An Adaptive Superfast Inexact Proximal Augmented Lagrangian Method for Smooth Nonconvex Composite Optimization Problems
arxiv(2023)
摘要
This work presents an adaptive superfast proximal augmented Lagrangian (AS-PAL) method for solving linearly-constrained smooth nonconvex composite optimization problems. Each iteration of AS-PAL inexactly solves a possibly nonconvex proximal augmented Lagrangian (AL) subproblem obtained by an aggressive/adaptive choice of prox stepsize with the aim of substantially improving its computational performance followed by a full Lagrange multiplier update. A major advantage of AS-PAL compared to other AL methods is that it requires no knowledge of parameters (e.g., size of constraint matrix, objective function curvatures, etc) associated with the optimization problem, due to its adaptive nature not only in choosing the prox stepsize but also in using a crucial adaptive accelerated composite gradient variant to solve the proximal AL subproblems. The speed and efficiency of AS-PAL is demonstrated through extensive computational experiments showing that it can solve many instances more than ten times faster than other state-of-the-art penalty and AL methods, particularly when high accuracy is required.
更多查看译文
关键词
First-order accelerated method, Augmented Lagrangian method, Smooth weakly convex function, Linearly-constrained nonconvex composite optimization, Iteration complexity, Adaptive method, 90C26, 90C30, 65K10, 90C60, 47J22
AI 理解论文
溯源树
样例
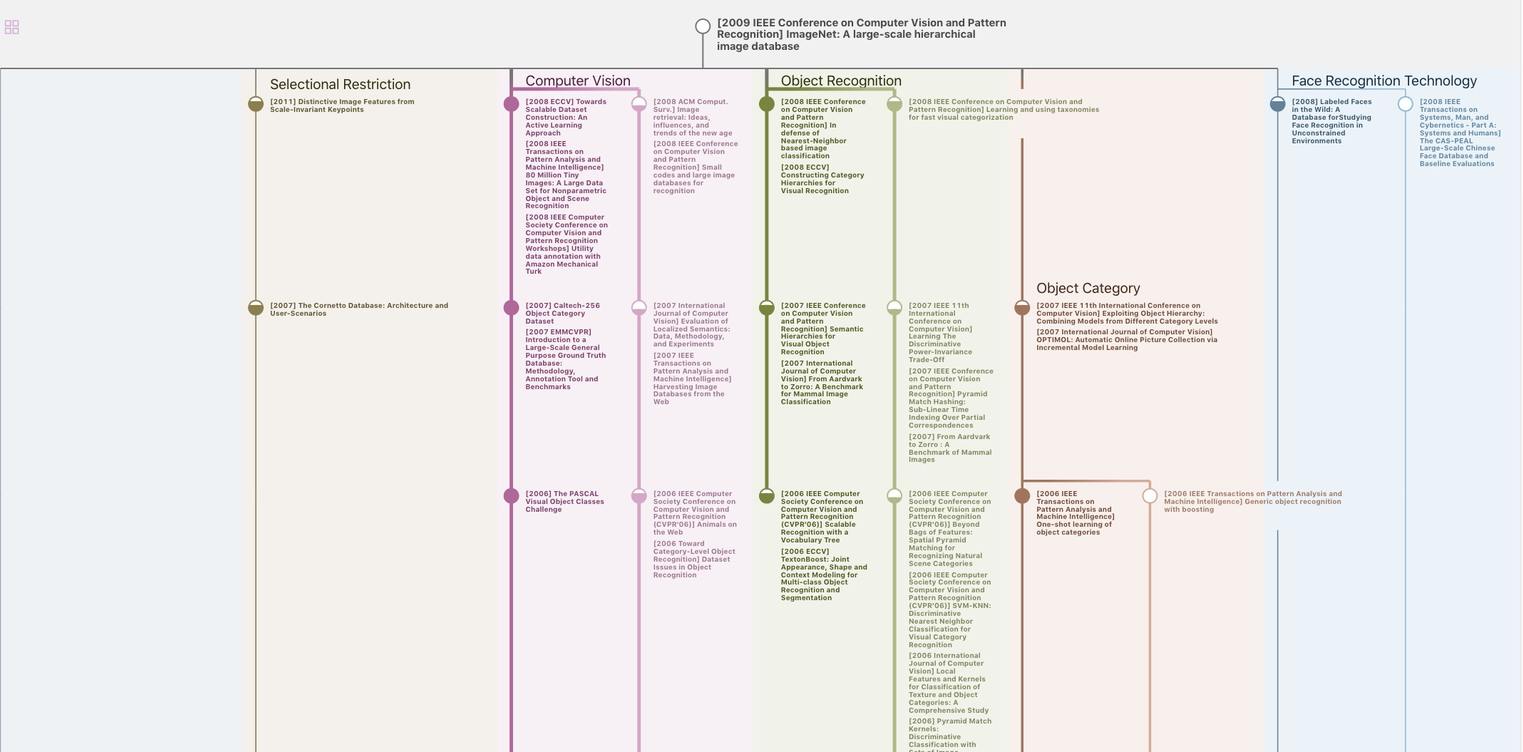
生成溯源树,研究论文发展脉络
Chat Paper
正在生成论文摘要