Practical perspectives on symplectic accelerated optimization
arxiv(2023)
摘要
Geometric numerical integration has recently been exploited to design symplectic accelerated optimization algorithms by simulating the Bregman Lagrangian and Hamiltonian systems from the variational framework introduced by Wibisono et al. In this paper, we discuss practical considerations which can significantly boost the computational performance of these optimization algorithms and considerably simplify the tuning process. In particular, we investigate how momentum restarting schemes ameliorate computational efficiency and robustness by reducing the undesirable effect of oscillations and ease the tuning process by making time-adaptivity superfluous. We also discuss how temporal looping helps avoiding instability issues caused by numerical precision, without harming the computational efficiency of the algorithms. Finally, we compare the efficiency and robustness of different geometric integration techniques and study the effects of the different parameters in the algorithms to inform and simplify tuning in practice. From this paper emerge symplectic accelerated optimization algorithms whose computational efficiency, stability and robustness have been improved, and which are now much simpler to use and tune for practical applications.
更多查看译文
关键词
Accelerated optimization,Lagrangian dynamics,Hamiltonian dynamics,Bregman dynamics,symplectic integrators,variational integrators,geometric numerical integration
AI 理解论文
溯源树
样例
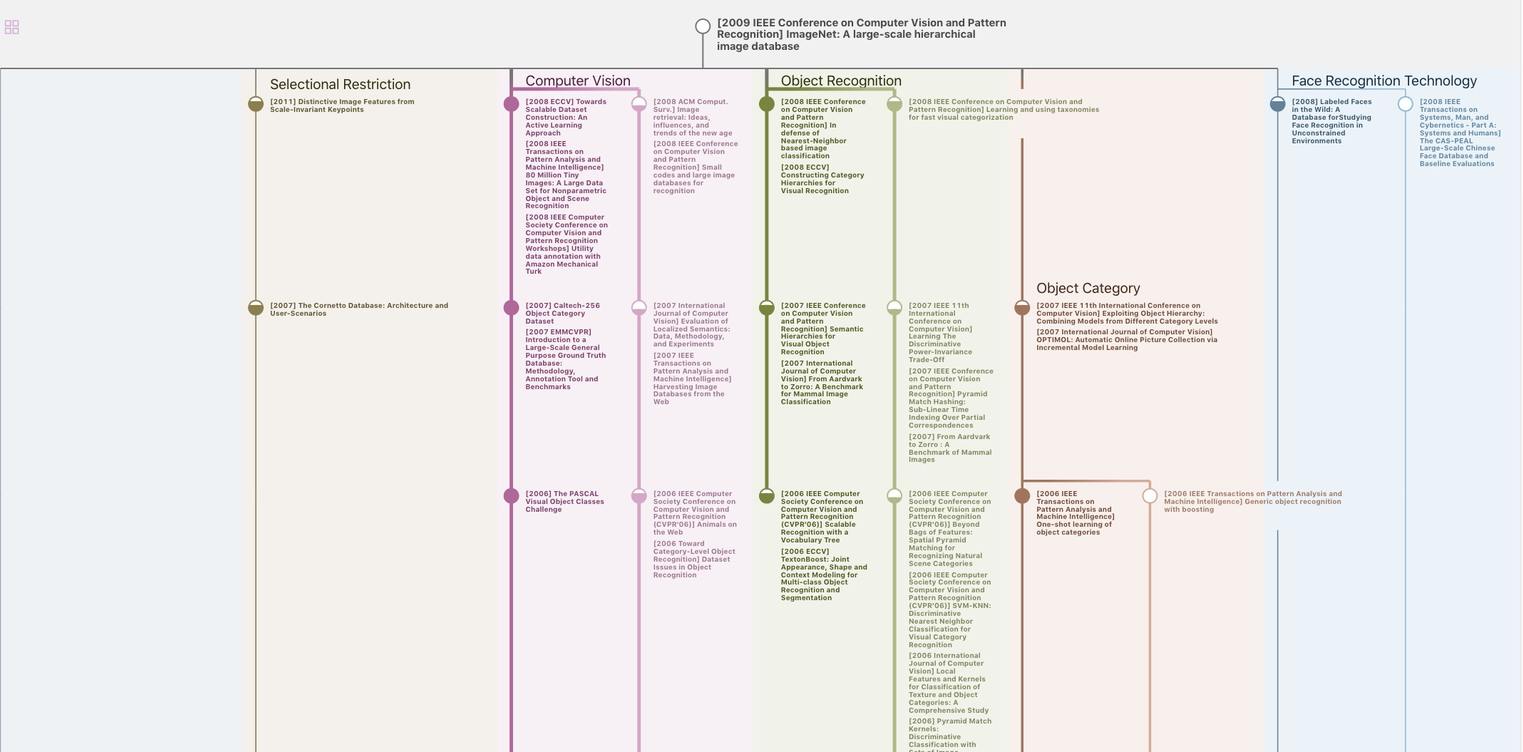
生成溯源树,研究论文发展脉络
Chat Paper
正在生成论文摘要