A Frequency-aware Software Cache for Large Recommendation System Embeddings
arxiv(2022)
摘要
Deep learning recommendation models (DLRMs) have been widely applied in Internet companies. The embedding tables of DLRMs are too large to fit on GPU memory entirely. We propose a GPU-based software cache approaches to dynamically manage the embedding table in the CPU and GPU memory space by leveraging the id's frequency statistics of the target dataset. Our proposed software cache is efficient in training entire DLRMs on GPU in a synchronized update manner. It is also scaled to multiple GPUs in combination with the widely used hybrid parallel training approaches. Evaluating our prototype system shows that we can keep only 1.5% of the embedding parameters in the GPU to obtain a decent end-to-end training speed.
更多查看译文
关键词
large recommendation system
AI 理解论文
溯源树
样例
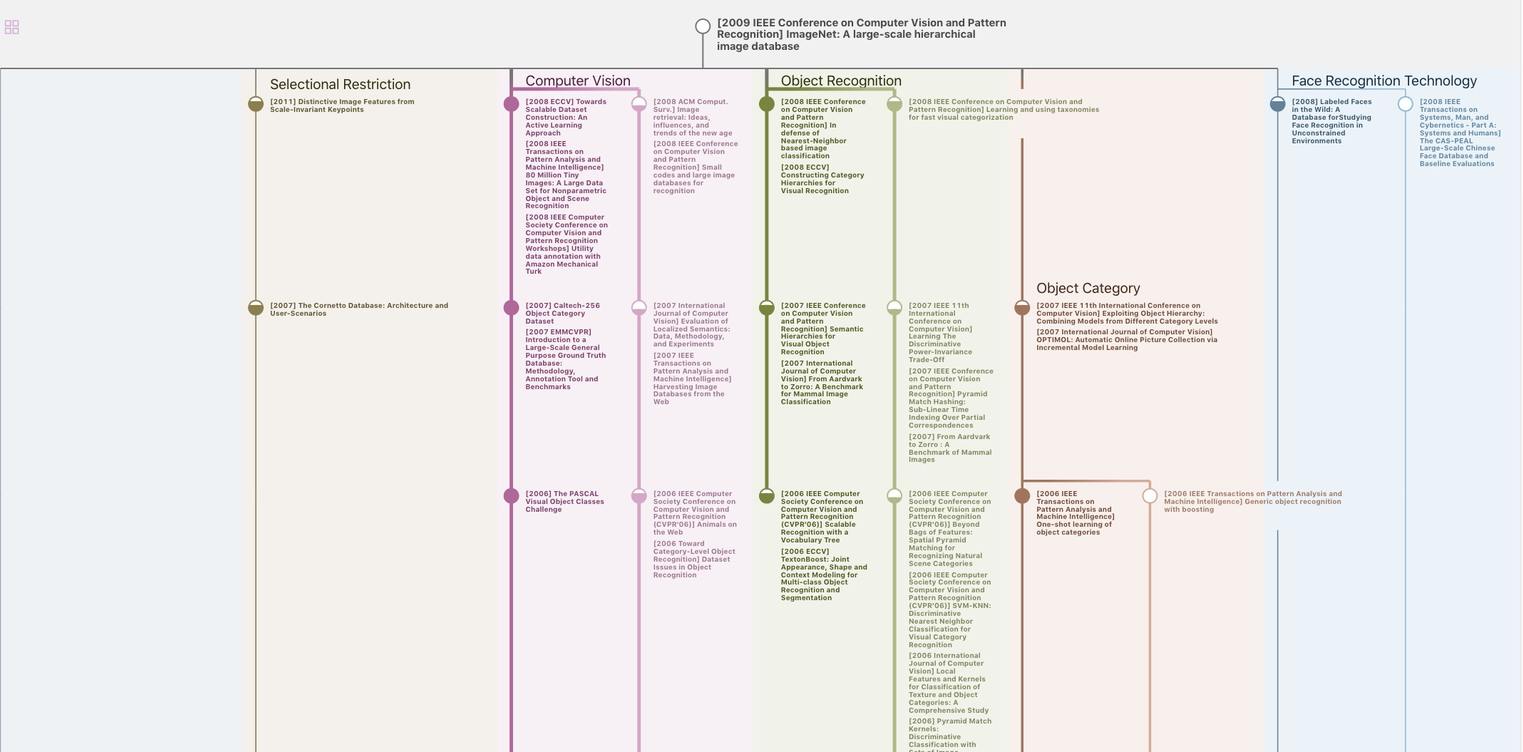
生成溯源树,研究论文发展脉络
Chat Paper
正在生成论文摘要