ELDA: Learning Explicit Dual-Interactions for Healthcare Analytics
2022 IEEE 38th International Conference on Data Engineering (ICDE)(2022)
摘要
Interaction learning plays an essential role in learning patients' comprehensive representations that contribute to improved performance in many analytical tasks. In healthcare, interactions among medical features (i.e., feature-level interactions) can exhibit different abnormal patterns in detail, while interactions among time steps (i.e., time-level interactions) can indicate the dynamic changes in patients' health conditions. Therefore, it is necessary to capture and analyze both types of interactions when conducting healthcare analytics. In this paper, we propose a general framework ELDA that is supported by the novel model ELDA-Net to learn dual interactions for healthcare analytics in an explicit manner. Specifically, we devise a Feature-level Interaction Learning Module that can enrich a separately processed medical feature by learned interactions among medical features, and a Time-level Interaction Learning Module that can enhance the representations of the patients' health conditions by learned interactions among time steps. In both levels, ELDA can provide explicit and intuitive interpretations via explaining through the designed attention mechanism. Further, to facilitate the feature-level interaction learning, we propose a novel Bi-directional Embedding Module in ELDA-Net which can efficiently embed the medical features recorded in numerical values. We evaluate the effectiveness and interpretability of ELDA over two public real-world clinical datasets. The experimental results confirm that ELDA consistently outperforms existing state-of-the-art methods with a significant margin, and supports fine-grained interpretability in both the feature level and the time level with medical insights.
更多查看译文
关键词
healthcare,interaction,interpretability
AI 理解论文
溯源树
样例
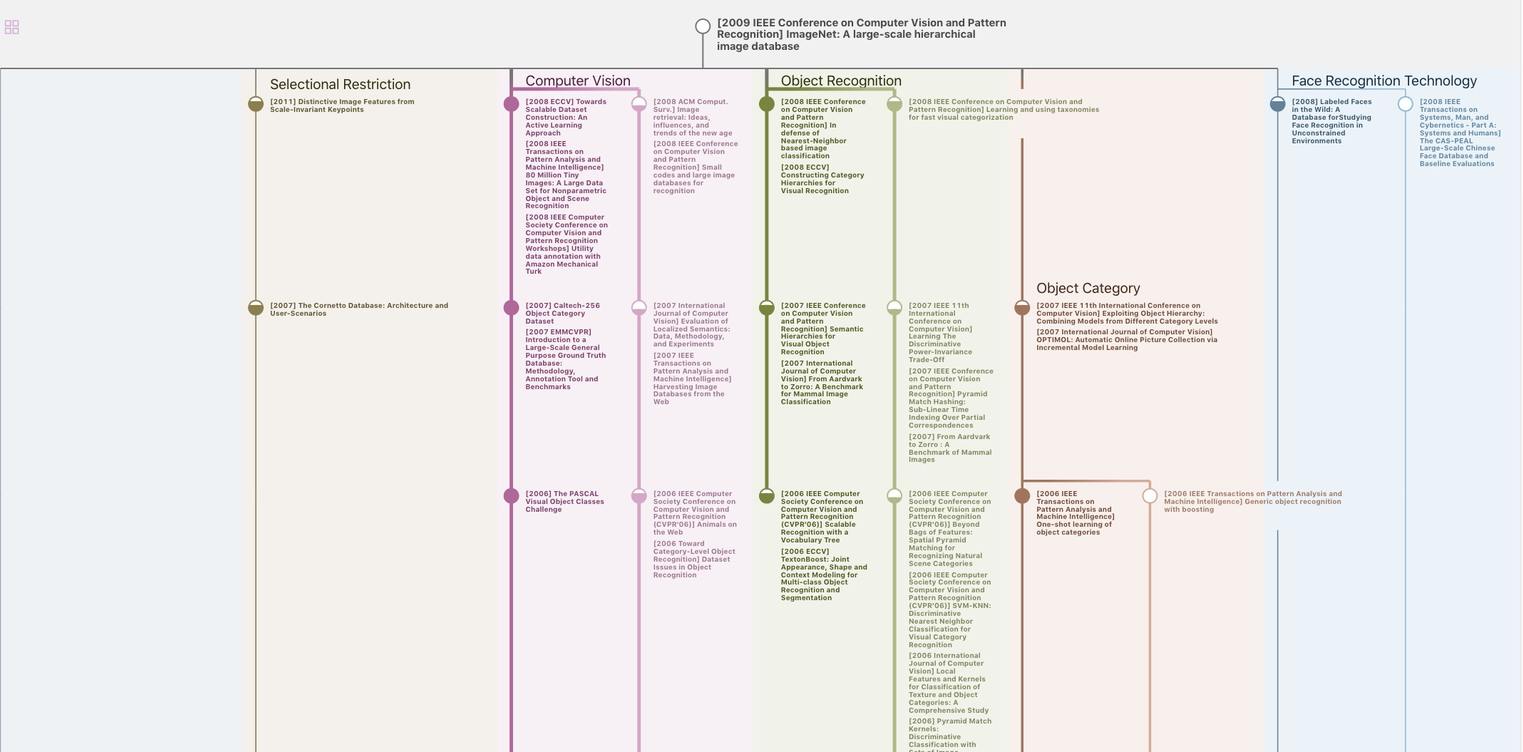
生成溯源树,研究论文发展脉络
Chat Paper
正在生成论文摘要