Evaluating the Stability of Deep Image Quality Assessment With Respect to Image Scaling
arxiv(2022)
摘要
Image quality assessment (IQA) is a fundamental metric for image processing tasks (e.g., compression). With full-reference IQAs, traditional IQAs, such as PSNR and SSIM, have been used. Recently, IQAs based on deep neural networks (deep IQAs), such as LPIPS and DISTS, have also been used. It is known that image scaling is inconsistent among deep IQAs, as some perform down-scaling as pre-processing, whereas others instead use the original image size. In this paper, we show that the image scale is an influential factor that affects deep IQA performance. We comprehensively evaluate four deep IQAs on the same five datasets, and the experimental results show that image scale significantly influences IQA performance. We found that the most appropriate image scale is often neither the default nor the original size, and the choice differs depending on the methods and datasets used. We visualized the stability and found that PieAPP is the most stable among the four deep IQAs.
更多查看译文
关键词
 ,full-reference image quality assessment,image scale,neural networks
AI 理解论文
溯源树
样例
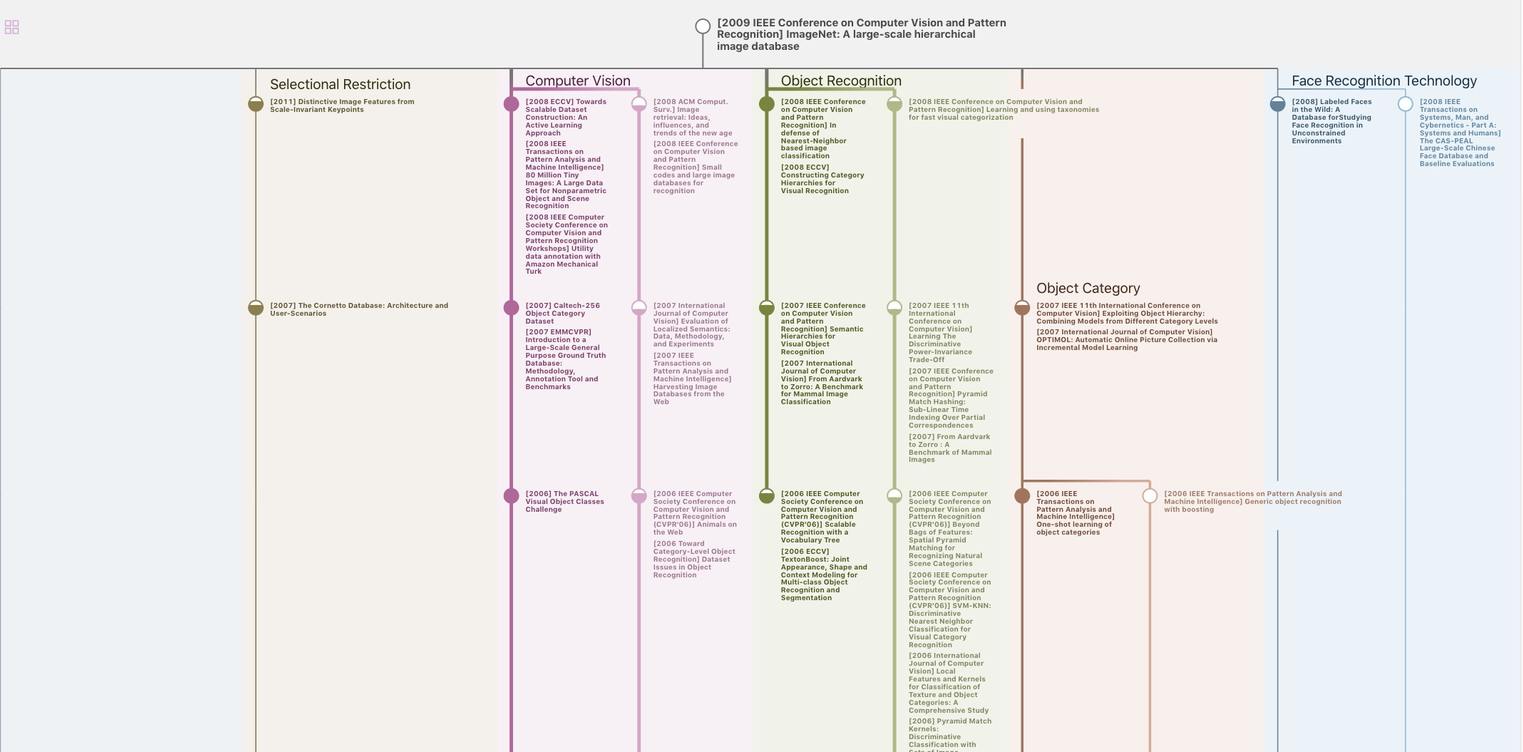
生成溯源树,研究论文发展脉络
Chat Paper
正在生成论文摘要