Equivariance and Invariance Inductive Bias for Learning from Insufficient Data.
European Conference on Computer Vision(2022)
摘要
We are interested in learning robust models from insufficient data, without the need for any externally pre-trained checkpoints. First, compared to sufficient data, we show why insufficient data renders the model more easily biased to the limited training environments that are usually different from testing. For example, if all the training swan samples are “white”, the model may wrongly use the “white” environment to represent the intrinsic class swan. Then, we justify that equivariance inductive bias can retain the class feature while invariance inductive bias can remove the environmental feature, leaving the class feature that generalizes to any environmental changes in testing. To impose them on learning, for equivariance, we demonstrate that any off-the-shelf contrastive-based self-supervised feature learning method can be deployed; for invariance, we propose a class-wise invariant risk minimization (IRM) that efficiently tackles the challenge of missing environmental annotation in conventional IRM. State-of-the-art experimental results on real-world benchmarks (VIPriors, ImageNet100 and NICO) validate the great potential of equivariance and invariance in data-efficient learning. The code is available at https://github.com/Wangt-CN/EqInv.
更多查看译文
关键词
Inductive bias,Equivariance,Invariant risk minimization
AI 理解论文
溯源树
样例
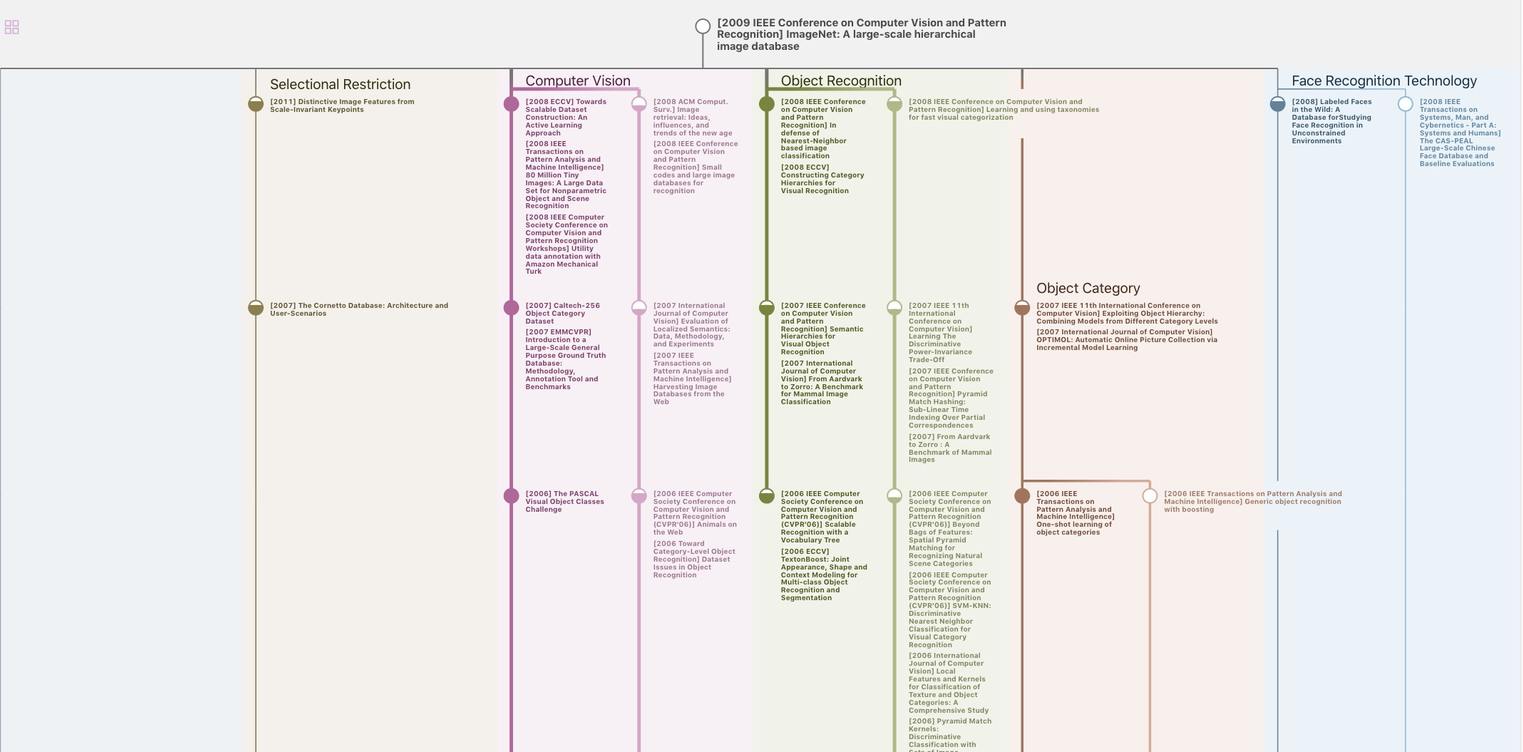
生成溯源树,研究论文发展脉络
Chat Paper
正在生成论文摘要