Optimizing the dynamic treatment regime of in-hospital warfarin anticoagulation in patients after surgical valve replacement using reinforcement learning
JOURNAL OF THE AMERICAN MEDICAL INFORMATICS ASSOCIATION(2022)
摘要
Objective Warfarin anticoagulation management requires sequential decision-making to adjust dosages based on patients' evolving states continuously. We aimed to leverage reinforcement learning (RL) to optimize the dynamic in-hospital warfarin dosing in patients after surgical valve replacement (SVR). Materials and Methods 10 408 SVR cases with warfarin dosage-response data were retrospectively collected to develop and test an RL algorithm that can continuously recommend daily warfarin doses based on patients' evolving multidimensional states. The RL algorithm was compared with clinicians' actual practice and other machine learning and clinical decision rule-based algorithms. The primary outcome was the ratio of patients without in-hospital INRs >3.0 and the INR at discharge within the target range (1.8-2.5) (excellent responders). The secondary outcomes were the safety responder ratio (no INRs >3.0) and the target responder ratio (the discharge INR within 1.8-2.5). Results In the test set (n = 1260), the excellent responder ratio under clinicians' guidance was significantly lower than the RL algorithm: 41.6% versus 80.8% (relative risk [RR], 0.51; 95% confidence interval [CI], 0.48-0.55), also the safety responder ratio: 83.1% versus 99.5% (RR, 0.83; 95% CI, 0.81-0.86), and the target responder ratio: 49.7% versus 81.1% (RR, 0.61; 95% CI, 0.58-0.65). The RL algorithms performed significantly better than all the other algorithms. Compared with clinicians' actual practice, the RL-optimized INR trajectory reached and maintained within the target range significantly faster and longer. Discussion RL could offer interactive, practical clinical decision support for sequential decision-making tasks and is potentially adaptable for varied clinical scenarios. Prospective validation is needed. Conclusion An RL algorithm significantly optimized the post-operation warfarin anticoagulation quality compared with clinicians' actual practice, suggesting its potential for challenging sequential decision-making tasks.
更多查看译文
关键词
anticoagulation, clinical decision-making, dynamic treatment regime, reinforcement learning, warfarin
AI 理解论文
溯源树
样例
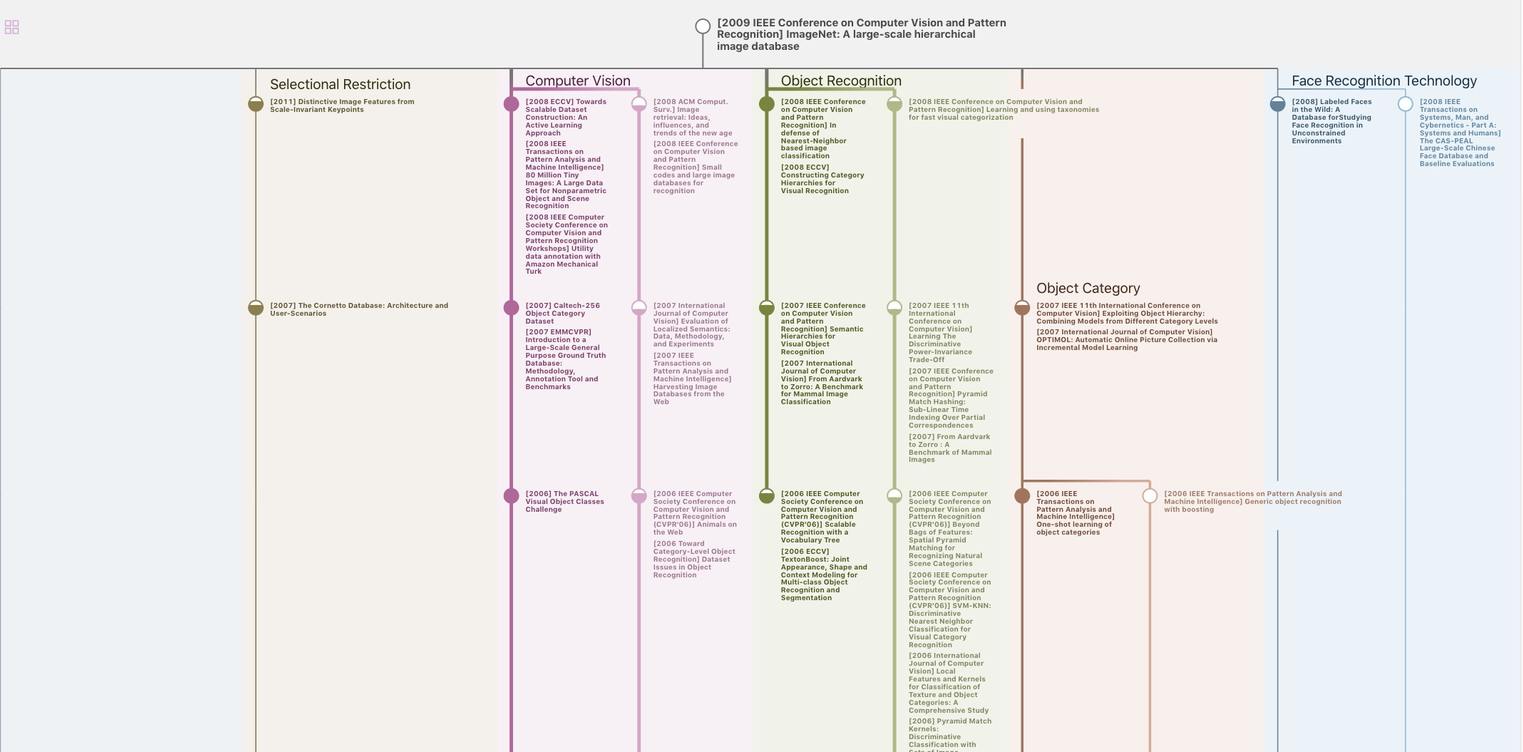
生成溯源树,研究论文发展脉络
Chat Paper
正在生成论文摘要