A general framework for enhancing relaxed Pareto dominance methods in evolutionary many-objective optimization
NATURAL COMPUTING(2022)
摘要
In the last decade, it is widely known that the Pareto dominance-based evolutionary algorithms (EAs) are unable to deal with many-objective optimization problems (MaOPs) well, as it is hard to maintain a good balance between convergence and diversity. Instead, most researchers in this domain tend to develop EAs that do not rely on Pareto dominance (e.g., decomposition-based and indicator-based techniques) to solve MaOPs. However, it is still hard for these non-Pareto-dominance-based methods to solve MaOPs with unknown irregular PF shapes. In this paper, we develop a general framework for enhancing relaxed Pareto dominance methods to solve MaOPs, which can promote both convergence and diversity. During the environmental selection step, we use M different cases of relaxed Pareto dominance simultaneously, where each expands the dominance area of solutions for M - 1 objectives to improve the selection pressure, while the remaining one objective keeps unchanged. We conduct the experiments on a variety of test problems, the result shows that our proposed framework can obviously improve the performance of relaxed Pareto dominance in solving MaOPs, and is very competitive against or outperform some state-of-the-art many-objective EAs.
更多查看译文
关键词
Evolutionary algorithms, Many-objective optimization, Pareto dominance, Enhance selection pressure
AI 理解论文
溯源树
样例
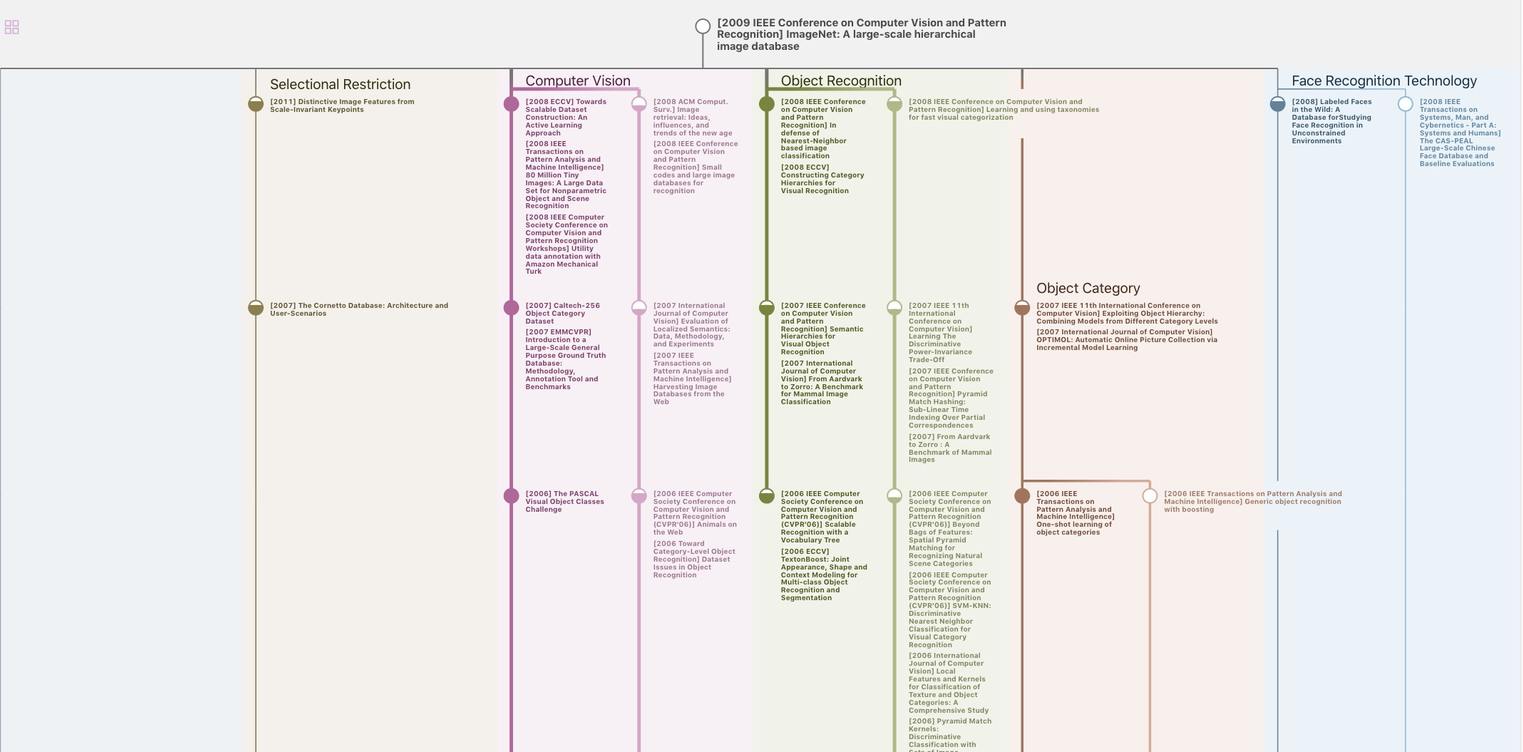
生成溯源树,研究论文发展脉络
Chat Paper
正在生成论文摘要