Image Compression Using Stochastic-AFD Based Multisignal Sparse Representation
IEEE TRANSACTIONS ON IMAGE PROCESSING(2022)
摘要
Adaptive Fourier decomposition (AFD) is a newly developed signal processing tool that can adaptively decompose any single signal using a Szego kernel dictionary. To process multiple signals, a novel stochastic-AFD (SAFD) theory was recently proposed. The innovation of this study is twofold. First, a SAFD-based general multi-signal sparse representation learning algorithm is designed and implemented for the first time in the literature, which can be used in many signal and image processing areas. Second, a novel SAFD based image compression framework is proposed. The algorithm design and implementation of the SAFD theory and image compression methods are presented in detail. The proposed compression methods are compared with 13 other state-of-the-art compression methods, including JPEG, JPEG2000, BPG, and other popular deep learning-based methods. The experimental results show that our methods achieve the best balanced performance. The proposed methods are based on single image adaptive sparse representation learning, and they require no pre-training. In addition, the decompression quality or compression efficiency can be easily adjusted by a single parameter, that is, the decomposition level. Our method is supported by a solid mathematical foundation, which has the potential to become a new core technology in image compression.
更多查看译文
关键词
Image coding,Dictionaries,Signal processing algorithms,Transform coding,Kernel,Training,Discrete cosine transforms,Stochastic adaptive Fourier decomposition,sparse representation,image compression,image reconstruction
AI 理解论文
溯源树
样例
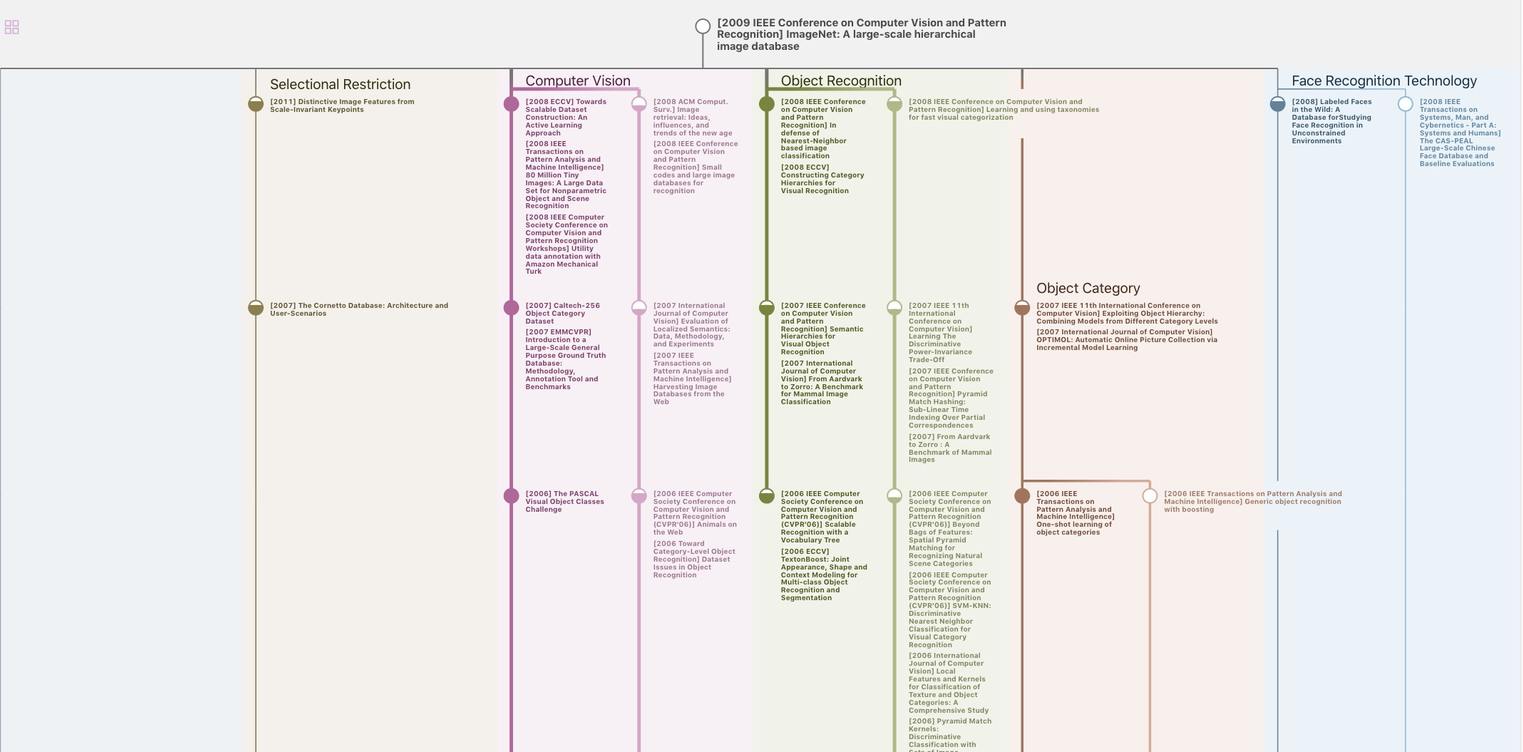
生成溯源树,研究论文发展脉络
Chat Paper
正在生成论文摘要