Evidence Mining for Interpretable Charge Prediction via Prompt Learning
IEEE TRANSACTIONS ON COMPUTATIONAL SOCIAL SYSTEMS(2022)
摘要
Nowadays, more and more researchers are committed to applying artificial intelligence technology to the legal field to support decision-making. Charge prediction is a subtask of legal judgment prediction (LJP). Its purpose is to analyze the fact description of natural language text and predict a charge corresponding to a case. At present, most of the research takes charge prediction as a multiclass classification task, which leads to unsatisfactory interpretation due to the weak semantic correlation between the fact descriptions and charge labels. In order to solve this problem, we propose a method of generative evidence mining based on prompt learning. Specifically, in the training phase, we reformulate the charge labels into the prompt template that we design to enhance the semantic correlation between the charge labels and the fact descriptions. In the testing phase, the charge labels are generated via the model based on prompt learning. Meanwhile, we calculate the attention score of each sentence from the multihead self-attention in the transformer encoder and choose the sentence with the highest attention score as the evidence. Our experimental results on a real dataset show that our method is better than the traditional fine-tuning-based classification method.
更多查看译文
关键词
Task analysis, Predictive models, Law, Semantics, Visualization, Neural networks, Computational modeling, Charge prediction, interpretability, prompt learning
AI 理解论文
溯源树
样例
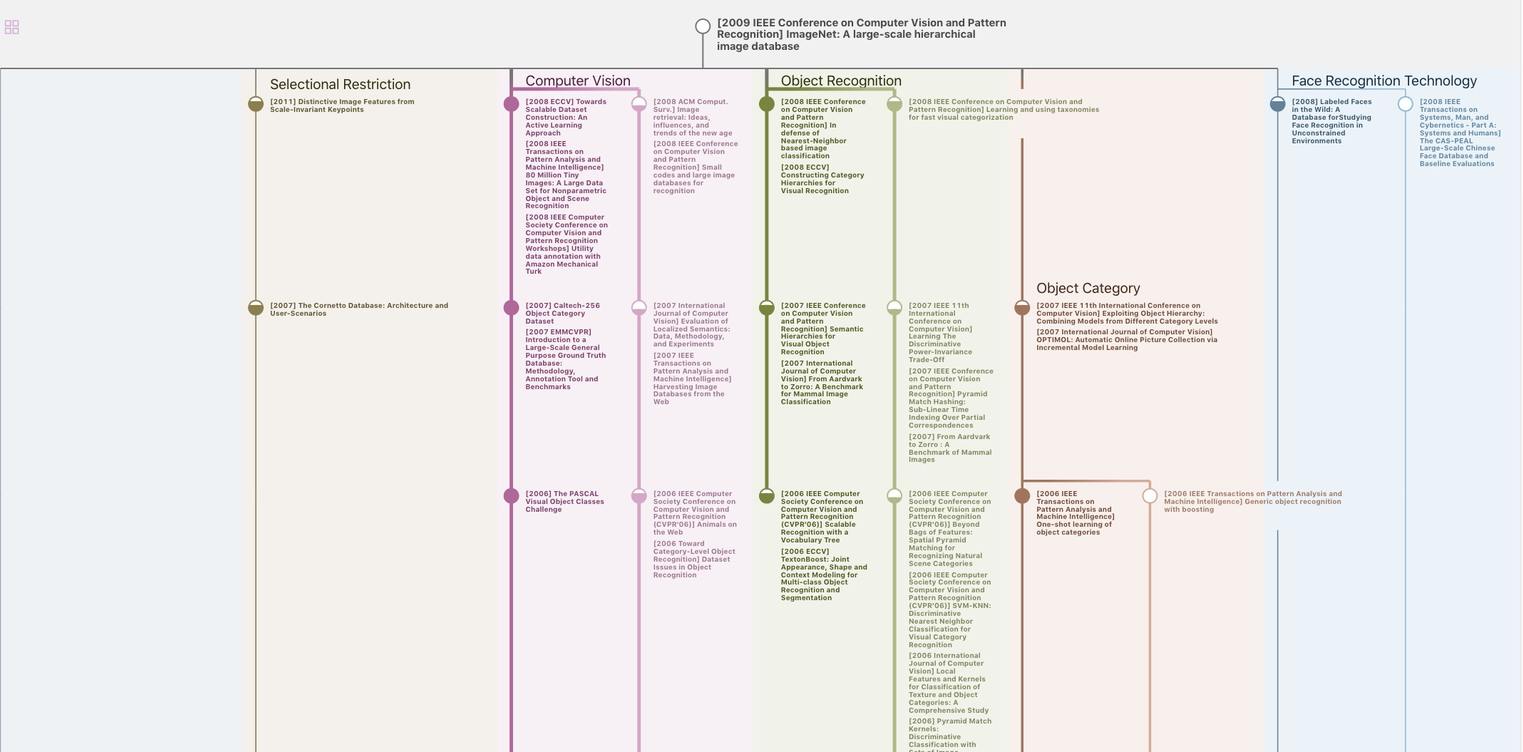
生成溯源树,研究论文发展脉络
Chat Paper
正在生成论文摘要