Kernel Biclustering algorithm in Hilbert Spaces
arxiv(2022)
摘要
Biclustering algorithms partition data and covariates simultaneously, providing new insights in several domains, such as analyzing gene expression to discover new biological functions. This paper develops a new model-free biclustering algorithm in abstract spaces using the notions of energy distance (ED) and the maximum mean discrepancy (MMD) -- two distances between probability distributions capable of handling complex data such as curves or graphs. The proposed method can learn more general and complex cluster shapes than most existing literature approaches, which usually focus on detecting mean and variance differences. Although the biclustering configurations of our approach are constrained to create disjoint structures at the datum and covariate levels, the results are competitive. Our results are similar to state-of-the-art methods in their optimal scenarios, assuming a proper kernel choice, outperforming them when cluster differences are concentrated in higher-order moments. The model's performance has been tested in several situations that involve simulated and real-world datasets. Finally, new theoretical consistency results are established using some tools of the theory of optimal transport.
更多查看译文
关键词
kernel,algorithm
AI 理解论文
溯源树
样例
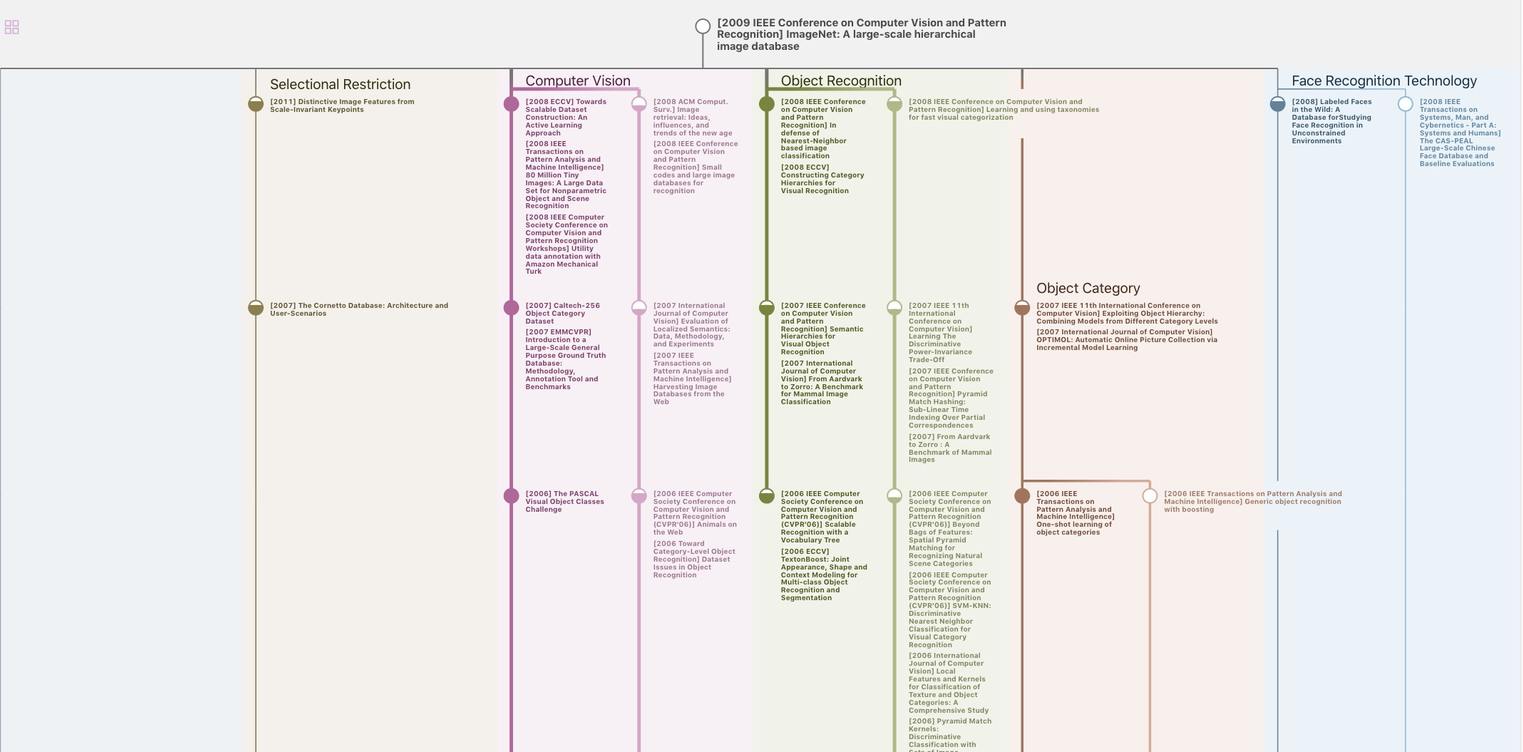
生成溯源树,研究论文发展脉络
Chat Paper
正在生成论文摘要