SGEM: stochastic gradient with energy and momentum
Numerical Algorithms(2023)
摘要
In this paper, we propose SGEM, stochastic gradient with energy and momentum, to solve a class of general non-convex stochastic optimization problems, based on the AEGD method introduced in AEGD (adaptive gradient descent with energy) Liu and Tian (Numerical Algebra, Control and Optimization, 2023 ). SGEM incorporates both energy and momentum so as to inherit their dual advantages. We show that SGEM features an unconditional energy stability property and provide a positive lower threshold for the energy variable. We further derive energy-dependent convergence rates in the general non-convex stochastic setting, as well as a regret bound in the online convex setting. Our experimental results show that SGEM converges faster than AEGD and generalizes better or at least as well as SGDM in training some deep neural networks.
更多查看译文
关键词
Gradient descent,Stochastic optimization,Energy stability,Momentum
AI 理解论文
溯源树
样例
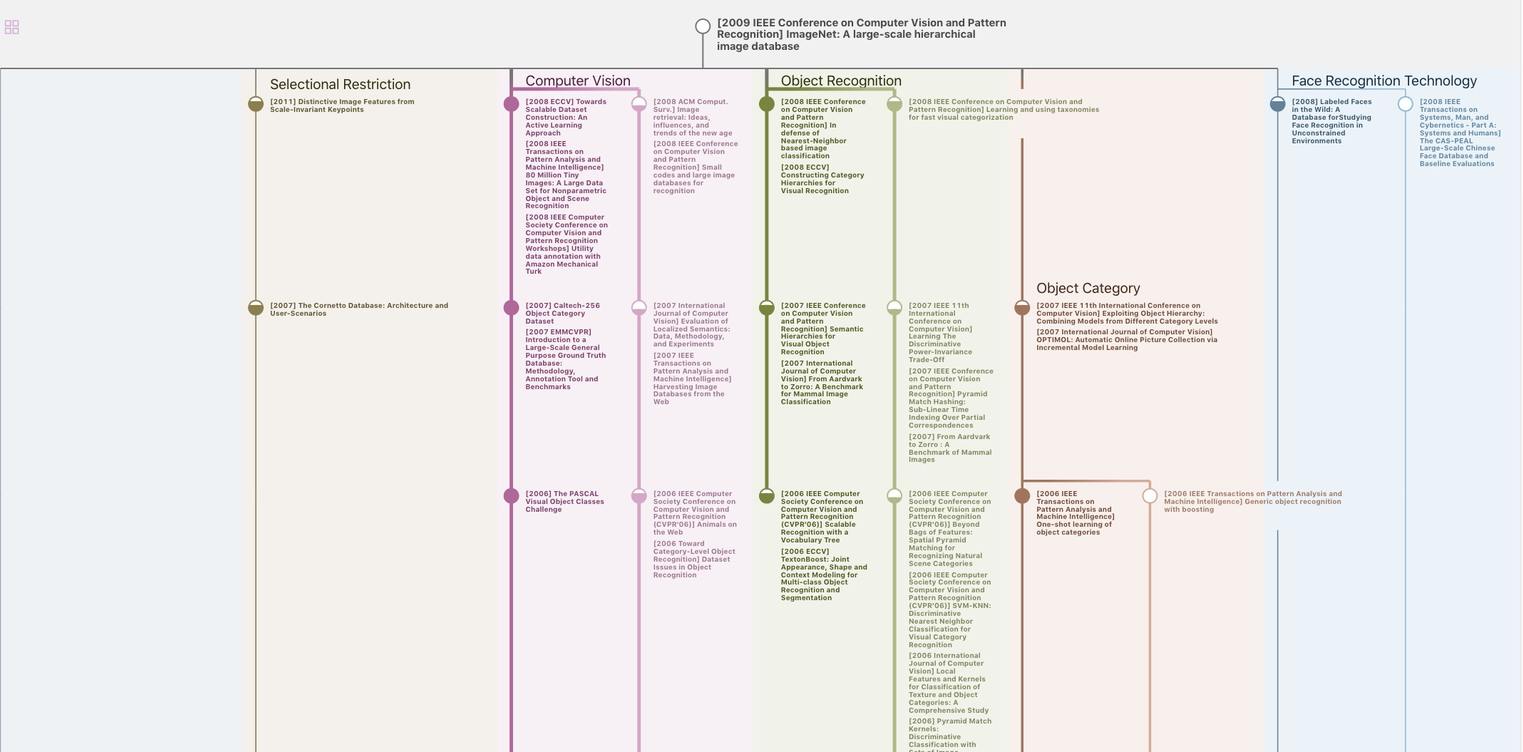
生成溯源树,研究论文发展脉络
Chat Paper
正在生成论文摘要