Multi-task deep learning for glaucoma detection from color fundus images
SCIENTIFIC REPORTS(2022)
摘要
Glaucoma is an eye condition that leads to loss of vision and blindness if not diagnosed in time. Diagnosis requires human experts to estimate in a limited time subtle changes in the shape of the optic disc from retinal fundus images. Deep learning methods have been satisfactory in classifying and segmenting diseases in retinal fundus images, assisting in analyzing the increasing amount of images. Model training requires extensive annotations to achieve successful generalization, which can be highly problematic given the costly expert annotations. This work aims at designing and training a novel multi-task deep learning model that leverages the similarities of related eye-fundus tasks and measurements used in glaucoma diagnosis. The model simultaneously learns different segmentation and classification tasks, thus benefiting from their similarity. The evaluation of the method in a retinal fundus glaucoma challenge dataset, including 1200 retinal fundus images from different cameras and medical centers, obtained a 96.76 ± 0.96 AUC performance compared to an 93.56 ± 1.48 obtained by the same backbone network trained to detect glaucoma. Our approach outperforms other multi-task learning models, and its performance pairs with trained experts using ∼ 3.5 times fewer parameters than training each task separately. The data and the code for reproducing our results are publicly available.
更多查看译文
关键词
Biomedical engineering,Computer science,Optic nerve diseases,Science,Humanities and Social Sciences,multidisciplinary
AI 理解论文
溯源树
样例
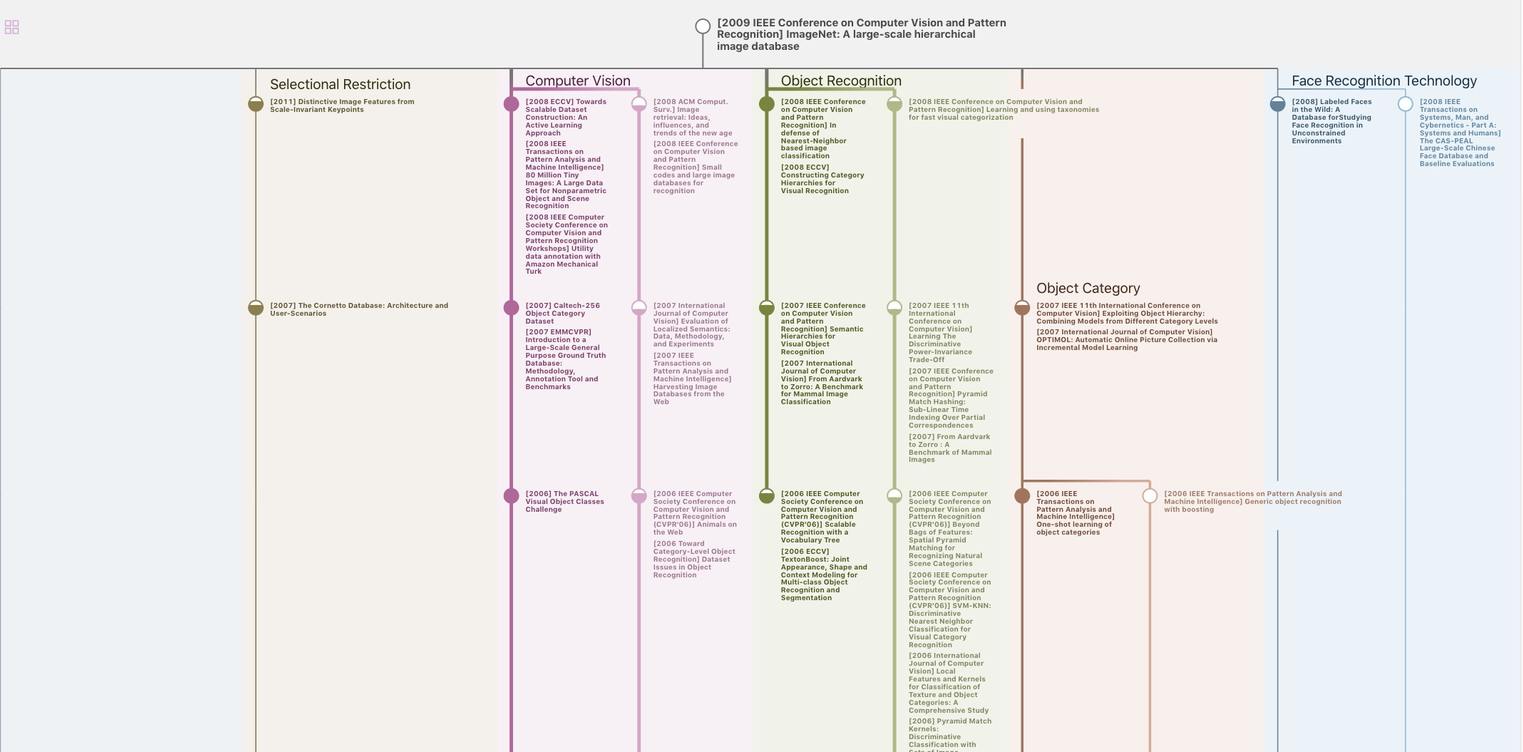
生成溯源树,研究论文发展脉络
Chat Paper
正在生成论文摘要