Scale dependant layer for self-supervised nuclei encoding
arxiv(2022)
摘要
Recent developments in self-supervised learning give us the possibility to further reduce human intervention in multi-step pipelines where the focus evolves around particular objects of interest. In the present paper, the focus lays in the nuclei in histopathology images. In particular we aim at extracting cellular information in an unsupervised manner for a downstream task. As nuclei present themselves in a variety of sizes, we propose a new Scale-dependant convolutional layer to bypass scaling issues when resizing nuclei. On three nuclei datasets, we benchmark the following methods: handcrafted, pre-trained ResNet, supervised ResNet and self-supervised features. We show that the proposed convolution layer boosts performance and that this layer combined with Barlows-Twins allows for better nuclei encoding compared to the supervised paradigm in the low sample setting and outperforms all other proposed unsupervised methods. In addition, we extend the existing TNBC dataset to incorporate nuclei class annotation in order to enrich and publicly release a small sample setting dataset for nuclei segmentation and classification.
更多查看译文
关键词
nuclei,dependant layer,encoding,scale,self-supervised
AI 理解论文
溯源树
样例
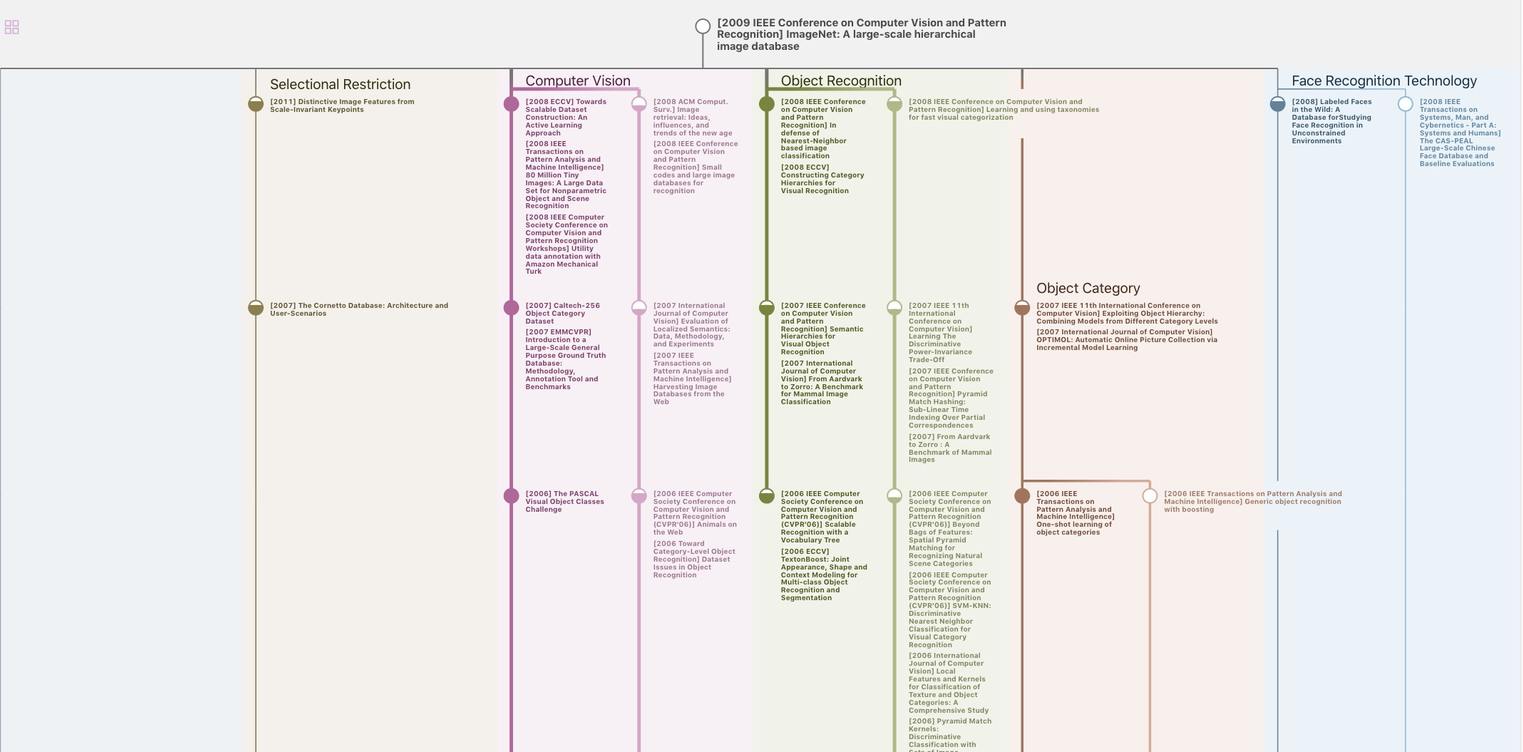
生成溯源树,研究论文发展脉络
Chat Paper
正在生成论文摘要