Ensembling of Efficient Deep Convolutional Networks and Machine Learning Algorithms for Resource Effective Detection of Tuberculosis using Thoracic (Chest) Radiography (June 2022)
IEEE Access(2022)
摘要
Tuberculosis (TB) is a communicable pulmonary disorder and countries with low and middle-income share a higher TB burden as compared to others. The year 2020-2021 universally saw a brutal pandemic in the form of COVID-19, that crushed various lives, health infrastructures, programs, and economies worldwide at an unprecedented speed. The gravity of this estimation gets intensified in systems with limited technological advancements. To assist in the identification of tuberculosis, we propose the ensembling of efficient deep convolutional networks and machine learning algorithms that do not entail heavy computational resources. In this paper, the three of the most efficient deep convolutional networks and machine learning algorithms are employed for resource-effective (low computational and basic Imaging requirements) detection of Tuberculosis. The pivotal features extracted from the deep networks are ensembled and subsequently, the machine learning algorithms are used to identify the images based on the extracted features. The said model underwent k-fold cross-validation and achieved an accuracy of 87.90% and 99.10% with an AUC of 0.94 and 1 respectively in identifying TB infected images from Normal and COVID infected images. Also, the model's error rate, F-score, and youden's index values of 0.0093, 0.9901, and 0.9812 for TB versus COVID identification along with the model's accuracy claim that its use can be beneficial in identifying TB infections amid this COVID-19 pandemic, predominantly in countries with limited resources.
更多查看译文
关键词
Feature extraction, Lung, COVID-19, Machine learning algorithms, Diseases, Radiography, X-ray imaging, COVID-19, chest X-ray, deep convolutional networks, ensemble learning, machine learning, tuberculosis
AI 理解论文
溯源树
样例
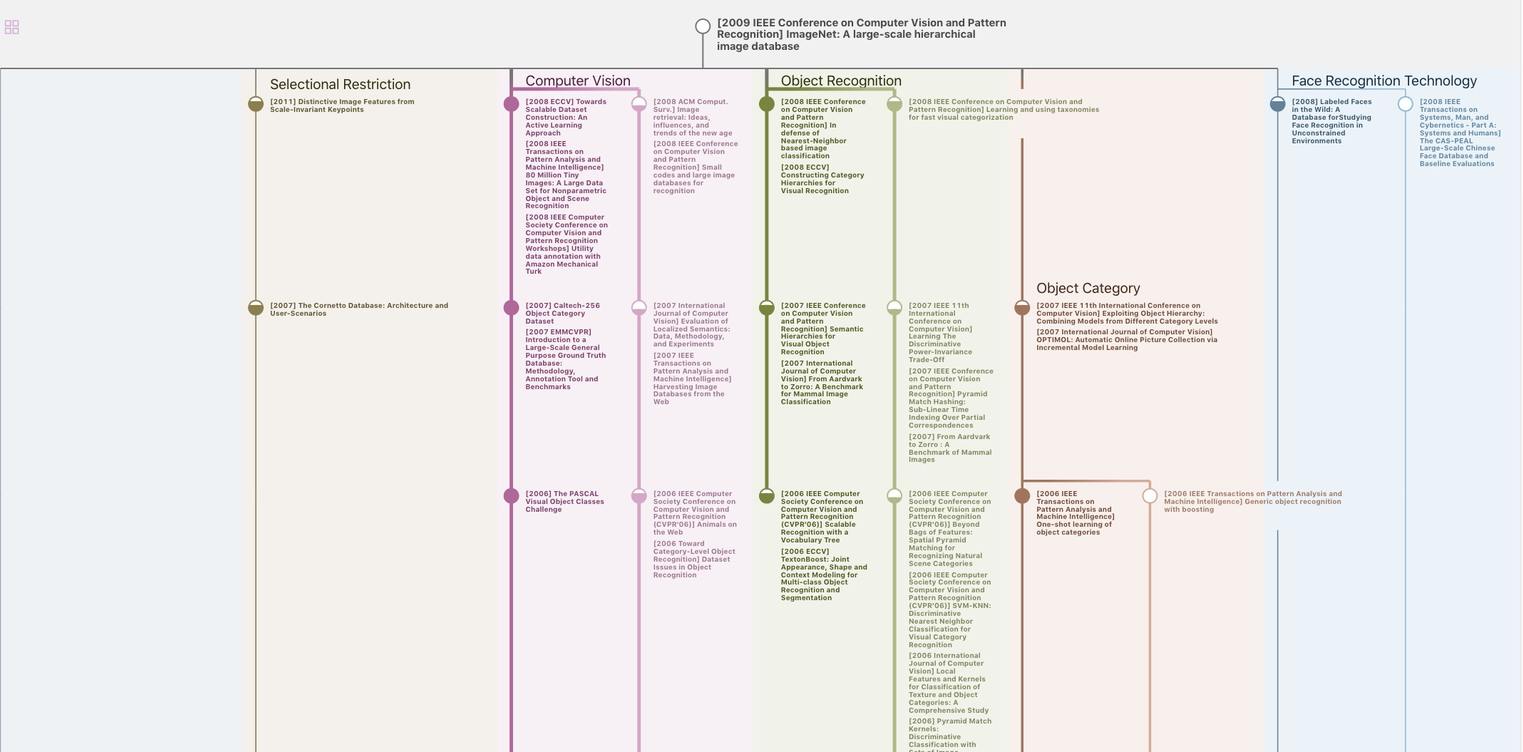
生成溯源树,研究论文发展脉络
Chat Paper
正在生成论文摘要