A Convolutional Attention Based Deep Learning Solution for 5G UAV Network Attack Recognition over Fading Channels and Interference.
arxiv(2022)
摘要
When users exchange data with Unmanned Aerial Vehicles - (UAVs) over Air-to-Ground - (A2G) wireless communication networks, they expose the link to attacks that could increase packet loss and might disrupt connectivity. For example, in emergency deliveries, losing control information (i.e., data related to the UAV control communication) might result in accidents that cause UAV destruction and damage to buildings or other elements. To prevent these problems, these issues must be addressed in 5G and 6G scenarios. This research offers a Deep Learning (DL) approach for detecting attacks on UAVs equipped with Orthogonal Frequency Division Multiplexing - (OFDM) receivers on Clustered Delay Line (CDL) channels in highly complex scenarios involving authenticated terrestrial users, as well as attackers in unknown locations. We use the two observable parameters available in 5G UAV connections: the Received Signal Strength Indicator (RSSI) and the Signal to Interference plus Noise Ratio (SINR). The developed algorithm is generalizable regarding attack identification, which does not occur during training. Further, it can identify all the attackers in the environment with 20 terrestrial users. A deeper investigation into the timing requirements for recognizing attacks shows that after training, the minimum time necessary after the attack begins is 100 ms, and the minimum attack power is 2 dBm, which is the same power that the authenticated UAV uses. The developed algorithm also detects moving attackers from a distance of 500 m.
更多查看译文
关键词
Cybersecurity, Convolutional Neural Networks, Deep Learning, Jamming Detection, Jamming Identification, Unmanned Aerial Vehicles, 5G
AI 理解论文
溯源树
样例
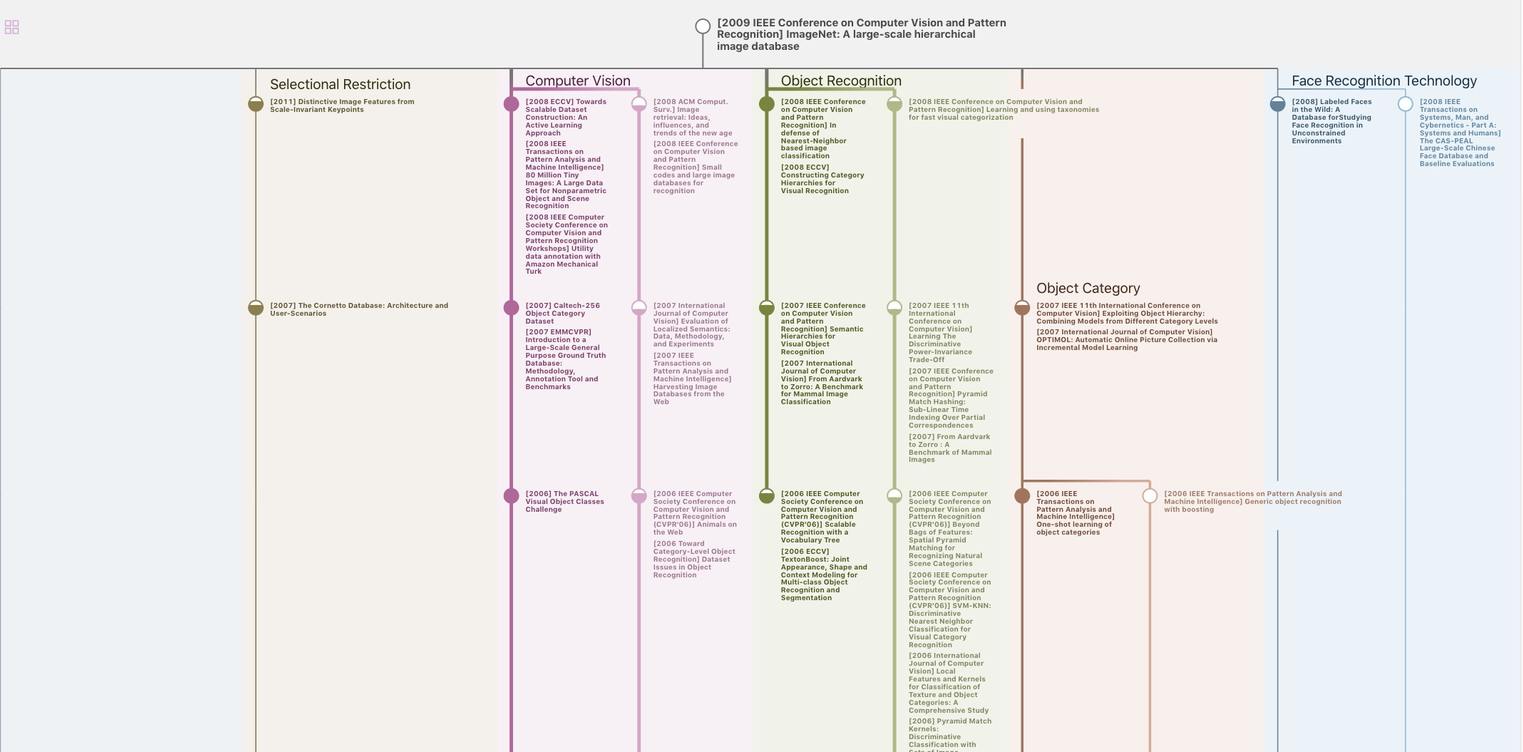
生成溯源树,研究论文发展脉络
Chat Paper
正在生成论文摘要