Context-Patch Representation Learning With Adaptive Neighbor Embedding for Robust Face Image Super-Resolution
IEEE Transactions on Multimedia(2023)
摘要
Representation learning steered robust face image super-resolution (FSR) methods have attracted extensive attention in the past few decades. Most previous methods were devoted to exploiting the local position patches in the training set for FSR. However, they usually overlooked the sufficient usage of the contextual information around the testing patches, which are useful for stable representation learning. In this article, we attempt to utilize the context-patch around the testing patch and propose a method named context-patch representation learning with adaptive neighbor embedding (CRL-ANE) for FSR. On one hand, we simultaneously use the testing position patch and its adjacent ones for stable representation weight learning. This contextual information can compensate for recovering missing details in the target patch. On the other hand, for each input patch set, due to its inherent facial structural properties, we design an adaptive neighbor embedding strategy to elaborately and adaptively choose primary candidates for more accurate reconstruction. These two improvements enable the proposed method to achieve better SR performance than some of the other methods. Qualitative and quantitative experiments on some benchmarks have validated the superiority of the proposed method over some state-of-the-art methods.
更多查看译文
关键词
Training, Faces, Image reconstruction, Face recognition, Representation learning, Adaptation models, Testing, Adaptive neighbor embedding, contextual information, face super-resolution, representation learning
AI 理解论文
溯源树
样例
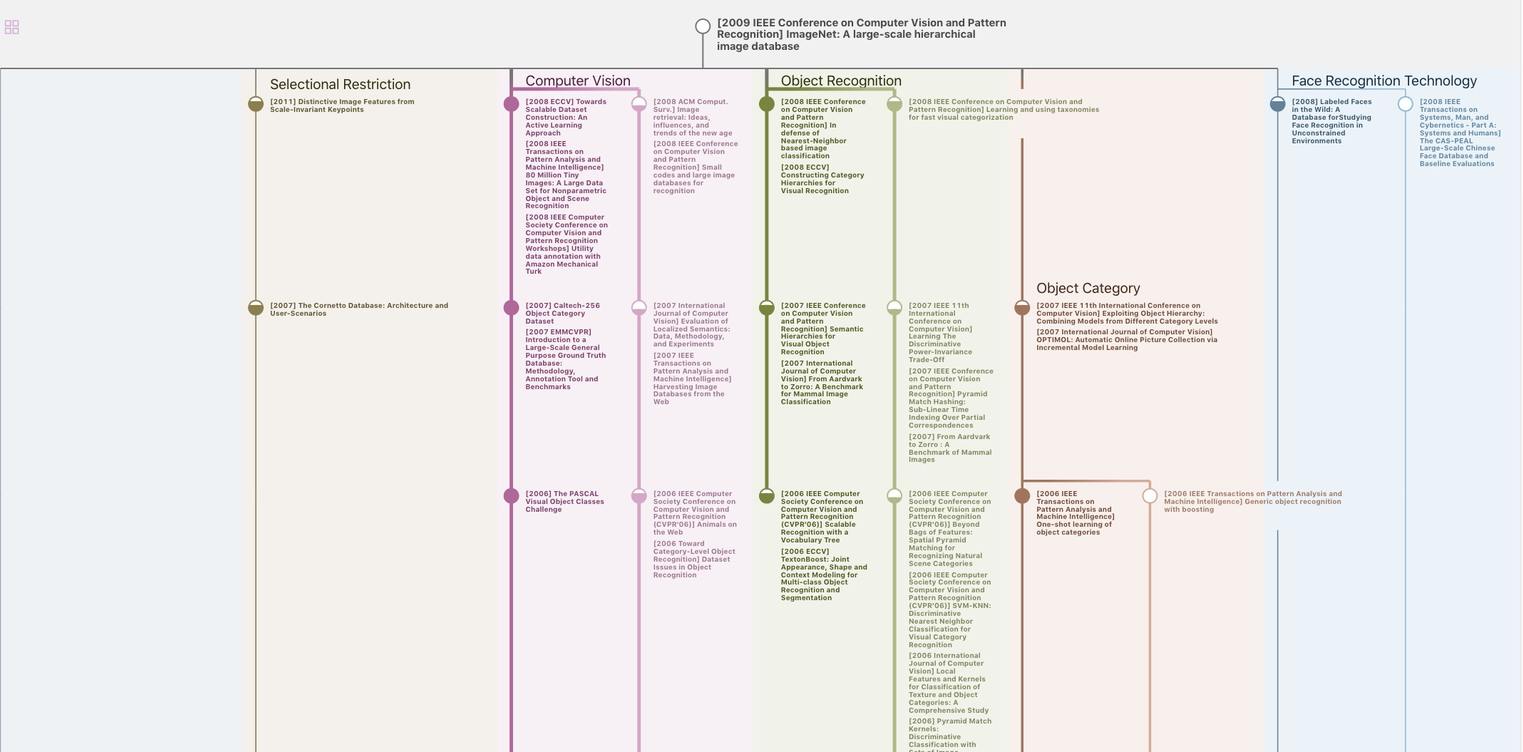
生成溯源树,研究论文发展脉络
Chat Paper
正在生成论文摘要