Dual-Fuzzy-Classifier-Based Evolutionary Algorithm for Expensive Multiobjective Optimization
IEEE TRANSACTIONS ON EVOLUTIONARY COMPUTATION(2023)
摘要
Multiobjective evolutionary algorithms (MOEAs) have been widely used to solve multiobjective optimization problems (MOPs). Conventional MOEAs usually require a large number of function evaluations (FEs) for evaluating the quality of solutions. However, only a limited number of FEs are affordable in many real-world optimization problems, where the FEs are computationally or economically expensive. The use of a large number of FEs decreases the effectiveness of MOEAs in solving these problems. This article proposes a dual-fuzzy-classifier-based surrogate model (DFC) and a DFC-based MOEA (DFC-MOEA) framework for expensive optimization problems. The DFC model is used for offspring preselection to choose high-quality offspring solutions and for categorization to divide unevaluated solutions into three categories. In the proposed framework, two fuzzy classifiers are built to predict the quality of each unevaluated solution. To strike a balance between convergence and diversity, one classifier is designed for predicting the dominance relation of unevaluated solutions (i.e., convergence), and another classifier is designed for predicting the crowdedness of unevaluated solutions (i.e., diversity). The integration of the proposed framework in three different types of MOEAs (i.e., a dominance-based MOEA, an indicator-based MOEA, and a decomposition-based MOEA) demonstrates its usefulness in handling expensive optimization problems. Comprehensive experiments on four well-known test suites and several real-world optimization problems demonstrate that the proposed framework is able to improve the performance of MOEAs under a limited number of FEs.
更多查看译文
关键词
Optimization,Iron,Linear programming,Convergence,Evolutionary computation,Computational modeling,Training,Expensive multiobjective optimization,fuzzy classifiers,multiobjective evolutionary algorithms (MOEAs),surrogate-based evolutionary algorithms
AI 理解论文
溯源树
样例
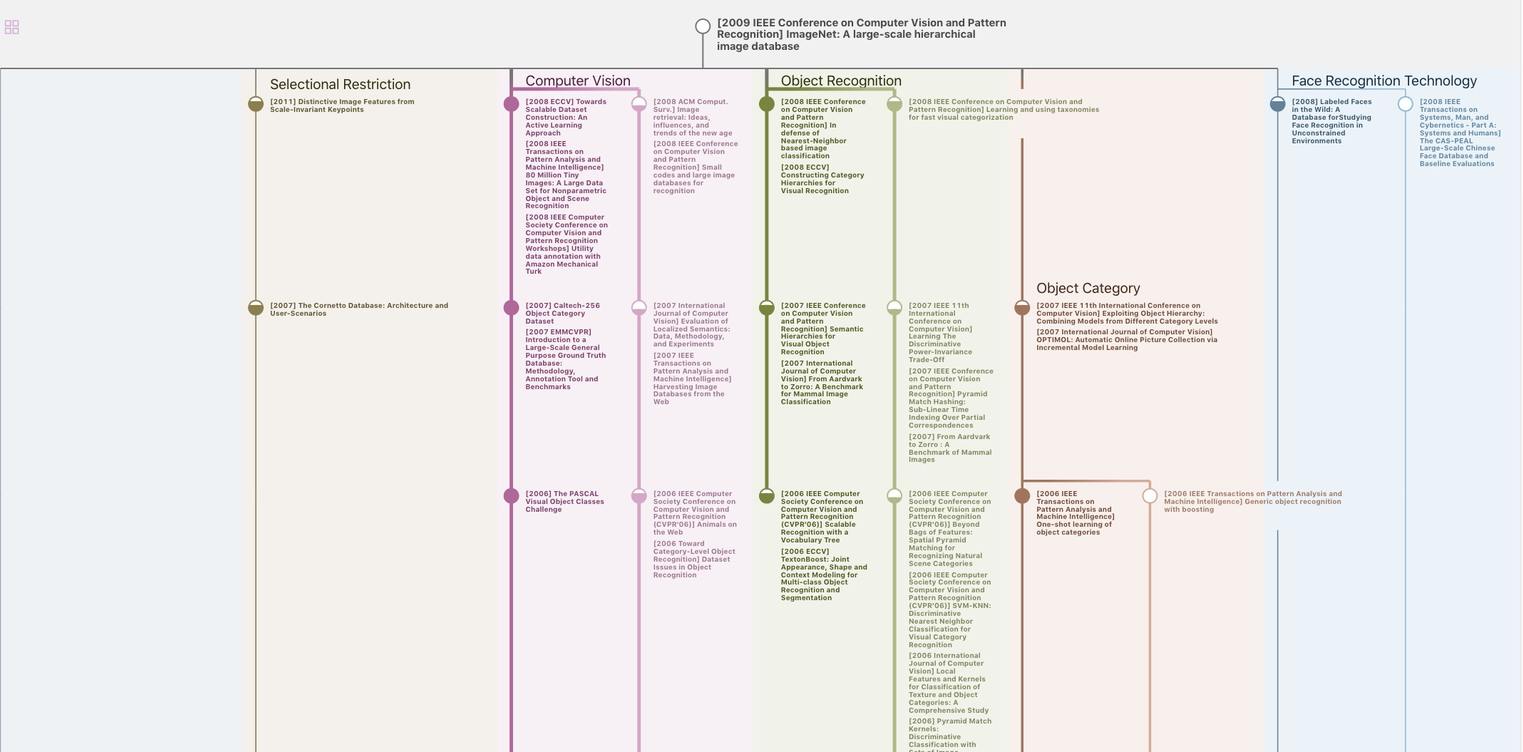
生成溯源树,研究论文发展脉络
Chat Paper
正在生成论文摘要