ML-Based Performance Modeling in SDN-Enabled Data Center Networks
IEEE Transactions on Network and Service Management(2023)
摘要
Traffic optimization and smart buffering are fundamental to achieve both great application performance and resource efficiency in data centers with heterogeneous workloads, including incast and elephant traffics. However, general performance models providing insights on how various factors affect traffic performance metrics needed by these management functions are missing. For the special case of incast, the existing models are analytical ones, either tightly coupled with a particular protocol version or specific to certain empirical data. Motivated by this observation, this paper proposes an SDN-enabled machine-learning-based performance modeling approach in data center networks that leverages random forest predictions. Evaluations based on datasets constructed through intensive NS-3 simulations show that we can achieve accurate predictions of incast and elephant performance metrics based on various features. With this performance modeling capability, smart buffering schemes or traffic optimization algorithms could anticipate and efficiently optimize system parameters adjustment to achieve optimal performance continuously in data centers.
更多查看译文
关键词
Data centers,TCP incast,elephant traffic,SDN,machine learning,performance modeling
AI 理解论文
溯源树
样例
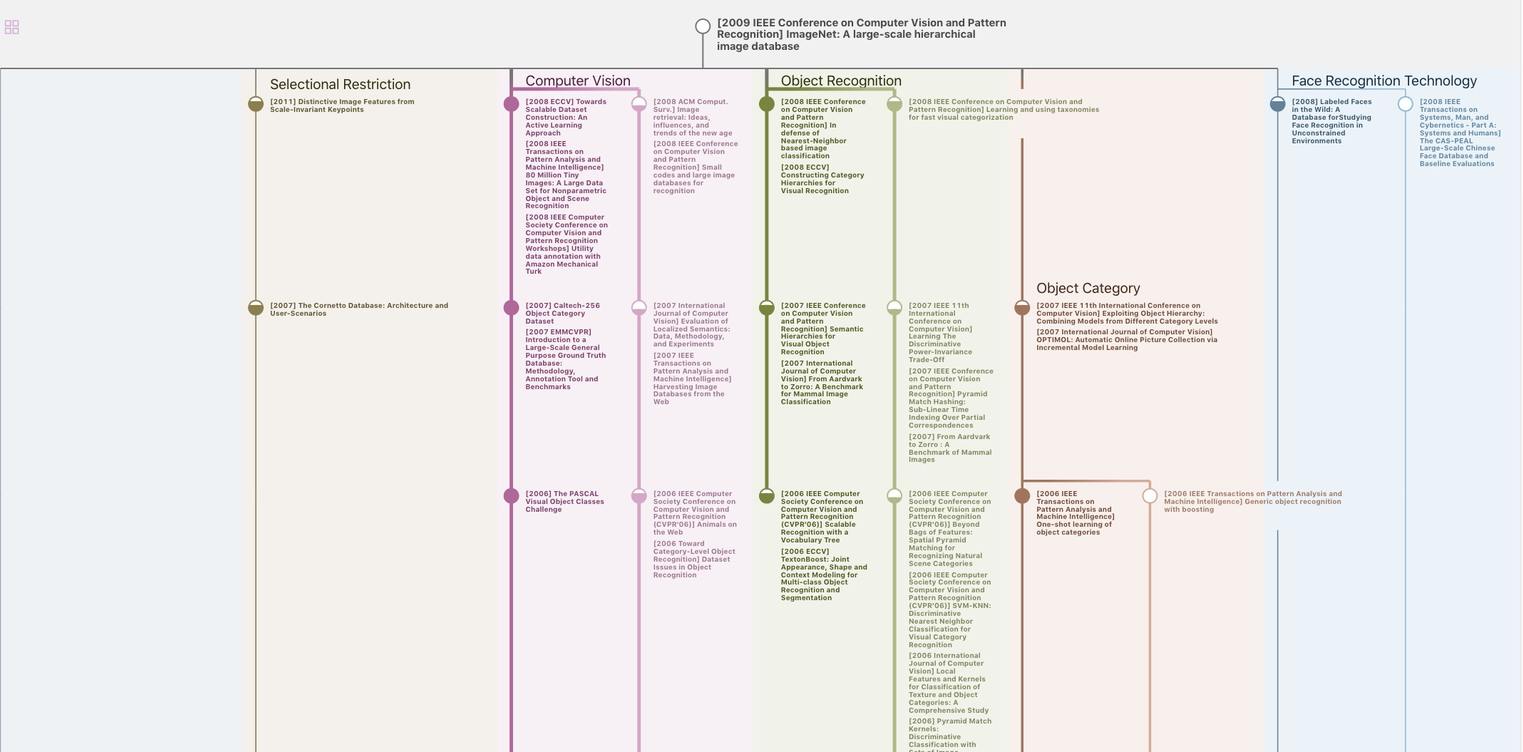
生成溯源树,研究论文发展脉络
Chat Paper
正在生成论文摘要