Semi-Supervised Junction Tree Variational Autoencoder for Molecular Property Prediction
arxiv(2023)
摘要
Molecular Representation Learning is essential to solving many drug discovery and computational chemistry problems. It is a challenging problem due to the complex structure of molecules and the vast chemical space. Graph representations of molecules are more expressive than traditional representations, such as molecular fingerprints. Therefore, they can improve the performance of machine learning models. We propose SeMole, a method that augments the Junction Tree Variational Autoencoders, a state-of-the-art generative model for molecular graphs, with semi-supervised learning. SeMole aims to improve the accuracy of molecular property prediction when having limited labeled data by exploiting unlabeled data. We enforce that the model generates molecular graphs conditioned on target properties by incorporating the property into the latent representation. We propose an additional pre-training phase to improve the training process for our semi-supervised generative model. We perform an experimental evaluation on the ZINC dataset using three different molecular properties and demonstrate the benefits of semi-supervision.
更多查看译文
关键词
property prediction,molecular
AI 理解论文
溯源树
样例
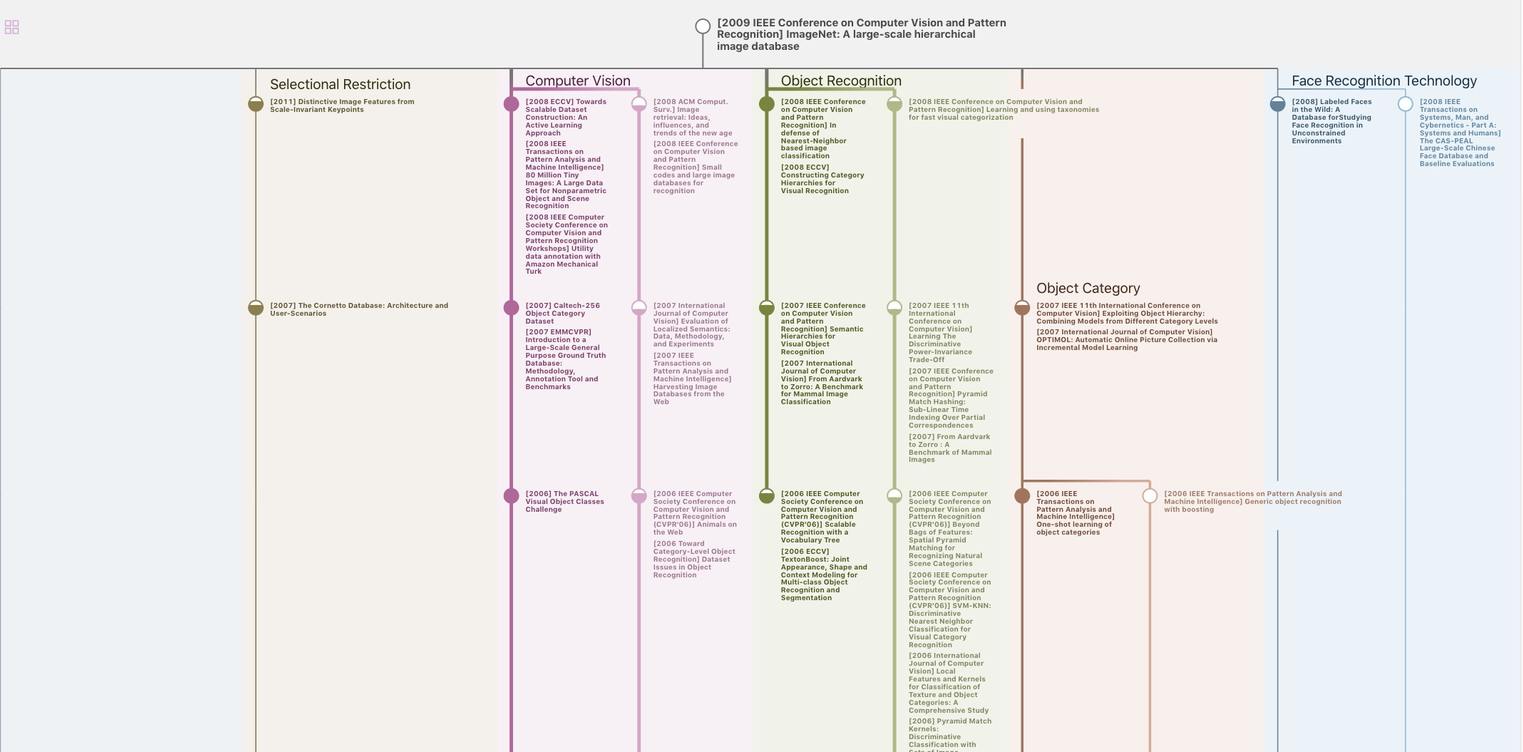
生成溯源树,研究论文发展脉络
Chat Paper
正在生成论文摘要