A Hybrid Scheduling Framework for Mixed Real-Time Tasks in an Automotive System With Vehicular Network
IEEE Transactions on Cloud Computing(2023)
摘要
As vehicles integrate more and more autonomous driving functionalities, it becomes more and more important to use vehicular networks to fully guarantee the safety and real-time performance of on-board computing tasks. Current studies on vehicular networks pay much attention to the performance improvement of network communication and resource allocation, while ignoring the fact that automotive on-board computing tasks play a significant role in vehicle safety and need to be elegantly handled in vehicular networks. In this article, we propose a hybrid scheduling framework for meeting all hard real-time task deadlines while minimizing soft real-time task deadline misses. In particular, the proposed scheduler is composed of some local schedulers and a global scheduler, where the former guarantees the schedulability of all hard real-time tasks, and the latter decides the assignment of soft real-time jobs dynamically online. Depending on the remaining processing capability of a vehicular network, arrival jobs are either assigned for further processing or discarded. An approach combining the utilization-based schedulability test and demand-supply analysis is proposed to effectively assign tasks to processors offline. To meet as many soft real-time task deadlines as possible, tasks’ demand and supply bounds are computed at runtime, such that online execution information can be used to compensate for the scheduling loss with the worst-case assumption made by the offline test. Experimental results demonstrate that, compared to a genetic algorithm, our proposed approach needs far less computation to assign more tasks offline. The online scheduling also saves many soft real-time jobs that were to be dropped by the offline algorithm.
更多查看译文
关键词
Schedule optimization, real-time performance, automotive tasks, vehicular network
AI 理解论文
溯源树
样例
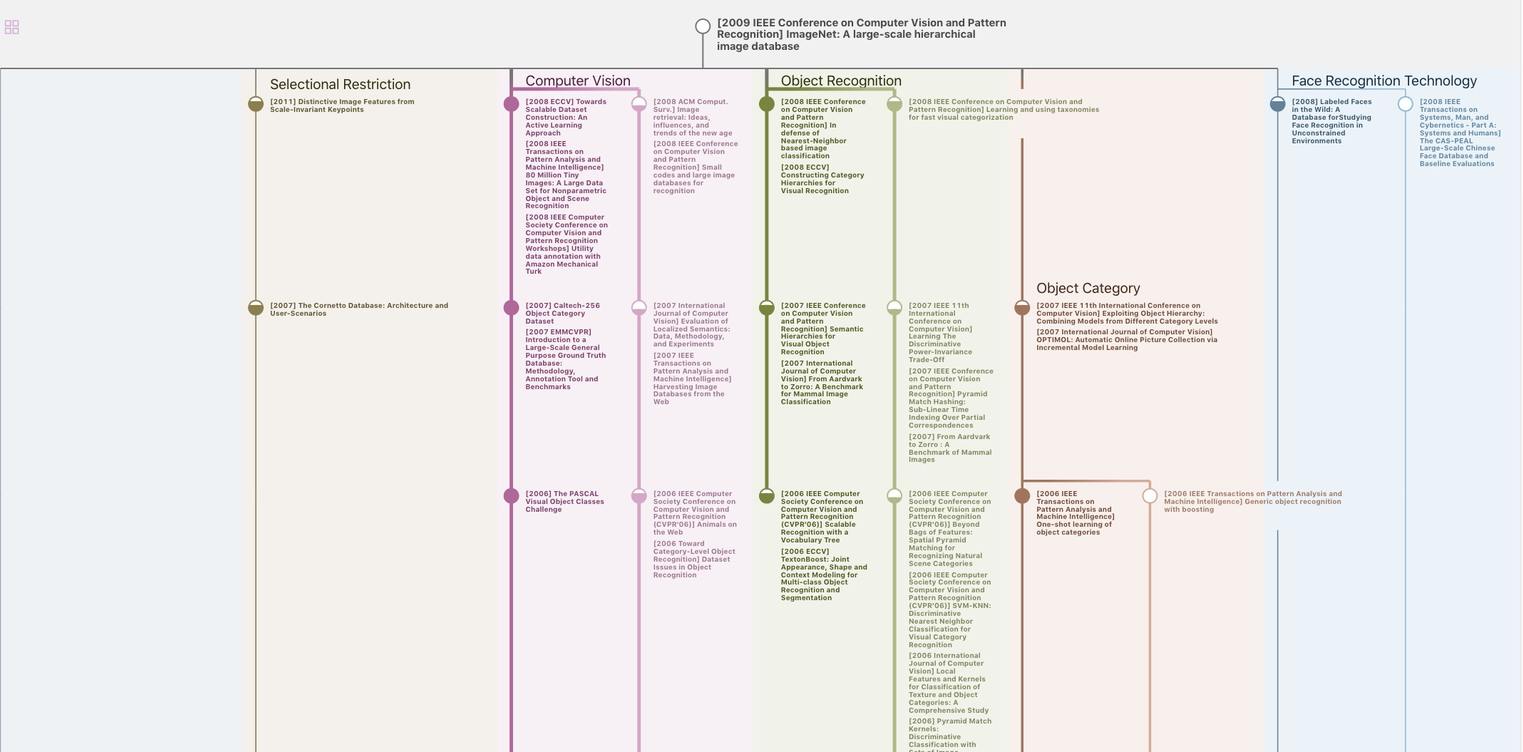
生成溯源树,研究论文发展脉络
Chat Paper
正在生成论文摘要