Bidirectional Deep Variational RNN for Refining Anomaly Detection of Multivariate Time Series
2022 IEEE 10th Joint International Information Technology and Artificial Intelligence Conference (ITAIC)(2022)
摘要
To characterize the complex temporal dependence and stochasticity of multivariate time series (MTS) and achieve their unsupervised anomaly detection, which is an essential task in service quality management of industry devices or Internet infrastructures, in this paper, we propose a bidirectional deep variational RNN (bi-DVRNN), a hierarchical probabilistic dynamical model incorporating bidirectional structures, that can achieve refined anomaly detection at a certain timestep with both past and future information of a specific time frame. Specifically, the core idea of bi-DVRNN is capture the normal patterns of input MTS by collecting transmitted information and long-range temporal dependencies from both directions through deep recurrent structures and hierarchical probabilistic generative operations, and then apply reconstruction probabilities to determine anomalies. For efficient inference of the proposed models, we construct a novel deterministic-upward-stochastic-downward autoencoding inference scheme to achieve an accurate posterior approximation of latent variables. The evaluation experiments conducted on two public datasets show the efficiency of bi-DVRNN in refining anomaly detection results.
更多查看译文
关键词
anomaly detection,bidirectional deep variational RNN,hierarchical prior,multivariate time series
AI 理解论文
溯源树
样例
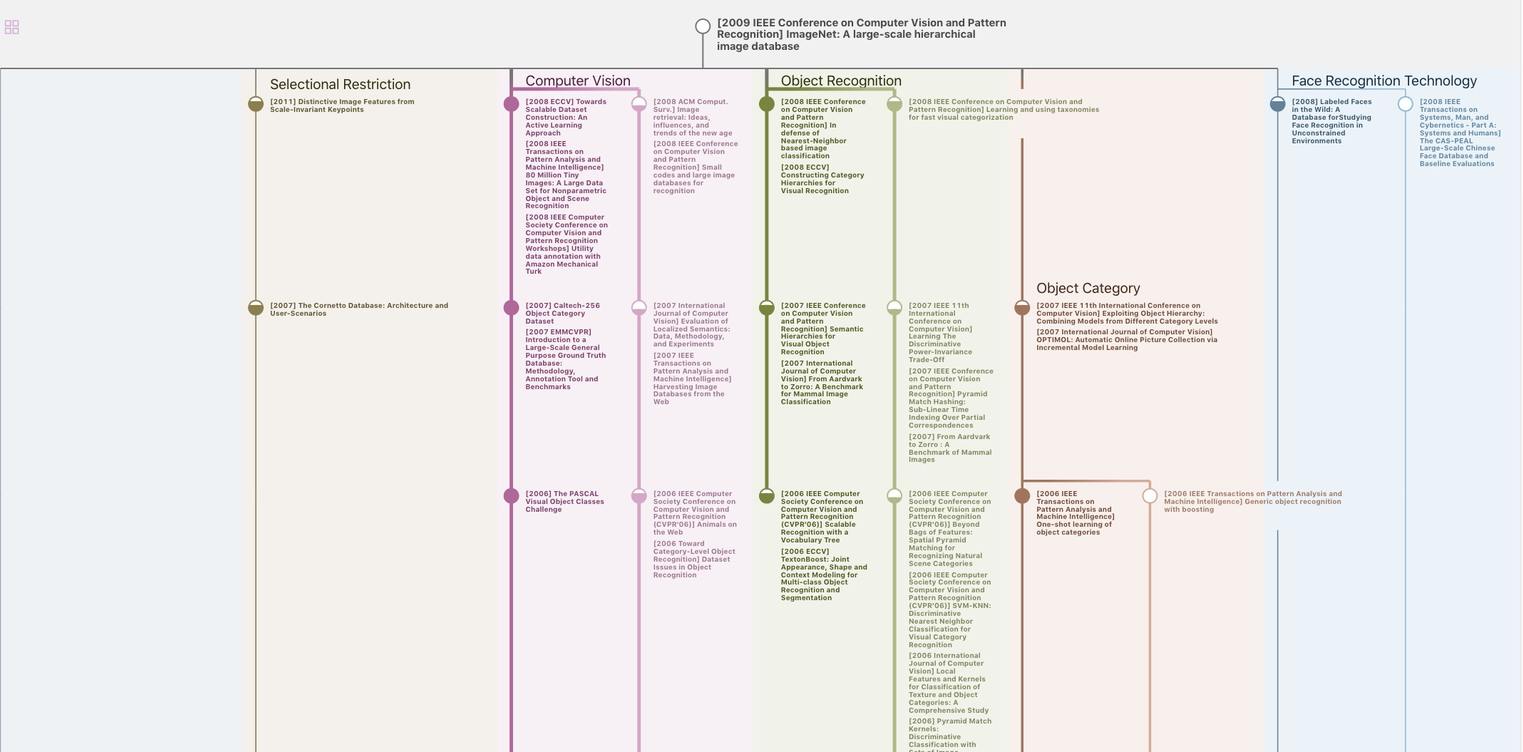
生成溯源树,研究论文发展脉络
Chat Paper
正在生成论文摘要