Beam Pattern Fingerprinting with Missing Features for Spoofing Attack Detection in Millimeter-Wave Networks.
Wireless Network Security (WISEC)(2022)
摘要
As one of the key enabling technologies of 5G wireless communication, millimeter-Wave (mmWave) technology unlocks the ultra-wide bandwidth opportunity in supporting high-throughput (e.g., multi-Gbps) and ultra-low latency applications at much lower cost-per-bit. However, due to the broadcast nature of wireless medium, like sub-6GHz communication, mmWave communication is still subject to various attacks, such as the identity spoofing attacks. Recently, beam pattern fingerprinting using the signal-to-noise-ratio (SNR) traces obtained during the beam sweeping process has been proposed to detect spoofing attacks in mmWave networks. However, a complete beam sweeping that tests all the tx-rx beam pairs is not always applied in practice. That is, to save link initialization or maintenance overhead, the implementation of efficient beam management schemes usually only probes a subset of tx-rx beam pairs. Therefore, SNR traces could randomly miss some features, which will bring challenges for fingerprint training as well as online prediction. In this work, we build a machine learning model that can achieve a fast and highly-accurate identity spoofing detection under missing features. Experimental results show that our proposed approach can achieve a high detection accuracy of almost 100% with short detection delay under various feature missing patterns. In addition, it improves the performance (i.e., accuracy, precision, recall, F1-score) of co-located attack detection by over 18% under missing features, compared to the model trained on data with only complete features.
更多查看译文
关键词
5G,spoofing attack detection,feature selection,ADMM,machine learning
AI 理解论文
溯源树
样例
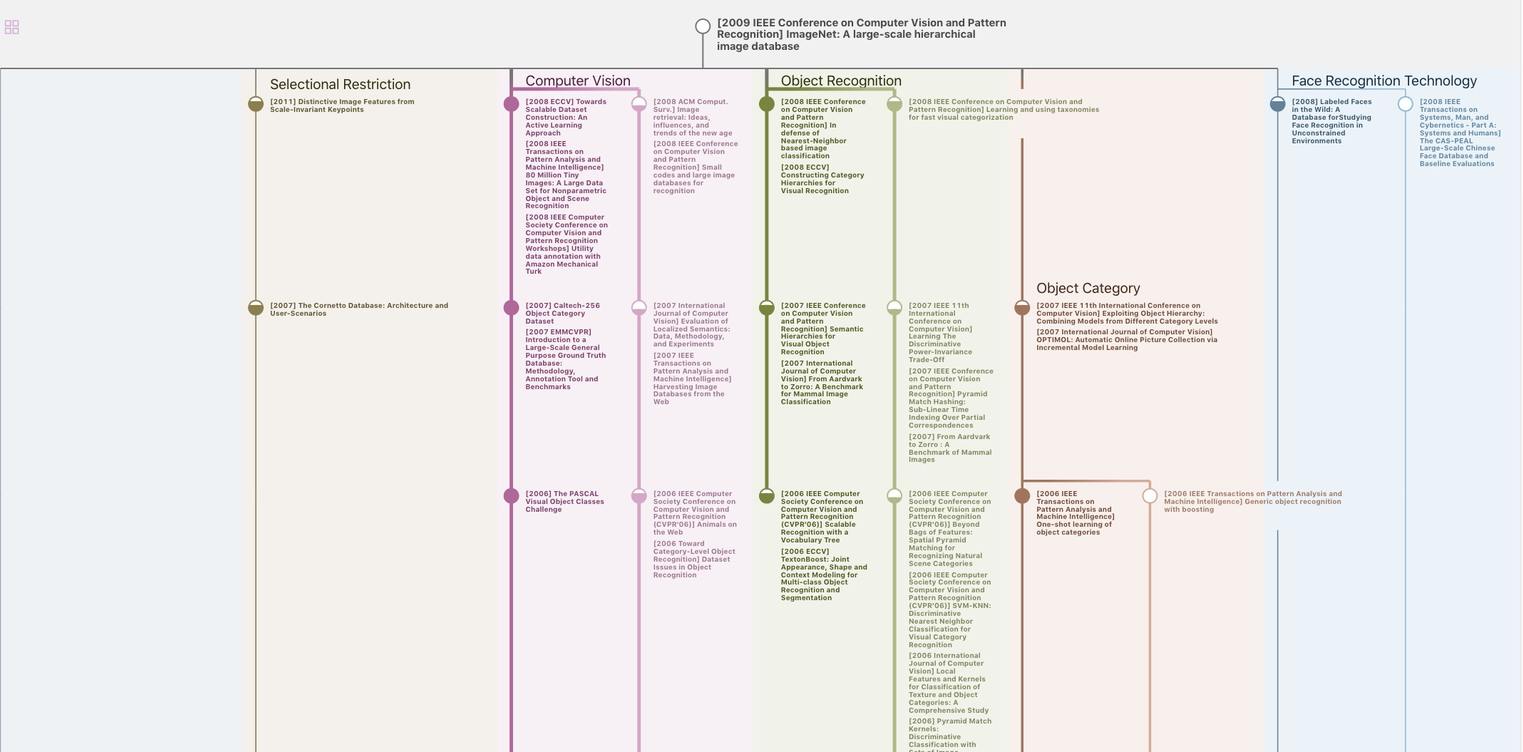
生成溯源树,研究论文发展脉络
Chat Paper
正在生成论文摘要