Multigraph Topology Design for Cross-Silo Federated Learning
arxiv(2022)
摘要
Cross-silo federated learning utilizes a few hundred reliable data silos with high-speed access links to jointly train a model. While this approach becomes a popular setting in federated learning, designing a robust topology to reduce the training time is still an open problem. In this paper, we present a new multigraph topology for cross-silo federated learning. We first construct the multigraph using the overlay graph. We then parse this multigraph into different simple graphs with isolated nodes. The existence of isolated nodes allows us to perform model aggregation without waiting for other nodes, hence reducing the training time. We further propose a new distributed learning algorithm to use with our multigraph topology. The intensive experiments on public datasets show that our proposed method significantly reduces the training time compared with recent state-of-the-art topologies while ensuring convergence and maintaining the model's accuracy.
更多查看译文
关键词
Federated Learning,Topology Design,Multigraph
AI 理解论文
溯源树
样例
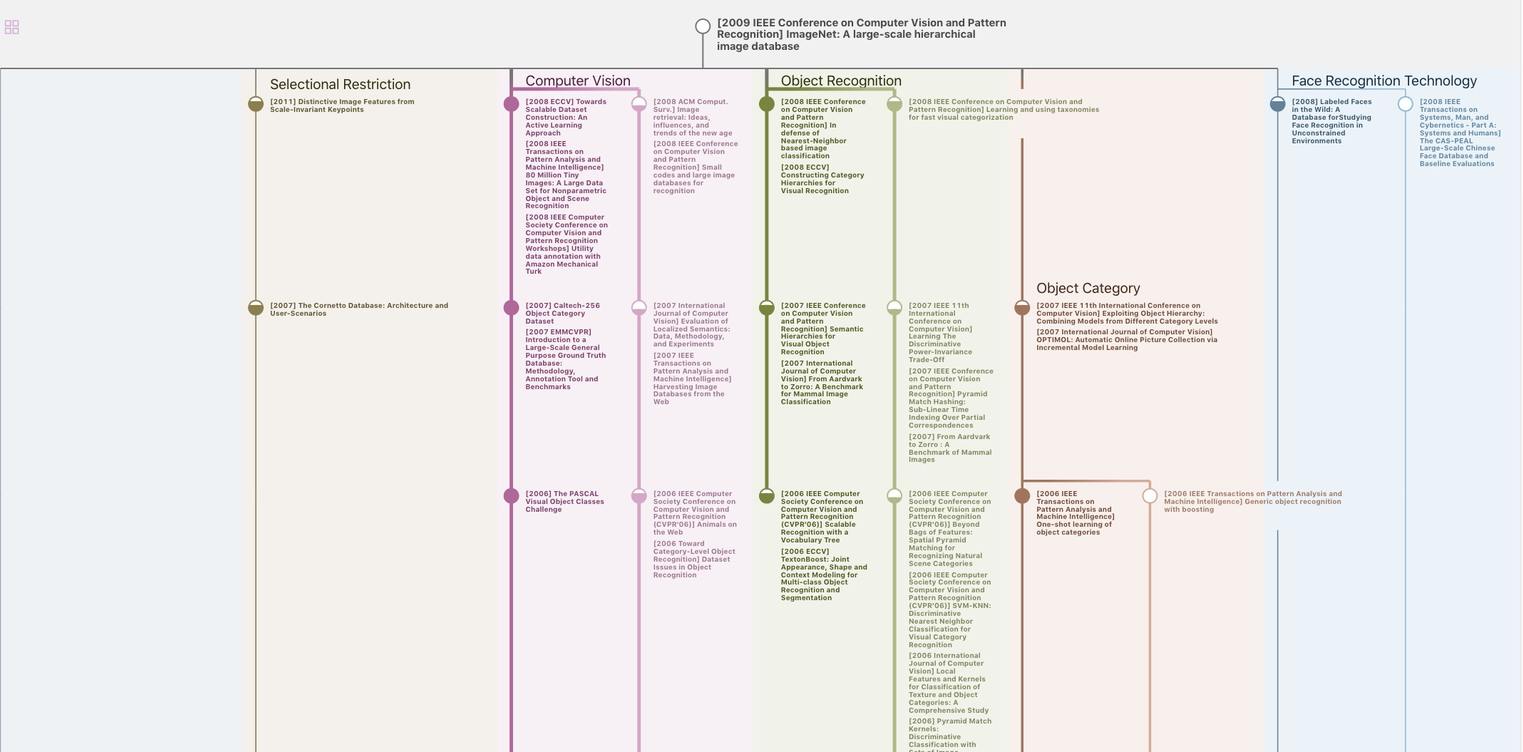
生成溯源树,研究论文发展脉络
Chat Paper
正在生成论文摘要