Combining detailed appearance and multi-scale representation: a structure-context complementary network for human pose estimation
Applied Intelligence(2022)
摘要
Human pose estimation is a fundamental and challenging task in the field of computer vision. Hard scenarios, such as occlusion and background confusion, set a great challenge for high-level feature representation because both detailed and multi-scale context must be correctly reasoned. In this paper, we propose a structure-context complementary network (SCC-Net) characterized by the complementarity between a pixel-wise enhanced attention mechanism and atrous convolution-based module. The proposed cross-coordinate attention bottleneck (CCAB) aims to utilize a cross-guide mechanism to promote the robustness of the existing coordinate attention module (CAM) for the background impact. As a complementary module for CCAB, waterfall residual atrous pooling (WRAP) is proposed to refine structure consistency by generating multi-scale features without the feature sparse defect of atrous-based methods. We evaluate our proposed modules and holistic SCC-Net on the COCO and MPII benchmark datasets. Ablation experiments demonstrate that our proposed modules can efficiently boost the performance of body joint detection. Competitive performance is also achieved by our holistic SCC-Net compared to other state-of-the-art methods.
更多查看译文
关键词
Pose estimation, Structure-context enhancement, Attention mechanism, Atrous convolution-based module
AI 理解论文
溯源树
样例
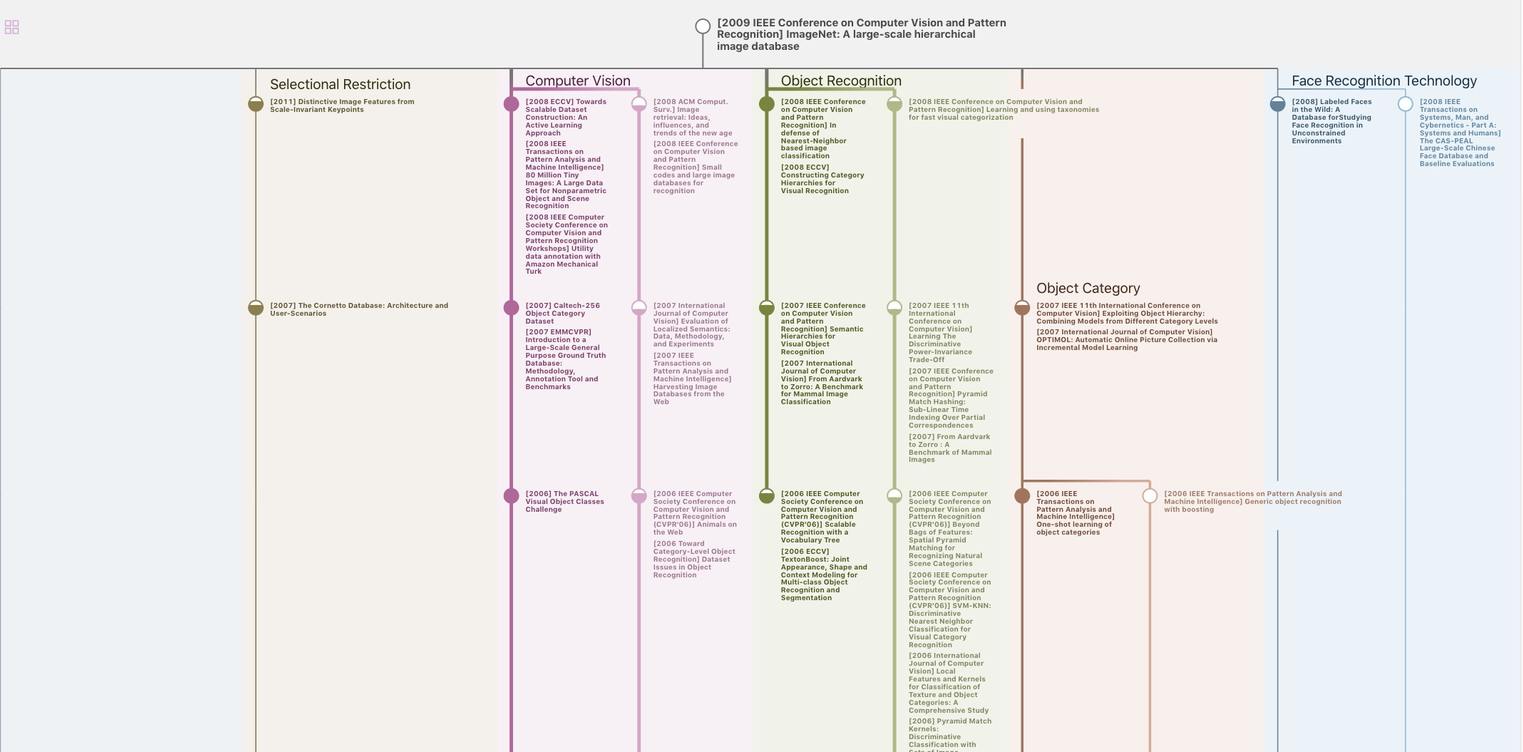
生成溯源树,研究论文发展脉络
Chat Paper
正在生成论文摘要