Self-Supervised Scene-Debiasing for Video Representation Learning via Background Patching
IEEE Transactions on Multimedia(2023)
摘要
Self-supervised learning has considerably improved video representation learning by discovering supervisory signals automatically from unlabeled videos. However, due to the scene-biased nature of existing video datasets, the current methods are biased to the dominant scene context during action inference. Hence, this paper proposes Background Patching (BP), a scene-debiasing augmentation strategy to alleviate the model reliance on the video background in a self-supervised contrastive manner. The BP reduces the negative influence of the video background by mixing a randomly patched frame to the video background. BP randomly crops four frames from four different videos and patches them to construct a new frame for each video separately. The patched frame is mixed with all frames of the target video to produce a spatially distorted video sample. Then, we use existing self-supervised contrastive frameworks to pull representations of the distorted and original videos closer together. Moreover, BP mixes the semantic labels of patches with the target video's label, resulting in the regularization of the contrastive model to soften the decision boundaries in the embedding space. Therefore, the model is explicitly constrained to suppress the background influence by emphasizing more on the motion changes. The extensive experimental results show that our BP significantly improved the performance of various video understanding downstream tasks including action recognition, action detection, and video retrieval.
更多查看译文
关键词
video representation learning,background patching,self-supervised,scene-debiasing
AI 理解论文
溯源树
样例
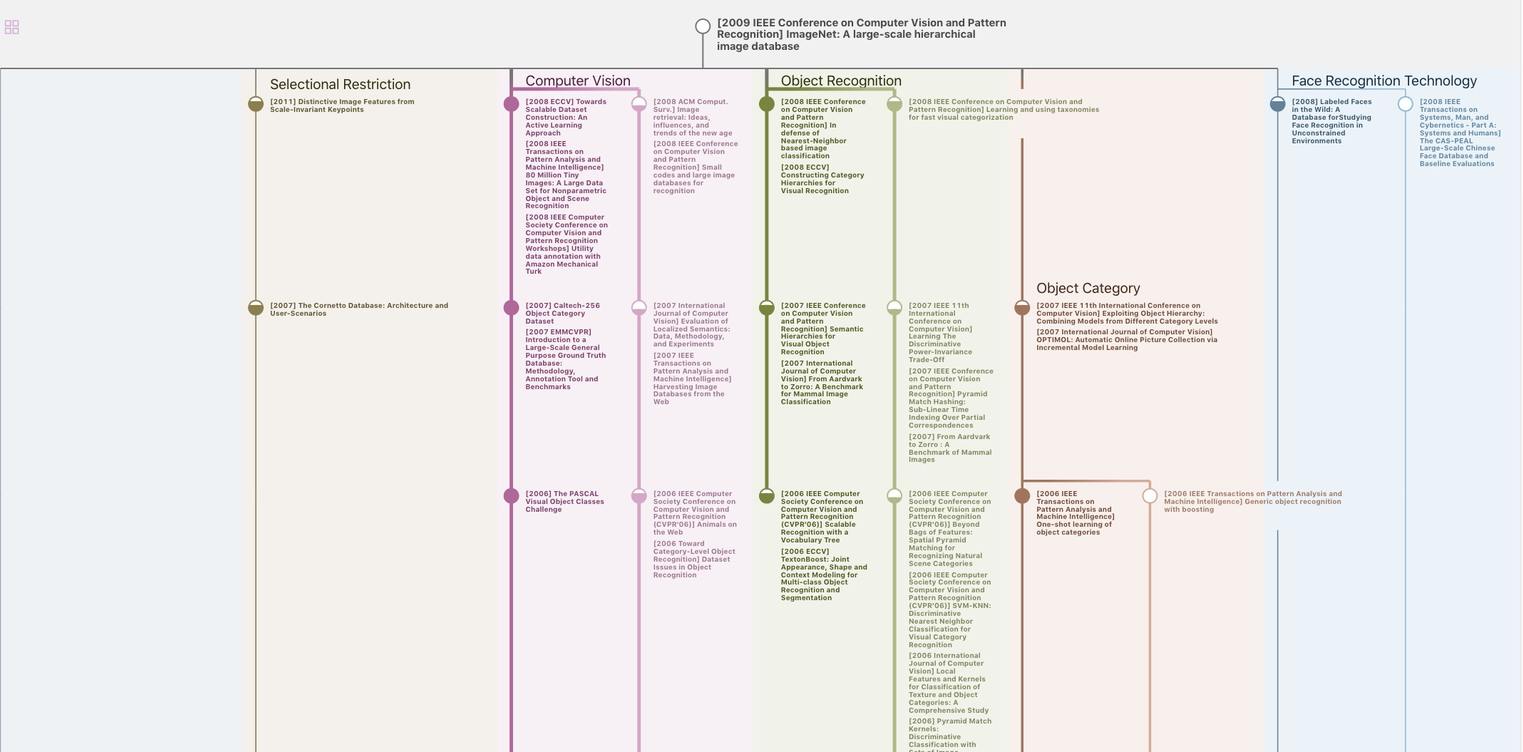
生成溯源树,研究论文发展脉络
Chat Paper
正在生成论文摘要