Deep learning framework for true amplitude imaging: Effect of conditioners and initial models
GEOPHYSICAL PROSPECTING(2024)
摘要
We propose a workflow to correct migration amplitudes by estimating the inverse Hessian operator weights using a neural network-based framework. We train the network such that it learns the transformation between the migration output and true amplitude reflectivity constrained by different conditioners. We analyse the network output with a velocity model and with source illumination as a conditioner. Compared to the velocity model, source illumination as a conditioner performs better because source illumination encodes the geometrical spreading information and accounts for non-stationarity. We further use the output of the deep neural network as a starting model for accelerating the convergence of iterative least-squares reverse time migration. Using a deep learning framework, the proposed method combines the model domain and data domain least-squares migration approaches to recover images with interpretable amplitudes, attenuated migration artefacts, better signal-to-noise ratio and improved resolution. We compare the output of the proposed algorithm with conventional least-squares output and show that the proposed workflow is more robust, especially in the areas with weak illumination.
更多查看译文
关键词
Deep neural networks,High-resolution seismic imaging,Least-squares migration,True amplitude imaging,Imaging,Seismics,Signal processing
AI 理解论文
溯源树
样例
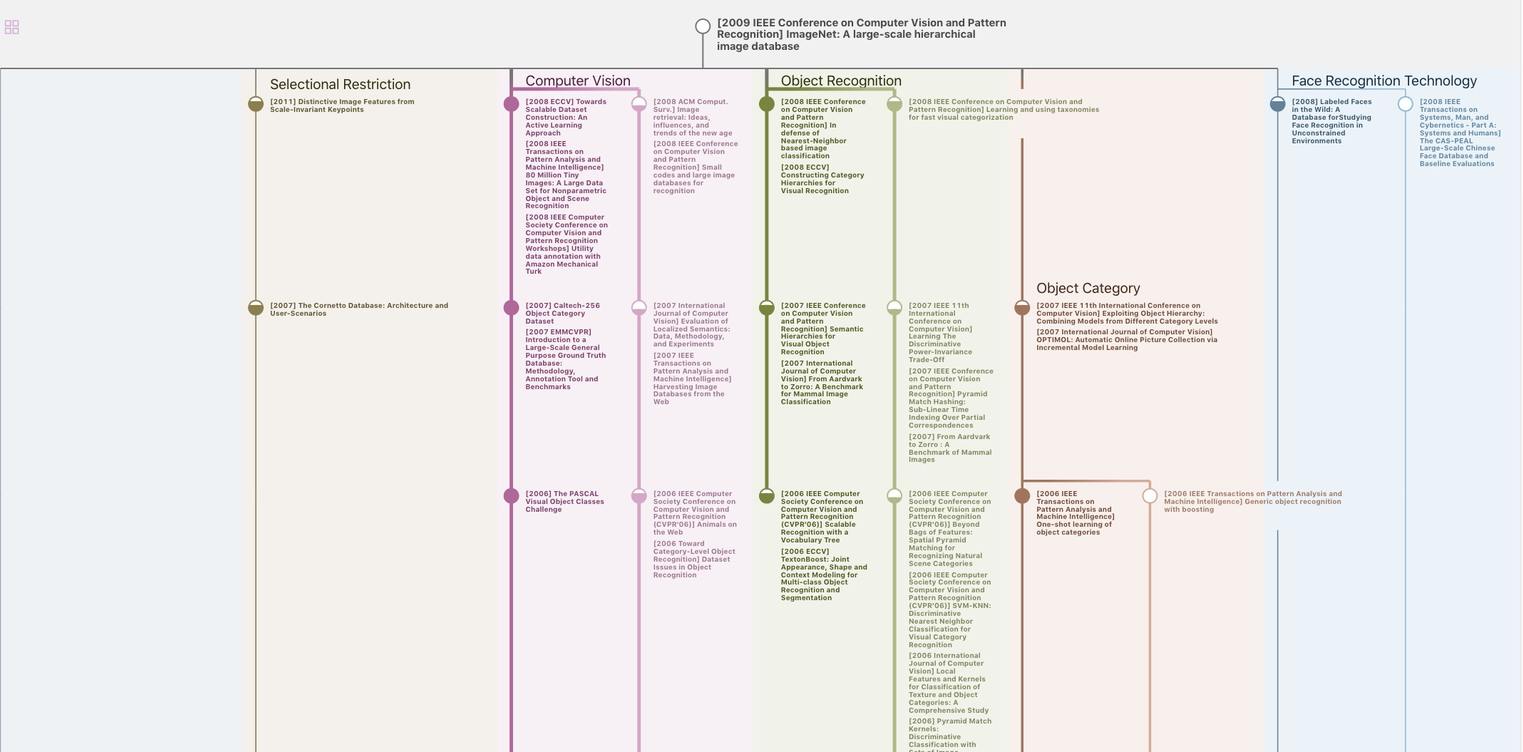
生成溯源树,研究论文发展脉络
Chat Paper
正在生成论文摘要