Few-shot Node Classification on Attributed Networks with Graph Meta-learning
SIGIR '22: Proceedings of the 45th International ACM SIGIR Conference on Research and Development in Information Retrieval(2022)
摘要
Attributed networks, as a manifestation of data in non-Euclidean domains, have a wide range of applications in the real world, such as molecular property prediction, social network analysis and anomaly detection. Node classification, as a fundamental research problem in attributed networks, has attracted increasing attention among research communities. However, most existing models cannot be directly applied to the data with limited labeled instances (\textiti.e., the few-shot scenario). Few-shot node classification on attributed networks is gradually becoming a research hotspot. Although several methods aim to integrate meta-learning with graph neural networks to address this problem, some limitations remain. First, they all assume node representation learning using graph neural networks in homophilic graphs. %Hence, suboptimal performance is obtained when these models are applied to heterophilic graphs. Second, existing models based on meta-learning entirely depend on instance-based statistics. %which in few-shot settings are unavoidably degraded by data noise or outliers. Third, most previous models treat all sampled tasks equally and fail to adapt their uniqueness. %which has a significant impact on the overall performance of the model. To solve the above three limitations, we propose a novel graph Meta -learning framework called G raph learning based on P rototype and S caling & shifting transformation (Meta-GPS ). More specifically, we introduce an efficient method for learning expressive node representations even on heterophilic graphs and propose utilizing a prototype-based approach to initialize parameters in meta-learning. Moreover, we also leverage S$^2$ (scaling & shifting) transformation to learn effective transferable knowledge from diverse tasks. Extensive experimental results on six real-world datasets demonstrate the superiority of our proposed framework, which outperforms other state-of-the-art baselines by up to 13% absolute improvement in terms of related metrics.
更多查看译文
关键词
graph neural networks, few-shot learning, node classification
AI 理解论文
溯源树
样例
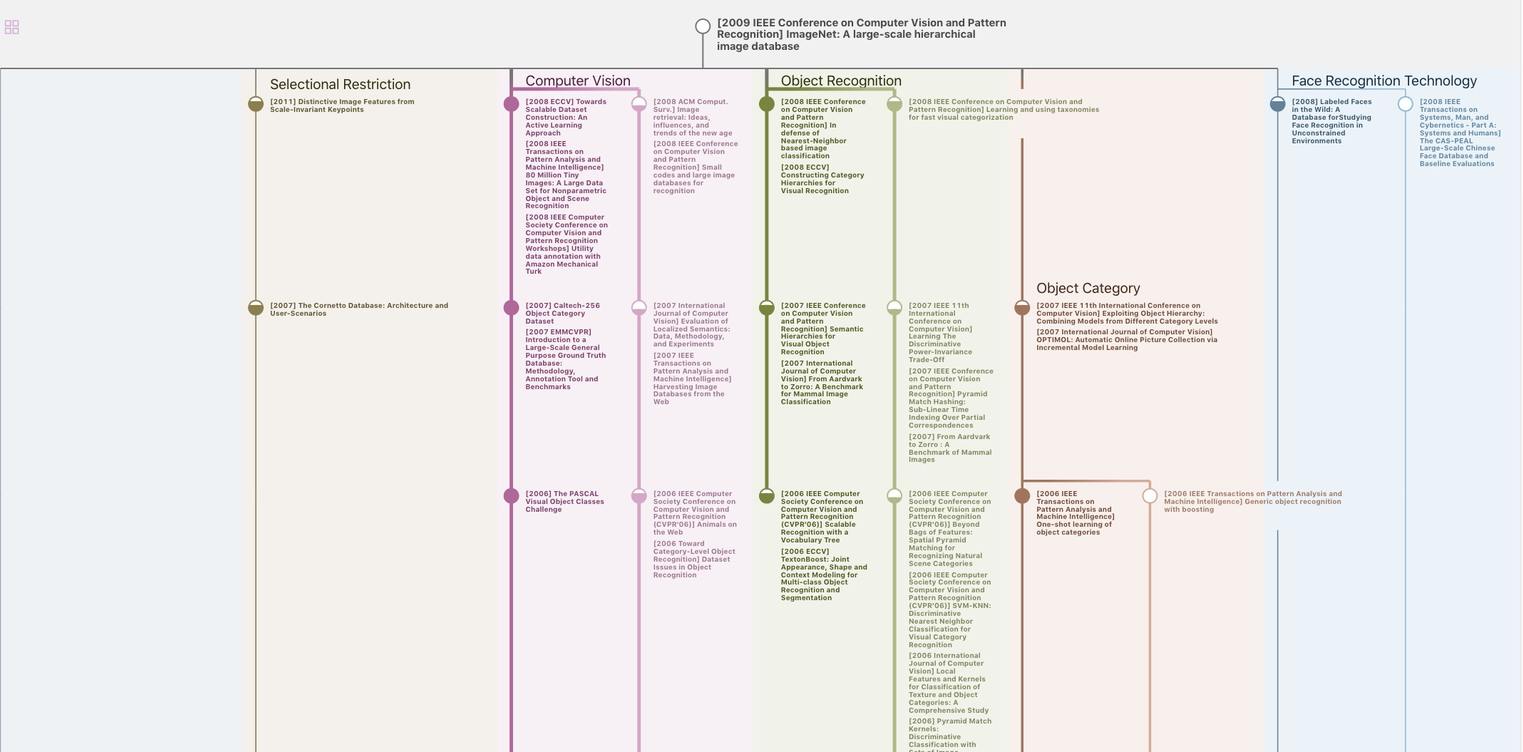
生成溯源树,研究论文发展脉络
Chat Paper
正在生成论文摘要