Bayesian Optimization with Informative Covariance
arxiv(2023)
摘要
Bayesian optimization is a methodology for global optimization of unknown and expensive objectives. It combines a surrogate Bayesian regression model with an acquisition function to decide where to evaluate the objective. Typical regression models are given by Gaussian processes with stationary covariance functions. However, these functions are unable to express prior input-dependent information, including possible locations of the optimum. The ubiquity of stationary models has led to the common practice of exploiting prior information via informative mean functions. In this paper, we highlight that these models can perform poorly, especially in high dimensions. We propose novel informative covariance functions for optimization, leveraging nonstationarity to encode preferences for certain regions of the search space and adaptively promote local exploration during optimization. We demonstrate that the proposed functions can increase the sample efficiency of Bayesian optimization in high dimensions, even under weak prior information.
更多查看译文
关键词
bayesian,covariance,optimization
AI 理解论文
溯源树
样例
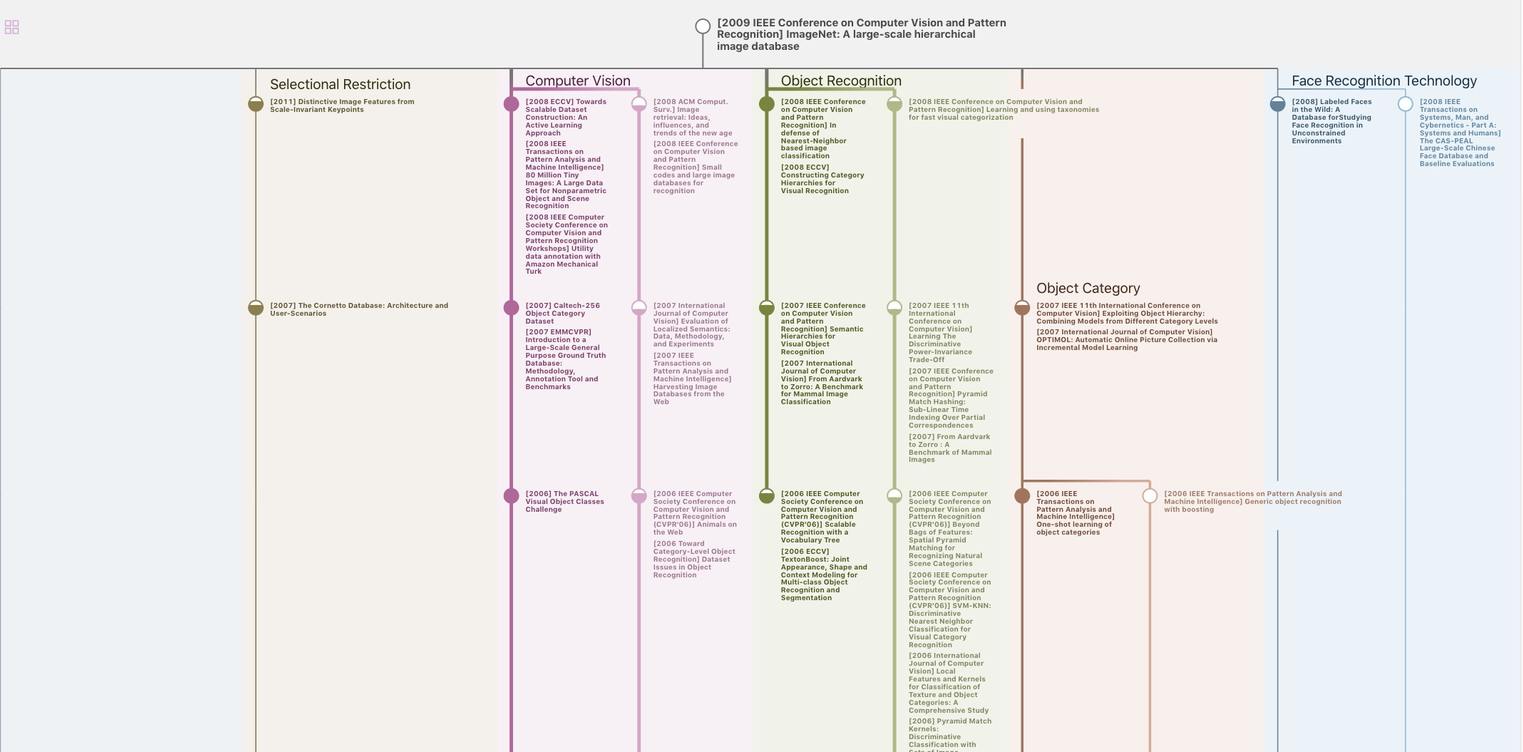
生成溯源树,研究论文发展脉络
Chat Paper
正在生成论文摘要