An efficient differential evolution with fitness-based dynamic mutation strategy and control parameters
Knowledge-Based Systems(2022)
摘要
It is known that the performance of the differential evolution (DE) algorithm highly depends on the mutation strategy and its control parameters. However, it is arduous to choose an appropriate mutation strategy and control parameters for a given optimization problem. Therefore, in this paper, an efficient framework of the DE named EFDE is proposed with a novel fitness-based dynamic mutation strategy and control parameters. This algorithm avoids the burden of selecting appropriate mutation strategy and control parameters and tries to maintain an appropriate balance between diversity and convergence. In the EFDE, the proposed mutation strategy adopts a dynamic number of fitness-based leading individuals to utilize the evolutionary state of the EFDE population for the evolution procedure. Furthermore, a new way of defining the control parameters is introduced based on the evolutionary state of each individual involved during the trial vector generation process. A comprehensive comparison of the proposed EFDE over challenging sets of problems from a well-known benchmark set of 23 problems, CEC2014, and CEC2017 real parameter single objective competition against several state-of-the-art algorithms is performed. The proposed EFDE is also used to solve four engineering design problems. Comparison and analysis of results confirm that the EFDE provides very competitive and better solution accuracy as compared to the other state-of-the-art algorithms.
更多查看译文
关键词
Global optimization,Differential evolution,Evolutionary state,Mutation strategy,Control parameters
AI 理解论文
溯源树
样例
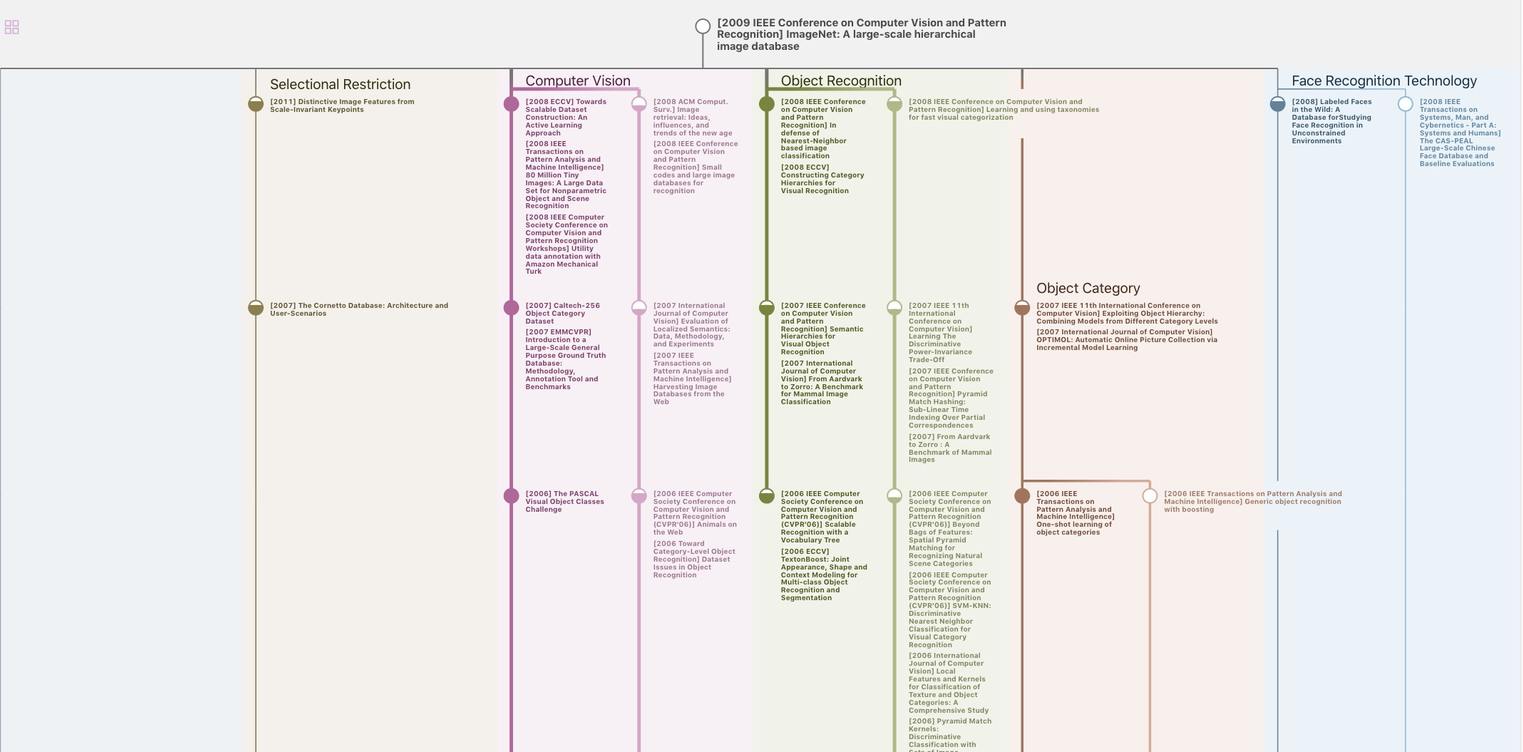
生成溯源树,研究论文发展脉络
Chat Paper
正在生成论文摘要