A Concurrent Multiscale Detector for End-to-End Image Matching
IEEE TRANSACTIONS ON NEURAL NETWORKS AND LEARNING SYSTEMS(2024)
摘要
This article focuses on end-to-end image matching through joint key-point detection and descriptor extraction. To find repeatable and high discrimination key points, we improve the deep matching network from the perspectives of network structure and network optimization. First, we propose a concurrent multiscale detector (CS-det) network, which consists of several parallel convolutional networks to extract multiscale features and multilevel discriminative information for key-point detection. Moreover, we introduce an attention module to fuse the response maps of various features adaptively. Importantly, we propose two novel rank consistent losses (RC-losses) for network optimization, significantly improving image matching performances. On the one hand, we propose a score rank consistent loss (RC-S-loss) to ensure that the key points have high repeatability. Different from the score difference loss merely focusing on the absolute score of an individual key point, our proposed RC-S-loss pays more attention to the relative score of key points in the image. On the other hand, we propose a score-discrimination RC-loss to ensure that the key point has high discrimination, which can reduce the confusion from other key points in subsequent matching and then further enhance the accuracy of image matching. Extensive experimental results demonstrate that the proposed CS-det improves the mean matching result of deep detector by 1.4%-2.1%, and the proposed RC-losses can boost the matching performances by 2.7%-3.4% than score difference loss. Our source codes are available at https://github.com/iquandou/CS-Net.
更多查看译文
关键词
Detectors,Feature extraction,Image matching,Optimization,Learning systems,Lighting,Deep learning,Concurrent detector,end-to-end learning,image matching,key-point detection,multilevel features,multiscale features,rank consistent
AI 理解论文
溯源树
样例
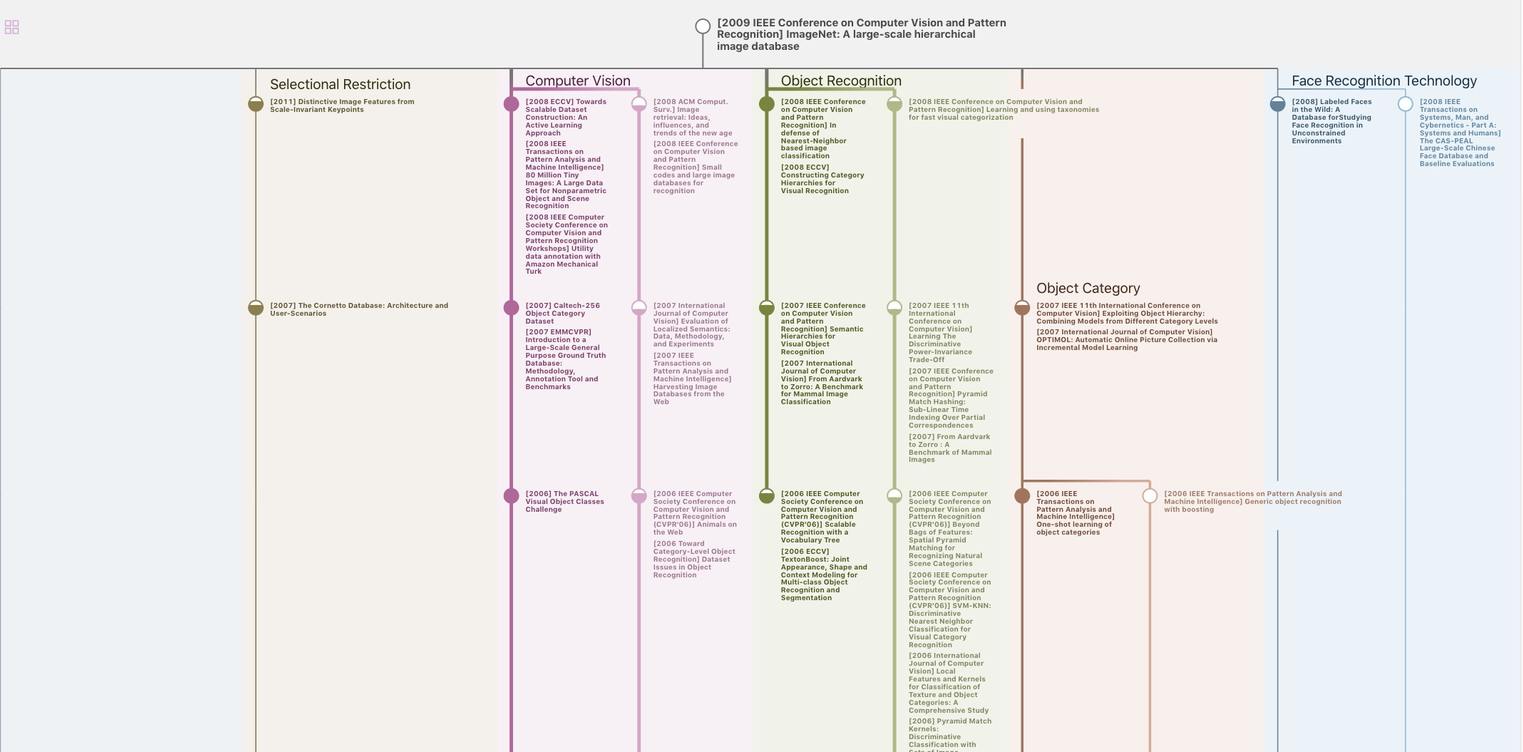
生成溯源树,研究论文发展脉络
Chat Paper
正在生成论文摘要