Analysis of grey-box neural network-based residuals for consistency-based fault diagnosis
IFAC-PapersOnLine(2022)
摘要
Data-driven fault diagnosis requires training data that is representative of the different operating conditions of the system to capture its behavior. If training data is limited, one solution is to incorporate physical insights into machine learning models to improve their effectiveness. However, while previous works show the usefulness of hybrid approaches for isolation of faults, the impact of training data must be taken into consideration when drawing conclusions from data-driven residuals in a consistency-based diagnosis framework. By giving an understanding of the physical interaction between the signals, a hybrid fault diagnosis approach, can enforce model properties of residual generators to isolate faults that are not represented in training data. The objective of this work is to analyze the impact of limited training data when training neural network-based residual generators. It is also investigated how the use of structural information when selecting the network structure is a solution to limited training data and how to ameliorate the performance of hybrid approaches in face of this challenge.
更多查看译文
关键词
Grey-box recurrent neural networks,structural analysis,fault diagnosis,machine learning,model-based diagnosis,anomaly classification
AI 理解论文
溯源树
样例
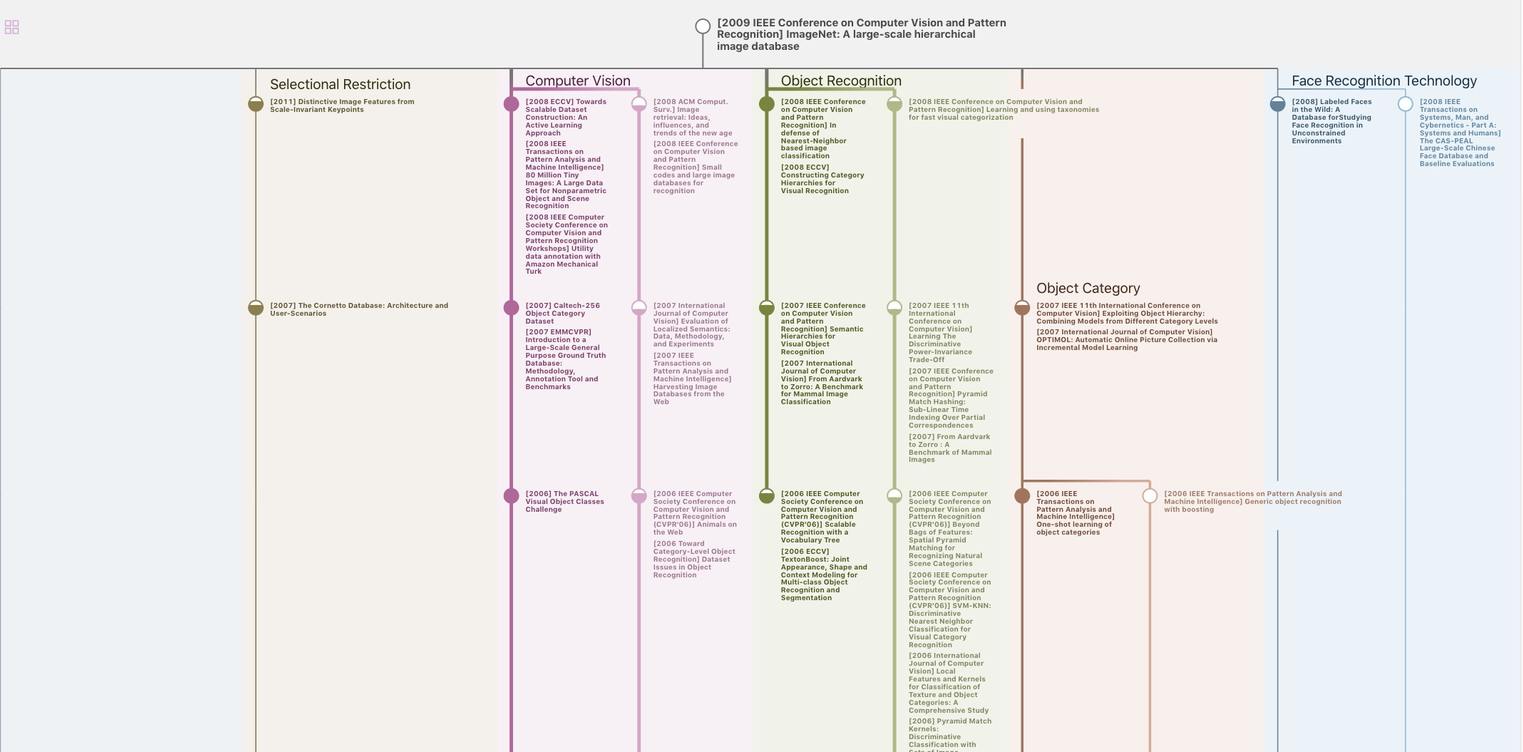
生成溯源树,研究论文发展脉络
Chat Paper
正在生成论文摘要