A scenario-based desirability function for correlated multi-response optimization problems considering modeling and implementation errors
Journal of Computational Science(2022)
摘要
Simultaneous improvement of multiple quality characteristics is one of the main concerns in today's complex industrial systems. Multiresponse optimization enables us to address this concern and find a compromise solution of design factors that leads to desirable quality outputs. This problem becomes complicated when some degree of uncertainty affects the result of the experiments. In this paper, two sources of uncertainty are included in multi-response optimization problems. The first is experimental errors related to unknown variables, also called environmental nuisances, creating response surface modeling errors. The second source is errors in implementation. In the experimentation, the controllable factors are mainly set at some predefined levels, but after the optimal design has been found, it oscillates around that value during the implementation phase. To handle this problem, a scenario-based two-stage stochastic programming is developed in which desirability functions are aggregated to form the final objective function. Scenarios are generated by sampling from probability distribution functions of the response surfaces' coefficients under normality assumptions. The numerical results show that joint consideration of the error sources leads to a smaller range of desirability values among the different scenarios and a higher value of the total desirability index.
更多查看译文
关键词
Multi-response optimization,Robust design,Implementation error,Stochastic programming,Desirability function
AI 理解论文
溯源树
样例
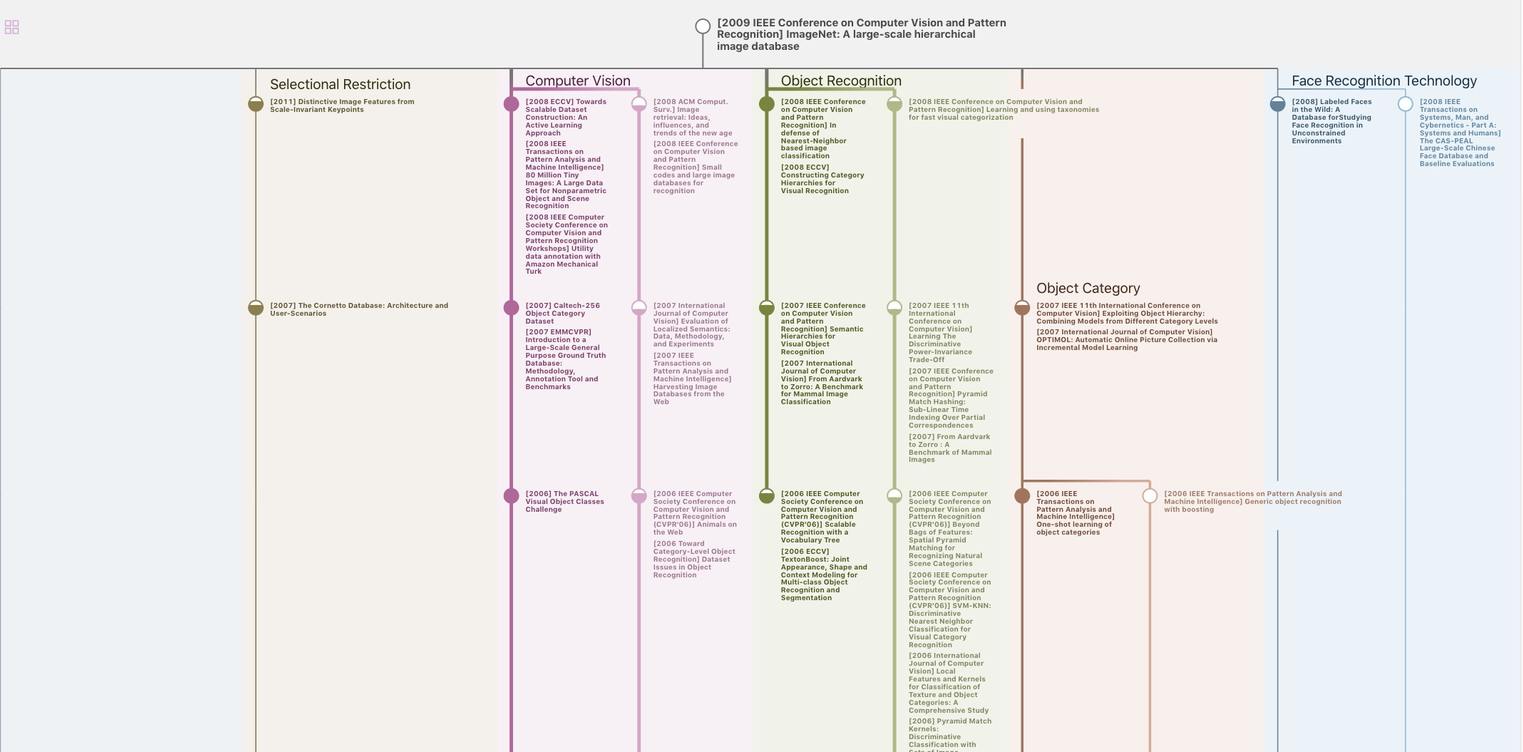
生成溯源树,研究论文发展脉络
Chat Paper
正在生成论文摘要