Enhancing deep learning algorithm accuracy and stability using multicriteria optimization: an application to distributed learning with MNIST digits
ANNALS OF OPERATIONS RESEARCH(2022)
摘要
The training phase is the most crucial stage during the machine learning process. In the case of labeled data and supervised learning, machine learning entails minimizing the loss function under various constraints. We provide an innovative model for learning with numerous data sets, resulting from the application of multicriteria optimization techniques to existing deep learning algorithms. Data fitting is formulated as a multicriteria model in which each criterion measures the data fitting error on a specific data set. This is an optimization model involving a vector-valued function, and it has to be analyzed using the notion of Pareto efficiency. We present stability results for efficient solutions in the presence of input and output data perturbations. The multiple data set environment comes into play to eliminate the bias caused by the selection of a specific training set. To apply this concept, we present a scalarization strategy as well as numerical experiments in digit classification using MNIST data.
更多查看译文
关键词
Artificial intelligence,Deep learning,Machine learning,Multicriteria optimization,Classification,MINST data
AI 理解论文
溯源树
样例
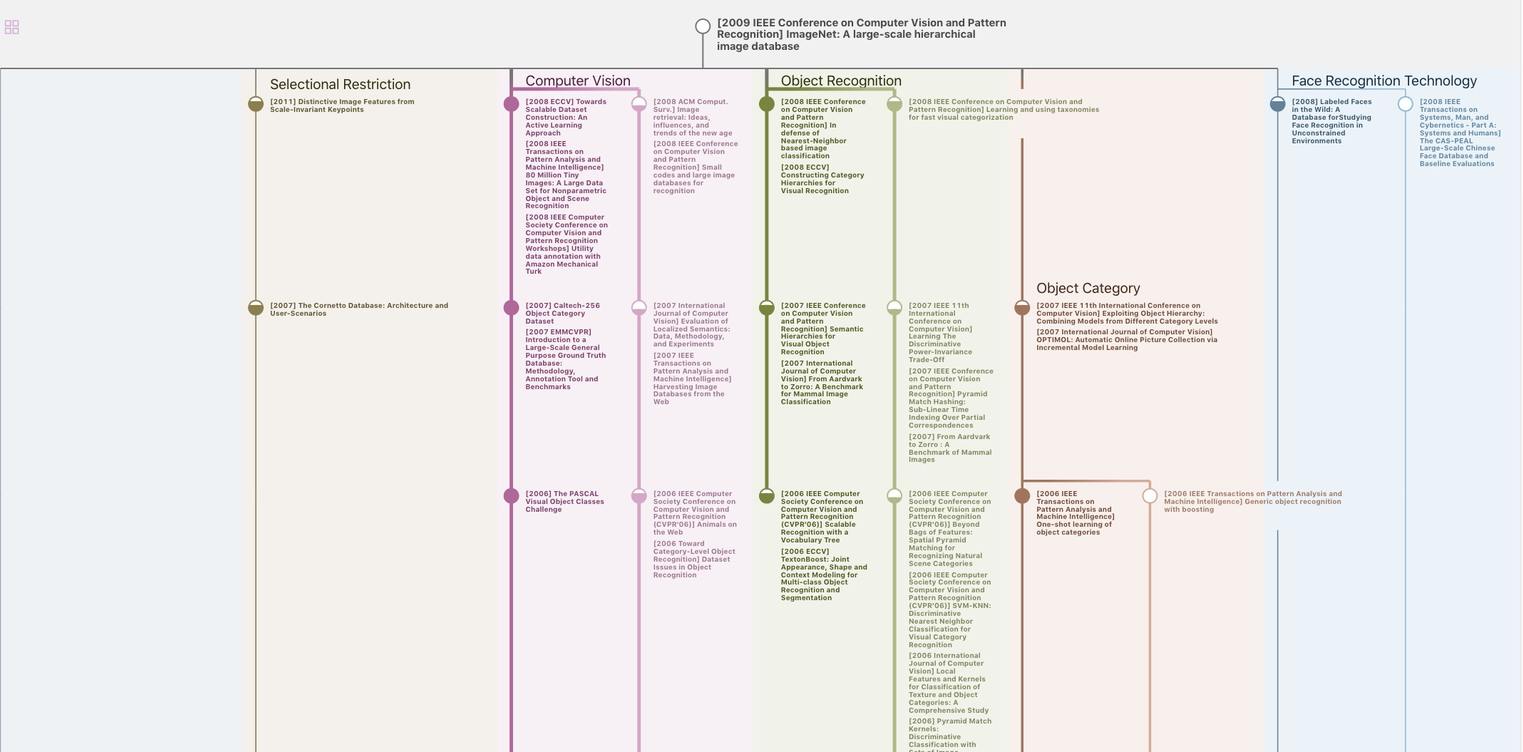
生成溯源树,研究论文发展脉络
Chat Paper
正在生成论文摘要