Infrared Attention Network for Woodland Segmentation Using Multispectral Satellite Images
IEEE TRANSACTIONS ON GEOSCIENCE AND REMOTE SENSING(2022)
摘要
Semantic segmentation of the remote sensing images (RSIs) has attracted increasing interest in recent years. However, large-area segmentation of the woodland presents challenges. The wide distribution and diverse tree species of the woodland make feature extraction difficult. For this reason, an infrared attention network (InfAttNet) is proposed to extract woodland from multispectral RSIs. InfAttNet has an extra infrared spectral encoder which makes use of the sensitivity of vegetation to near-infrared and red edge spectrums. This extra encoder applies learning about vegetation to improve woodland segmentation. Several attention blocks are designed to enhance learning about vegetation features and so improve the performance. In addition, a new dataset is built, containing a large number of woodland RSIs and covering several typical woodland distribution regions in China. The experimental results demonstrate that compared with other networks, InfAttNet has the highest accuracy and is capable of rapid extraction of the woodland in RSIs.
更多查看译文
关键词
Feature extraction,Vegetation mapping,Image segmentation,Task analysis,Semantics,Deep learning,Image edge detection,Attention block,deep learning,infrared spectrums,remote sensing image (RSI),woodland segmentation
AI 理解论文
溯源树
样例
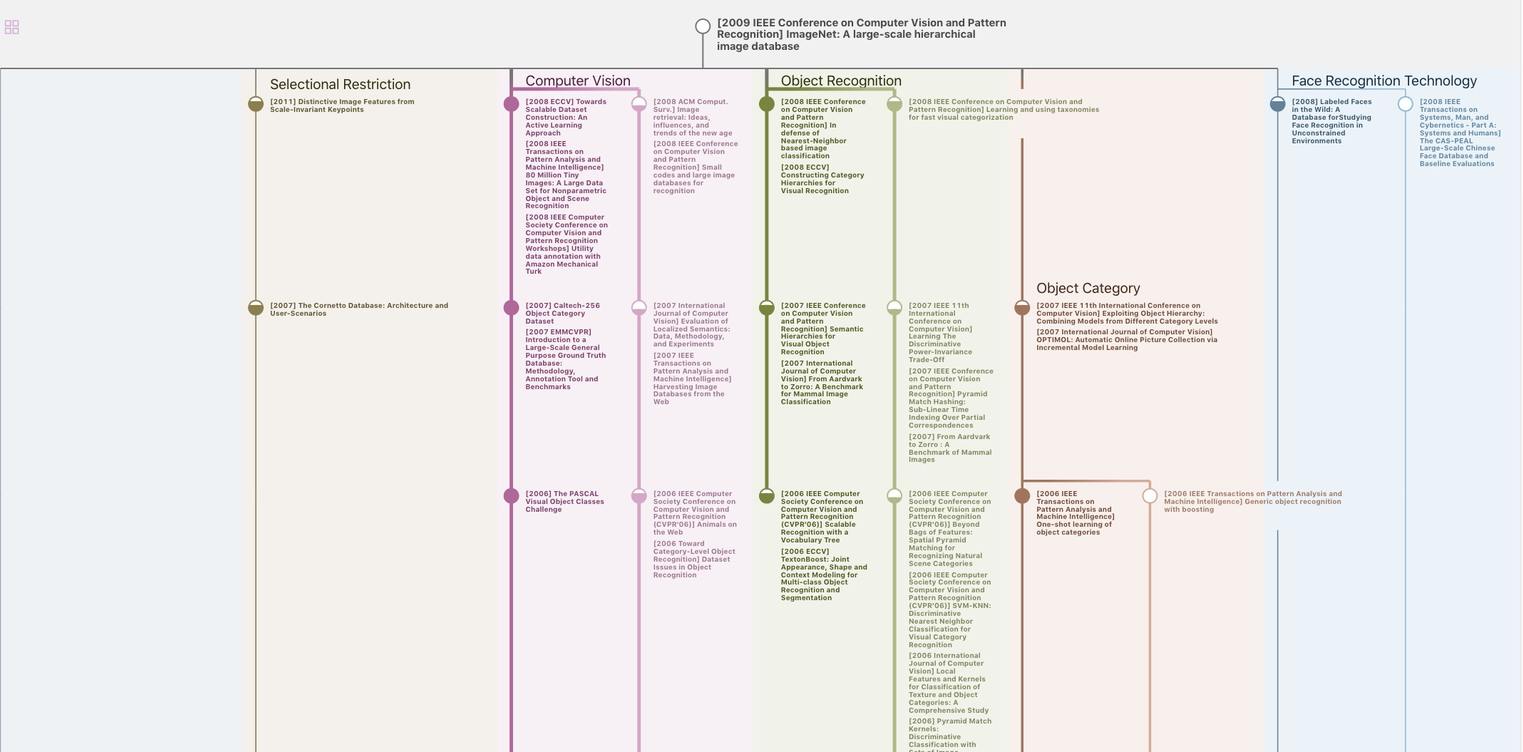
生成溯源树,研究论文发展脉络
Chat Paper
正在生成论文摘要