Compositional Reinforcement Learning for Discrete-Time Stochastic Control Systems
arxiv(2022)
摘要
We propose a compositional approach to synthesize policies for networks of continuous-space stochastic control systems with unknown dynamics using model-free reinforcement learning (RL). The approach is based on implicitly abstracting each subsystem in the network with a finite Markov decision process with unknown transition probabilities, synthesizing a strategy for each abstract model in an assume-guarantee fashion using RL, and then mapping the results back over the original network with approximate optimality guarantees. We provide lower bounds on the satisfaction probability of the overall network based on those over individual subsystems. A key contribution is to leverage the convergence results for adversarial RL (minimax Q-learning) on finite stochastic arenas to provide control strategies maximizing the probability of satisfaction over the network of continuous-space systems. We consider finite-horizon properties expressed in the syntactically co-safe fragment of linear temporal logic. These properties can readily be converted into automata-based reward functions, providing scalar reward signals suitable for RL. Since such reward functions are often sparse, we supply a potential-based reward shaping technique to accelerate learning by producing dense rewards. The effectiveness of the proposed approaches is demonstrated via two physical benchmarks including regulation of a room temperature network and control of a road traffic network.
更多查看译文
关键词
Compositional controller synthesis,minimax-Q learning,reinforcement learning,stochastic control systems
AI 理解论文
溯源树
样例
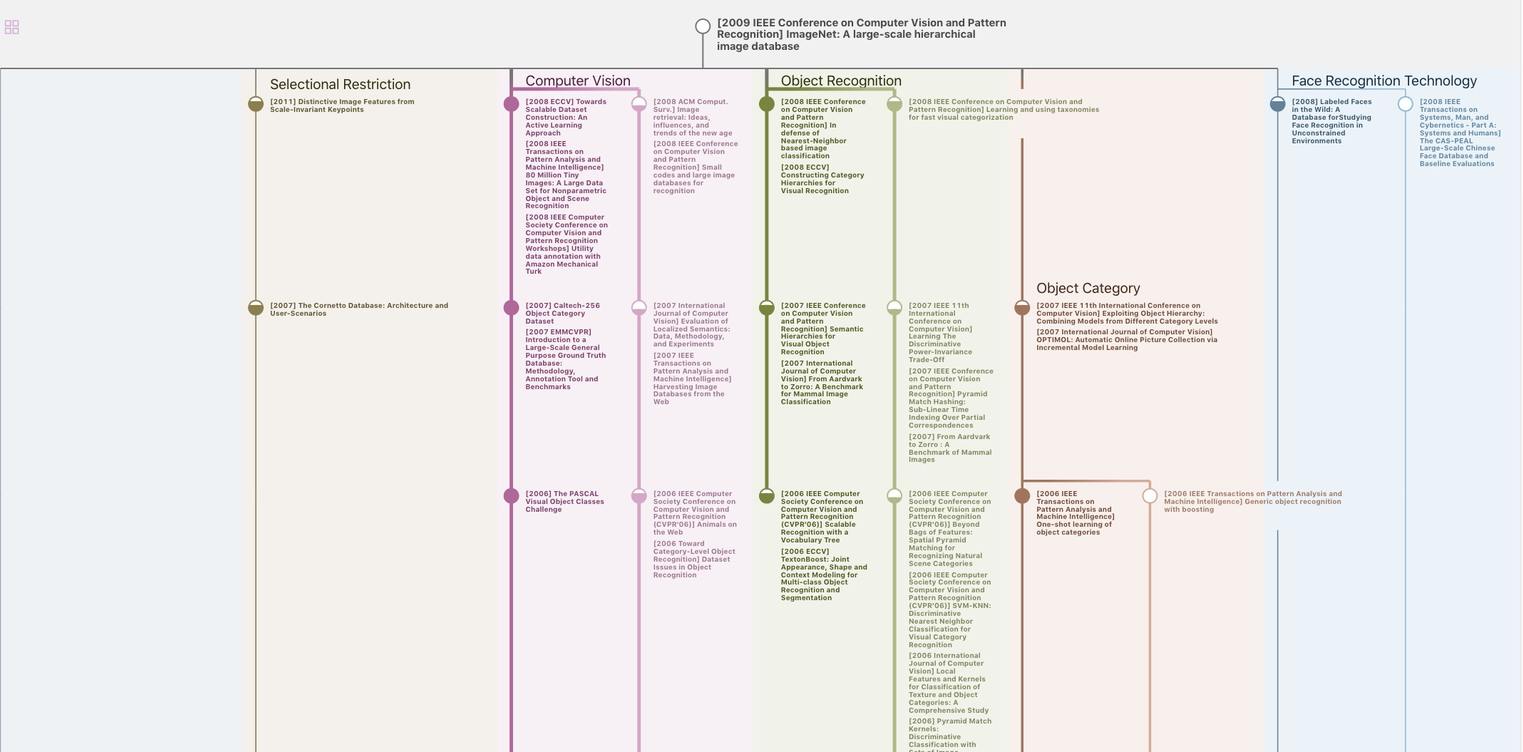
生成溯源树,研究论文发展脉络
Chat Paper
正在生成论文摘要