Fast target-aware learning for few-shot video object segmentation
Science China Information Sciences(2022)
摘要
Few-shot video object segmentation (FSVOS) aims to segment a specific object throughout a video sequence when only the first-frame annotation is given. In this study, we develop a fast target-aware learning approach for FSVOS, where the proposed approach adapts to new video sequences from its first-frame annotation through a lightweight procedure. The proposed network comprises two models. First, the meta knowledge model learns the general semantic features for the input video image and up-samples the coarse predicted mask to the original image size. Second, the target model adapts quickly from the limited support set. Concretely, during the online inference for testing the video, we first employ fast optimization techniques to train a powerful target model by minimizing the segmentation error in the first frame and then use it to predict the subsequent frames. During the offline training, we use a bilevel-optimization strategy to mimic the full testing procedure to train the meta knowledge model across multiple video sequences. The proposed method is trained only on an individual public video object segmentation (VOS) benchmark without additional training sets and compared favorably with state-of-the-art methods on DAVIS-2017, with a J& F overall score of 71.6
更多查看译文
关键词
video object segmentation, few-shot, target-aware, meta knowledge, bilevel-optimization
AI 理解论文
溯源树
样例
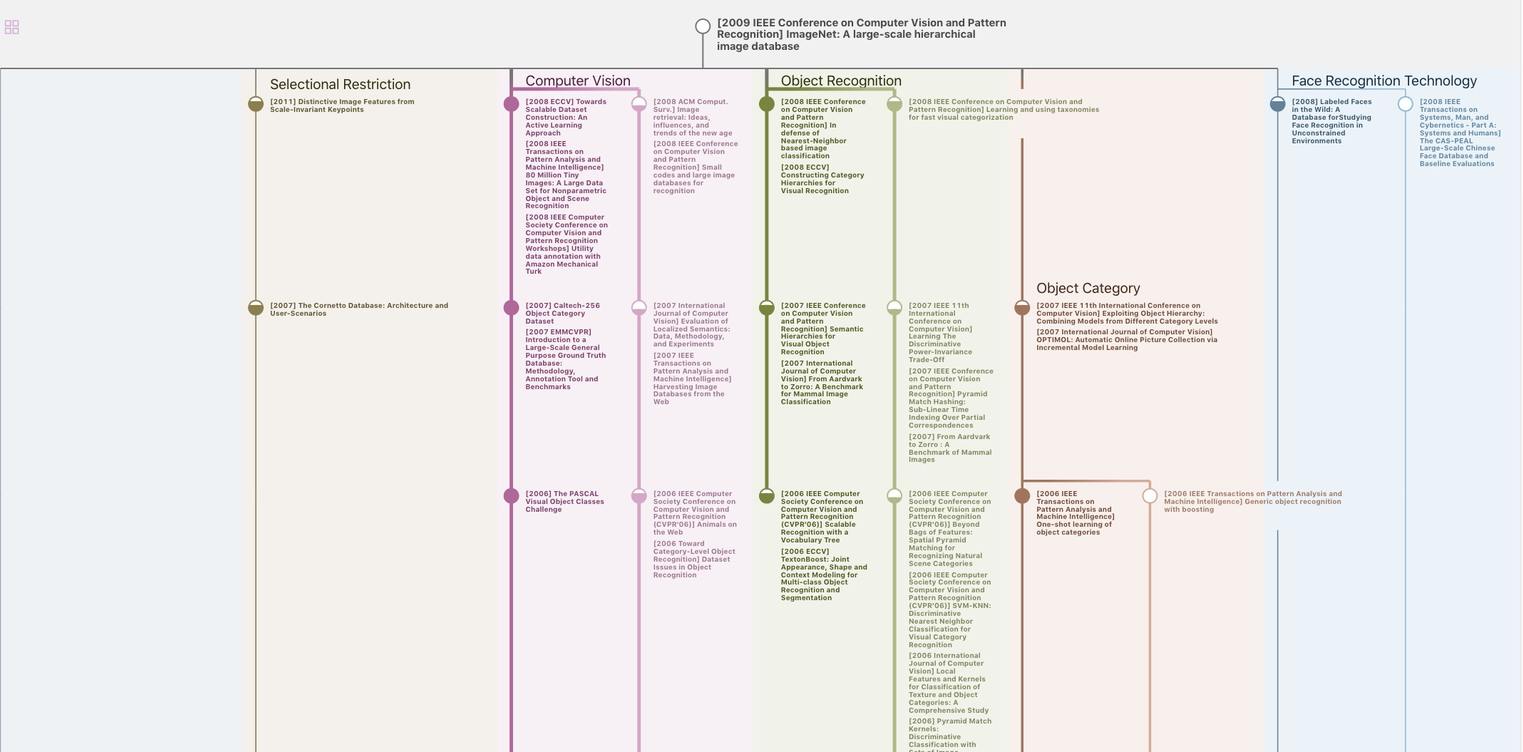
生成溯源树,研究论文发展脉络
Chat Paper
正在生成论文摘要