Recycling an anechoic pre-trained speech separation deep neural network for binaural dereverberation of a single source
arxiv(2022)
摘要
Reverberation results in reduced intelligibility for both normal and hearing-impaired listeners. This paper presents a novel psychoacoustic approach of dereverberation of a single speech source by recycling a pre-trained binaural anechoic speech separation neural network. As training the deep neural network (DNN) is a lengthy and computationally expensive process, the advantage of using a pre-trained separation network for dereverberation is that the network does not need to be retrained, saving both time and computational resources. The interaural cues of a reverberant source are given to this pretrained neural network to discriminate between the direct path signal and the reverberant speech. The results show an average improvement of 1.3% in signal intelligibility, 0.83 dB in SRMR (signal to reverberation energy ratio) and 0.16 points in perceptual evaluation of speech quality (PESQ) over other state-of-the-art signal processing dereverberation algorithms and 14% in intelligibility and 0.35 points in quality over orthogonal matching pursuit with spectral subtraction (OSS), a machine learning based dereverberation algorithm.
更多查看译文
关键词
binaural dereverberation,speech separation,pre-trained
AI 理解论文
溯源树
样例
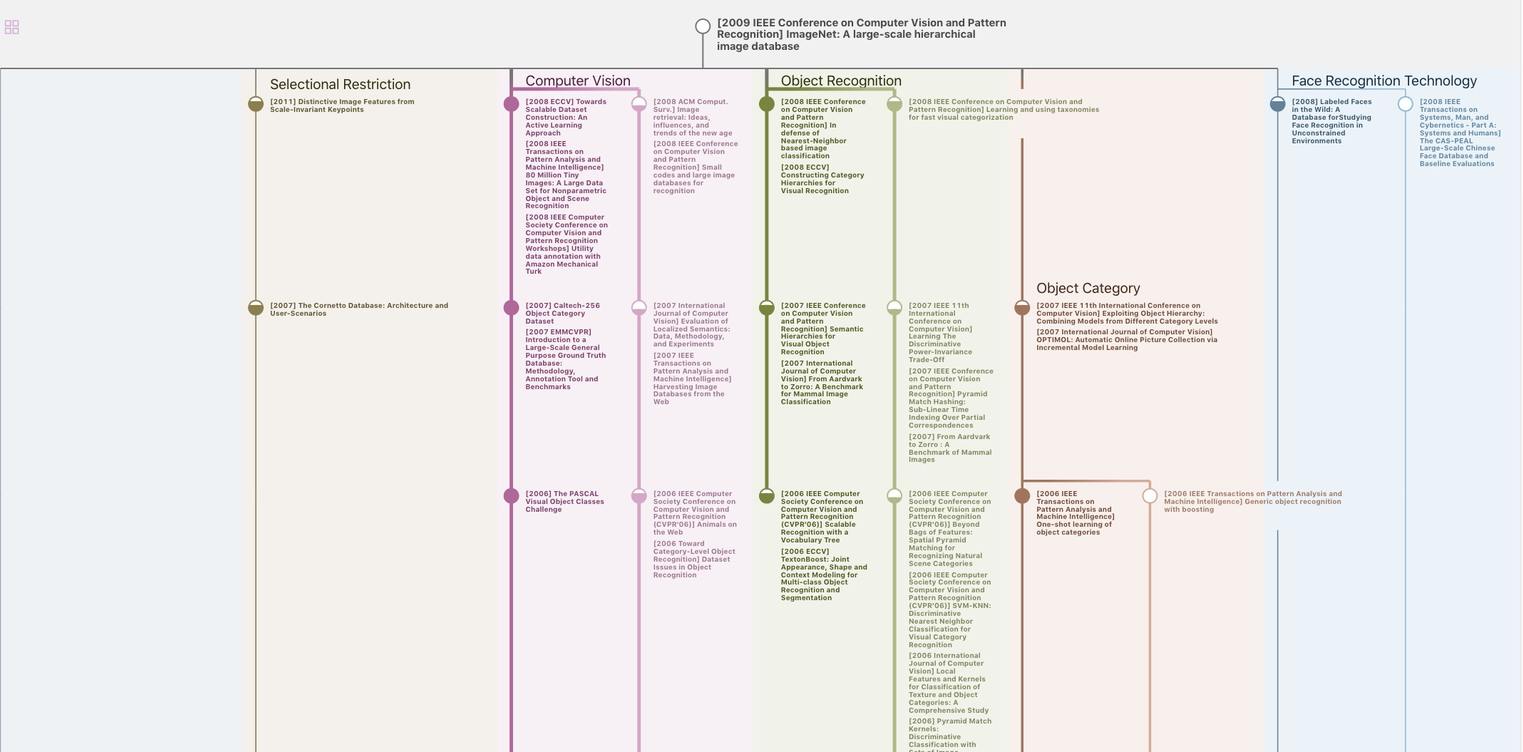
生成溯源树,研究论文发展脉络
Chat Paper
正在生成论文摘要