 理解论文
溯源树
样例
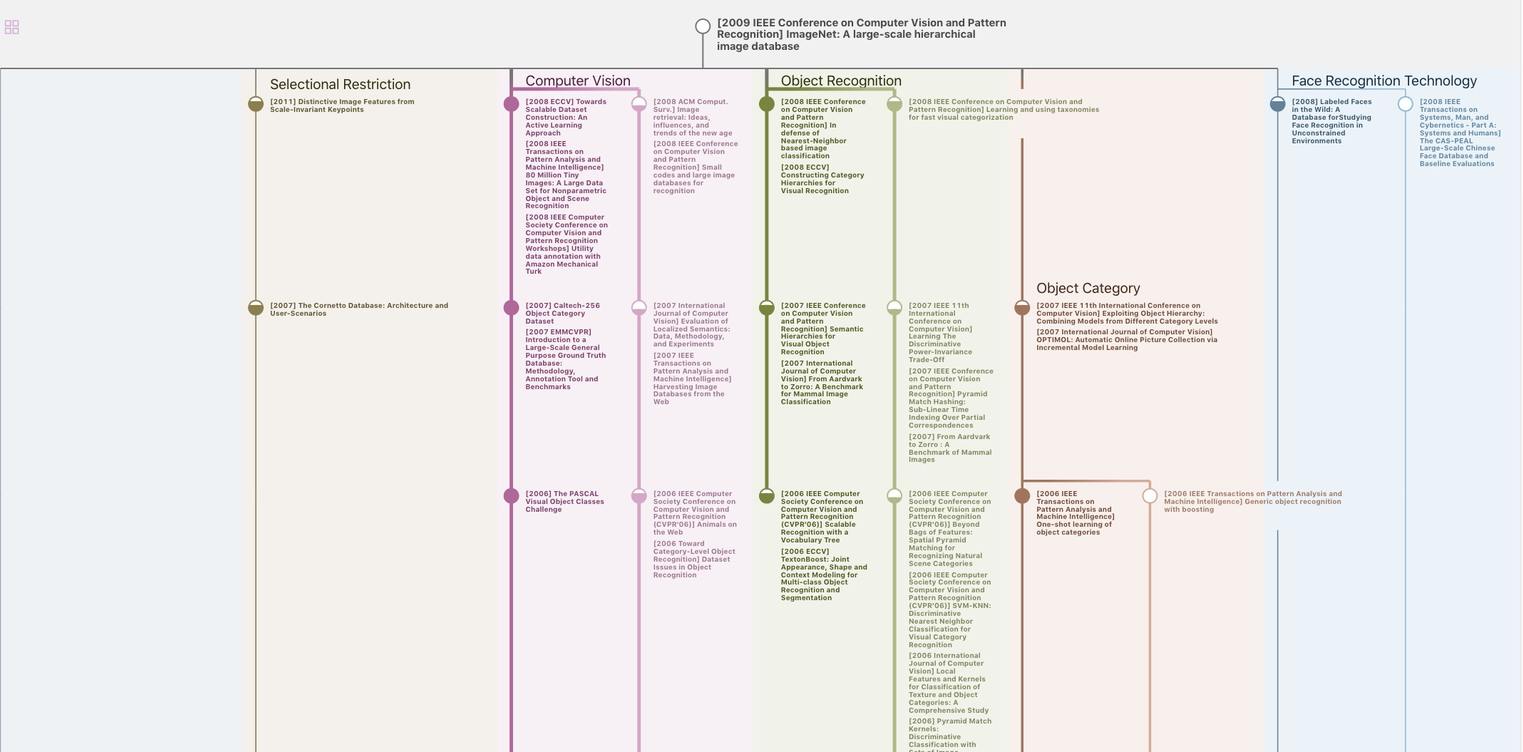
生成溯源树,研究论文发展脉络
Chat Paper
正在生成论文摘要