Data-Efficient Collaborative Decentralized Thermal-Inertial Odometry
IEEE Robotics and Automation Letters(2022)
摘要
We propose a system solution to achieve data-efficient, decentralized state estimation for a team of flying robots using thermal images and inertial measurements. Each robot can fly independently, and exchange data when possible to refine its state estimate. Our system front-end applies an online photometric calibration to refine the thermal images so as to enhance feature tracking and place recognition. Our system back-end uses a covariance-intersection fusion strategy to neglect the cross-correlation between agents so as to lower memory usage and computational cost. The communication pipeline uses Vector of Locally Aggregated Descriptors (VLAD) to construct a request-response policy that requires low bandwidth usage. We test our collaborative method on both synthetic and real-world data. Our results show that the proposed method improves by up to 46% trajectory estimation with respect to an individual-agent approach, while reducing up to 89% the communication exchange. Datasets and code are released to the public, extending the already-public JPL xVIO library.
更多查看译文
关键词
Collaborative localization,drones,space robotics,thermal-inertial odometry
AI 理解论文
溯源树
样例
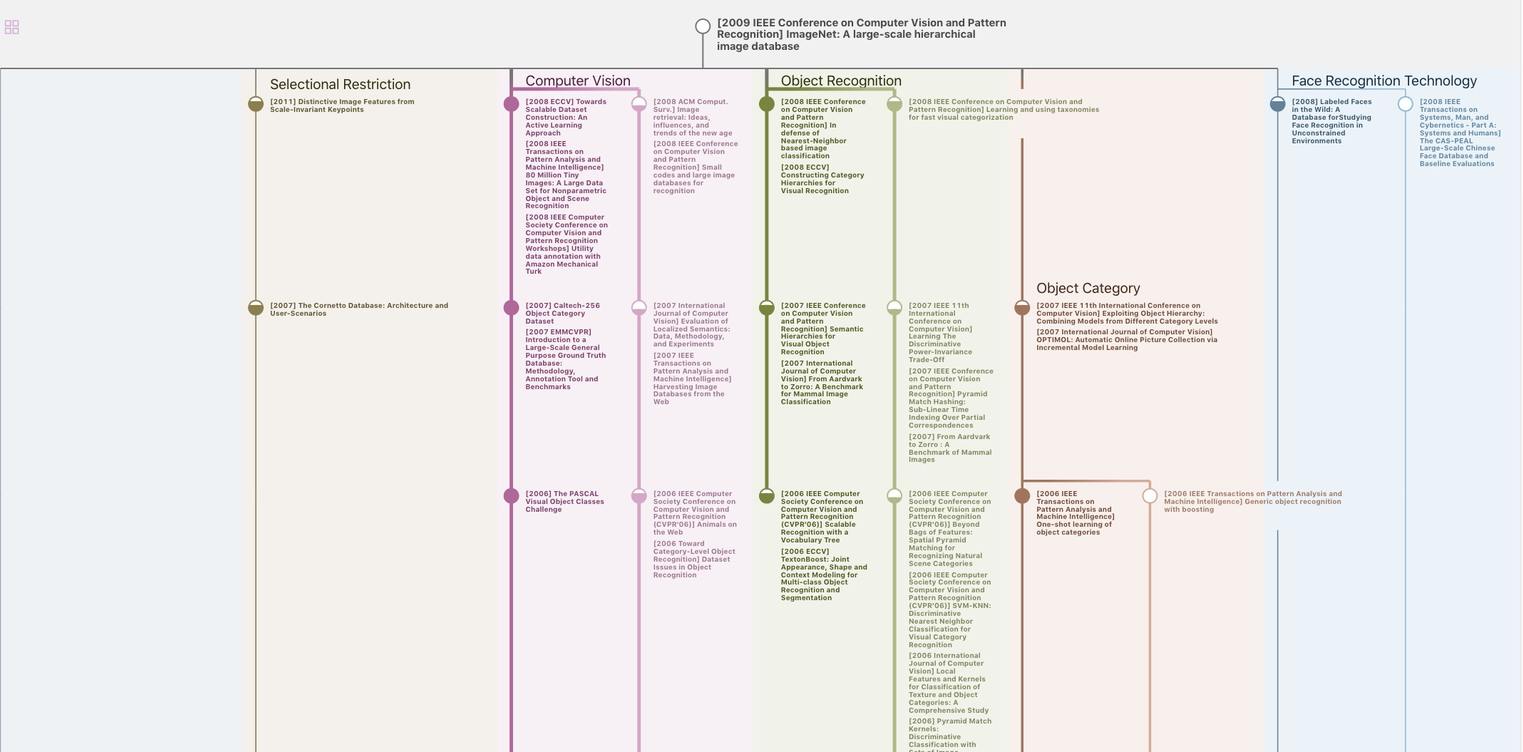
生成溯源树,研究论文发展脉络
Chat Paper
正在生成论文摘要