Semantic Correlation Attention-Based Multiorder Multiscale Feature Fusion Network for Human Motion Prediction
IEEE TRANSACTIONS ON CYBERNETICS(2024)
摘要
Human motion prediction is to predict future human states based on the observed human states. However, current research ignores the semantic correlations between body parts (joints and bones) in the observed human states and motion time; thus, the prediction accuracy is limited. To address this issue, we propose a novel semantic correlation attention-based multiorder multiscale feature fusion network (SCAFF), which includes an encoder and a decoder. In the encoder, a multiorder difference calculation module (MODC) is designed to calculate the multiorder difference information of joint and bone attributes in the observed human states. Then, multiple semantic correlation attention-based graph calculation operators (SCA-GCOs) are stacked to extract the multiscale features of the multiorder difference information. Each SCA-GCO captures joint and bone dependencies of the multiorder difference information, refines them with a semantic correlation attention module (SCAM), and captures temporal dynamics of the refined joint and bone dependencies as the output features. Note that SCAM learns a semantic attention mask describing the semantic correlations between body parts and motion time for feature refinement. Afterward, multiple multiorder feature fusion modules (MOFFs) and multiscale feature fusion modules (MSFFs) are designed to fuse the multiscale features of the multiorder difference information extracted by multiple SCA-GCOs, thus obtaining the motion features of the observed human states. Based on the obtained motion features, the decoder recurrently recruits a composite gated recurrent module (CGRM) and multilayer perceptrons (MLPs) to predict future human states. As far as we know, this is the first attempt to consider the semantic correlations between body parts and motion time in human motion prediction. The results on public datasets demonstrate that SCAFF outperforms existing models.
更多查看译文
关键词
Semantics,Feature extraction,Correlation,Joints,Predictive models,Bones,Decoding,Feature fusion,graph neural network (GNN),human motion prediction,semantic correlation attention
AI 理解论文
溯源树
样例
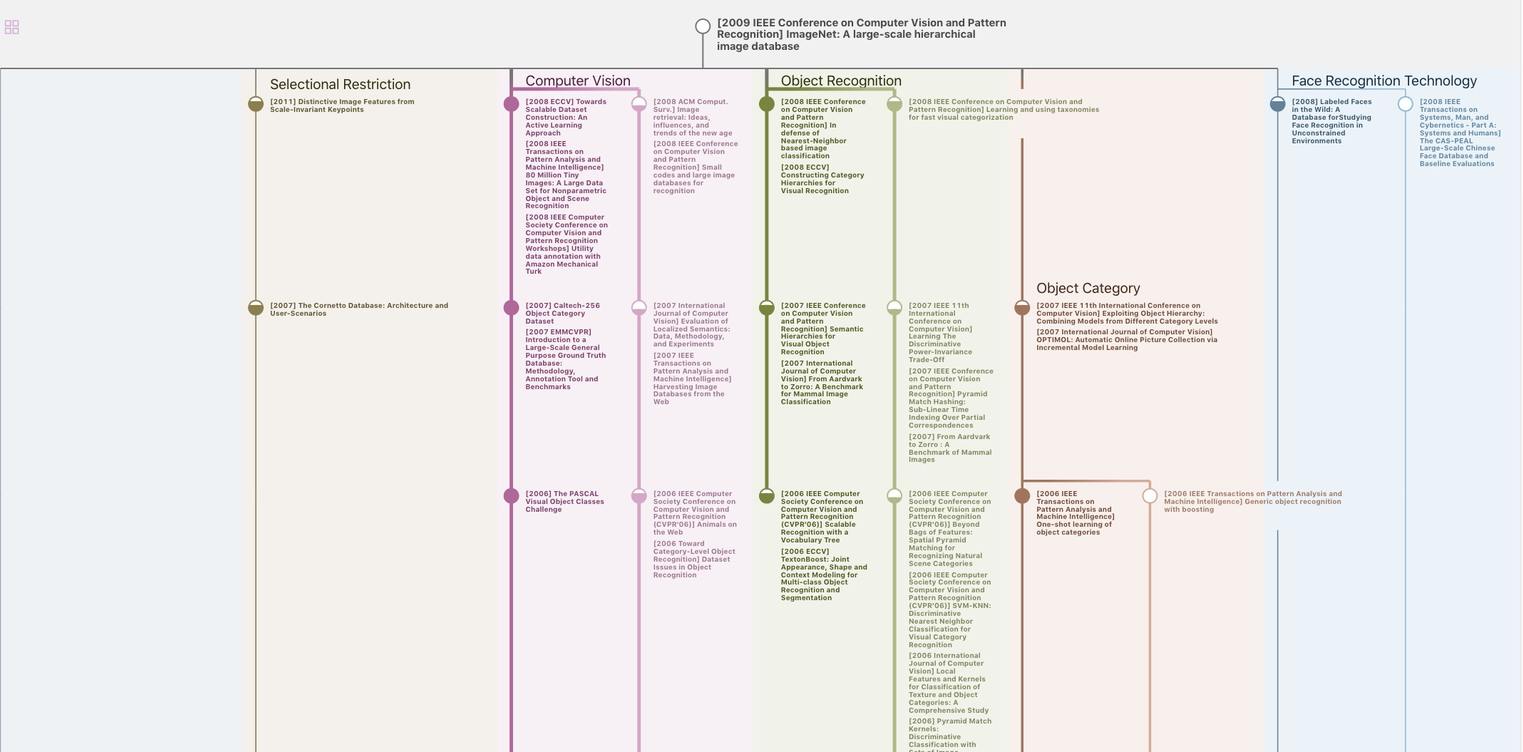
生成溯源树,研究论文发展脉络
Chat Paper
正在生成论文摘要