Mitigating Algorithmic Bias with Limited Annotations
MACHINE LEARNING AND KNOWLEDGE DISCOVERY IN DATABASES: RESEARCH TRACK, ECML PKDD 2023, PT II(2023)
摘要
Existing work on fairness modeling commonly assumes that sensitive attributes for all instances are fully available, which may not be true in many real-world applications due to the high cost of acquiring sensitive information. When sensitive attributes are not disclosed or available, it is needed to manually annotate a small part of the training data to mitigate bias. However, the skewed distribution across different sensitive groups preserves the skewness of the original dataset in the annotated subset, which leads to non-optimal bias mitigation. To tackle this challenge, we propose Active Penalization Of Discrimination (APOD), an interactive framework to guide the limited annotations towards maximally eliminating the effect of algorithmic bias. The proposed APOD integrates discrimination penalization with active instance selection to efficiently utilize the limited annotation budget, and it is theoretically proved to be capable of bounding the algorithmic bias. According to the evaluation on five benchmark datasets, APOD outperforms the state-of-the-arts baseline methods under the limited annotation budget, and shows comparable performance to fully annotated bias mitigation, which demonstrates that APOD could benefit real-world applications when sensitive information is limited. The source code of the proposed method is available at: https://github.com/guanchuwang/APOD-fairness.
更多查看译文
关键词
Bias mitigation,Limitied annotation
AI 理解论文
溯源树
样例
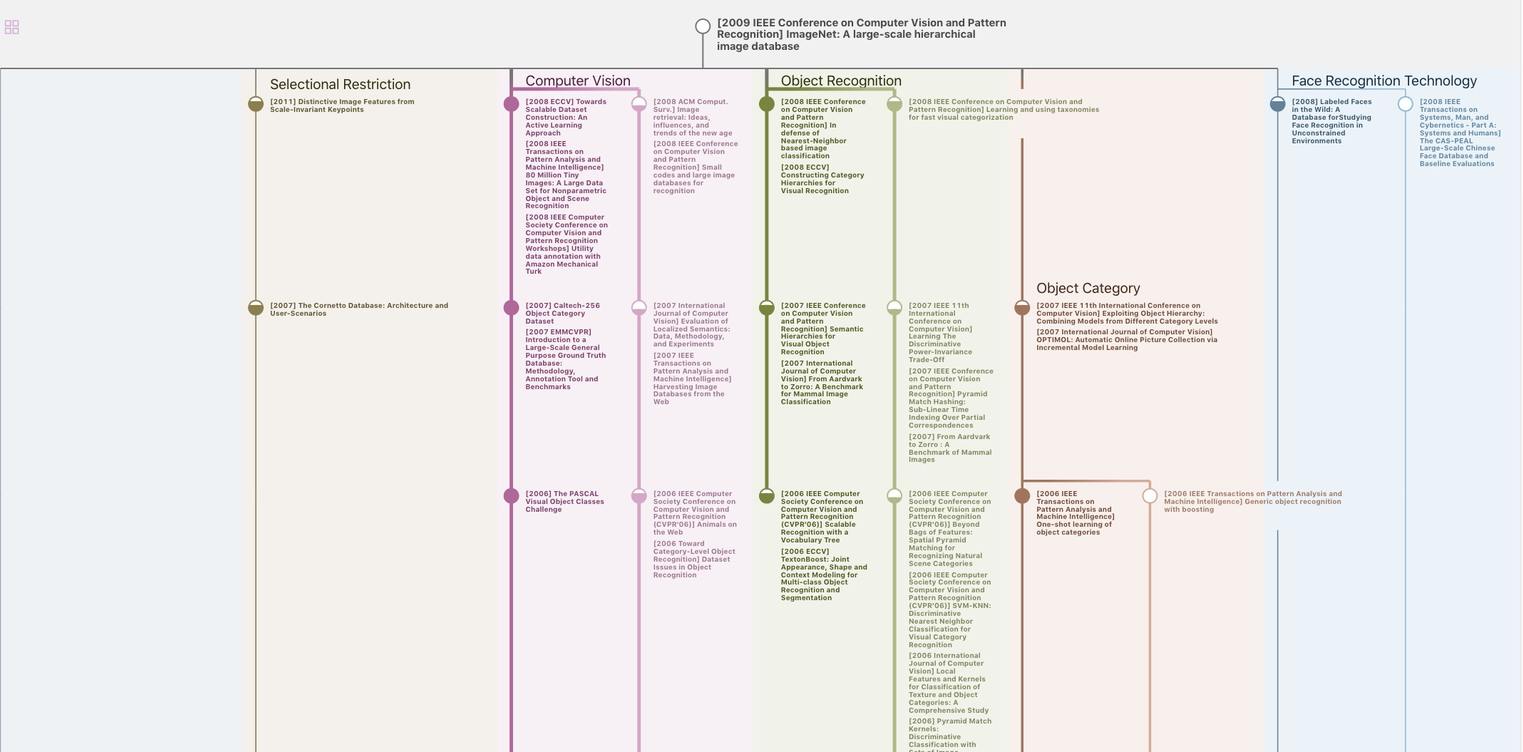
生成溯源树,研究论文发展脉络
Chat Paper
正在生成论文摘要